Convolutional Generative Adversarial Network, via Transfer Learning, for Traditional Scottish Music Generation.
EvoMUSART(2021)
摘要
The concept of a Binary Multi-track Sequential Generative Adversarial Network (BinaryMuseGAN) used for the generation of music has been applied and tested for various types of music. However, the concept is yet to be tested on more specific genres of music such as traditional Scottish music, for which extensive collections are not readily available. Hence exploring the capabilities of a Transfer Learning (TL) approach on these types of music is an interesting challenge for the methodology. The curated set of MIDI Scottish melodies was preprocessed in order to obtain the same number of tracks used in the BinaryMuseGAN model; converted into pianoroll format and then used as a training set to fine tune a pretrained model, generated from the Lakh MIDI dataset. The results obtained have been compared with the results obtained by training the same GAN model from scratch on the sole Scottish music dataset. Results are presented in terms of variation and average performances achieved at different epochs for five performance metrics, three adopted from the Lakh dataset (qualified note rate, polyphonicity, tonal distance) and two custom defined to highlight Scottish music characteristics (dotted rhythm and pentatonic note). From these results, the TL method shows to be more effective, with lower number of epochs, to converge stably and closely to the original dataset reference metrics values.
更多查看译文
关键词
Generative Adversarial Network, Transfer Learning, Convolutional Neural Network, Scottish music
AI 理解论文
溯源树
样例
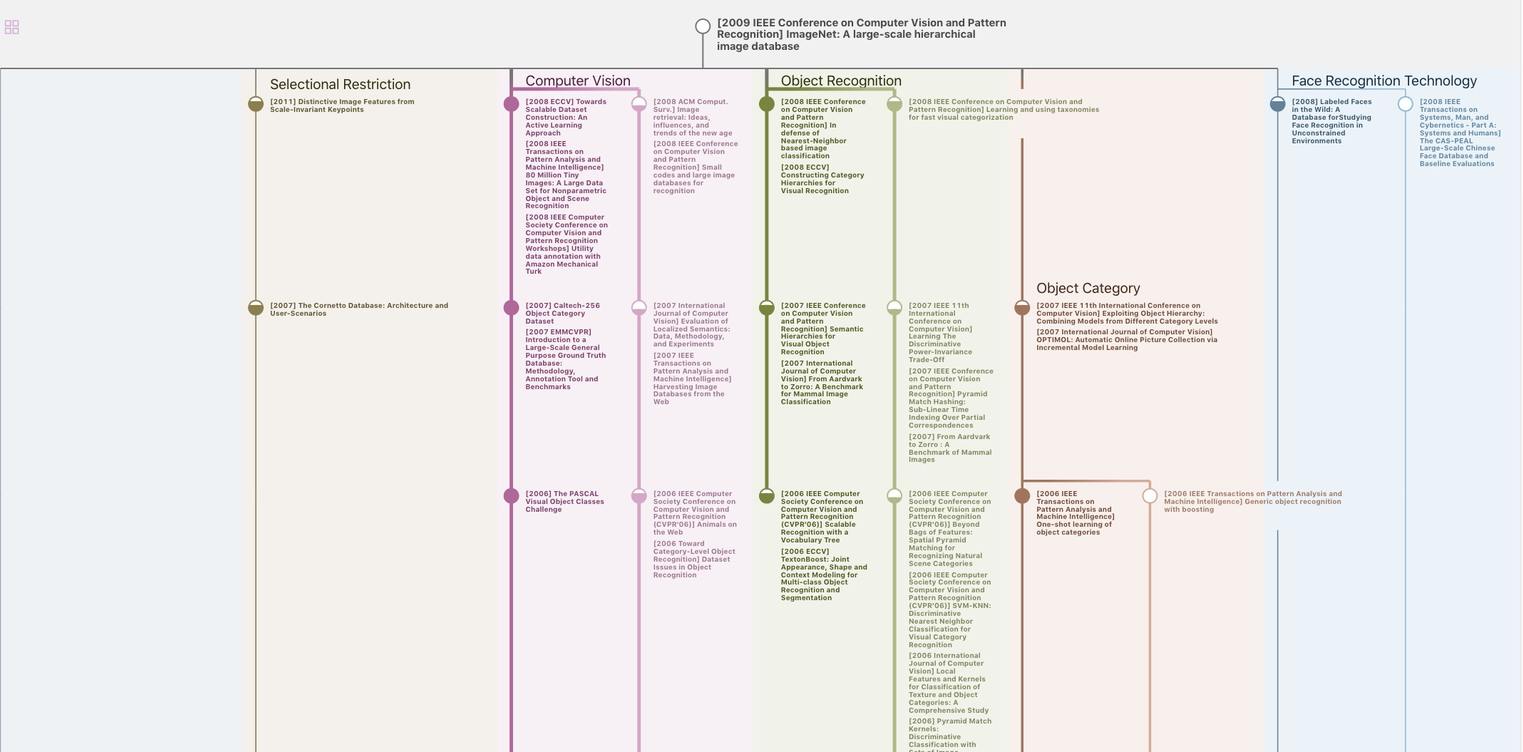
生成溯源树,研究论文发展脉络
Chat Paper
正在生成论文摘要