Alzheimer’s Brain Network Analysis Using Sparse Learning Feature Selection
S+SSPR(2020)
摘要
Accurate identification of Mild Cognitive Impairment (MCI) based on resting-state functional Magnetic Resonance Imaging (RS-fMRI) is crucial for reducing the risk of developing Alzheimer’s disease (AD). In the literature, functional connectivity (FC) is often used to extract brain network features. However, it still remains challenging for the estimation of FC because RS-fMRI data are often high-dimensional and small in sample size. Although various Lasso-type sparse learning feature selection methods have been adopted to identify the most discriminative features for brain disease diagnosis, they suffer from two common drawbacks. First, Lasso is instable and not very satisfactory for the high-dimensional and small sample size problem. Second, existing Lasso-type feature selection methods have not simultaneously encapsulate the joint correlations between pairwise features and the target, the correlations between pairwise features, and the joint feature interaction into the feature selection process, thus may lead to suboptimal solutions. To overcome these issues, we propose a novel sparse learning feature selection method for MCI classification in this work. It unifies the above measures into a minimization problem associated with a least square error and an Elastic Net regularizer. Experimental results demonstrate that the diagnosis accuracy for MCI subjects can be significantly improved using our proposed feature selection method.
更多查看译文
关键词
Alzheimer’s disease,Feature selection,Elastic net
AI 理解论文
溯源树
样例
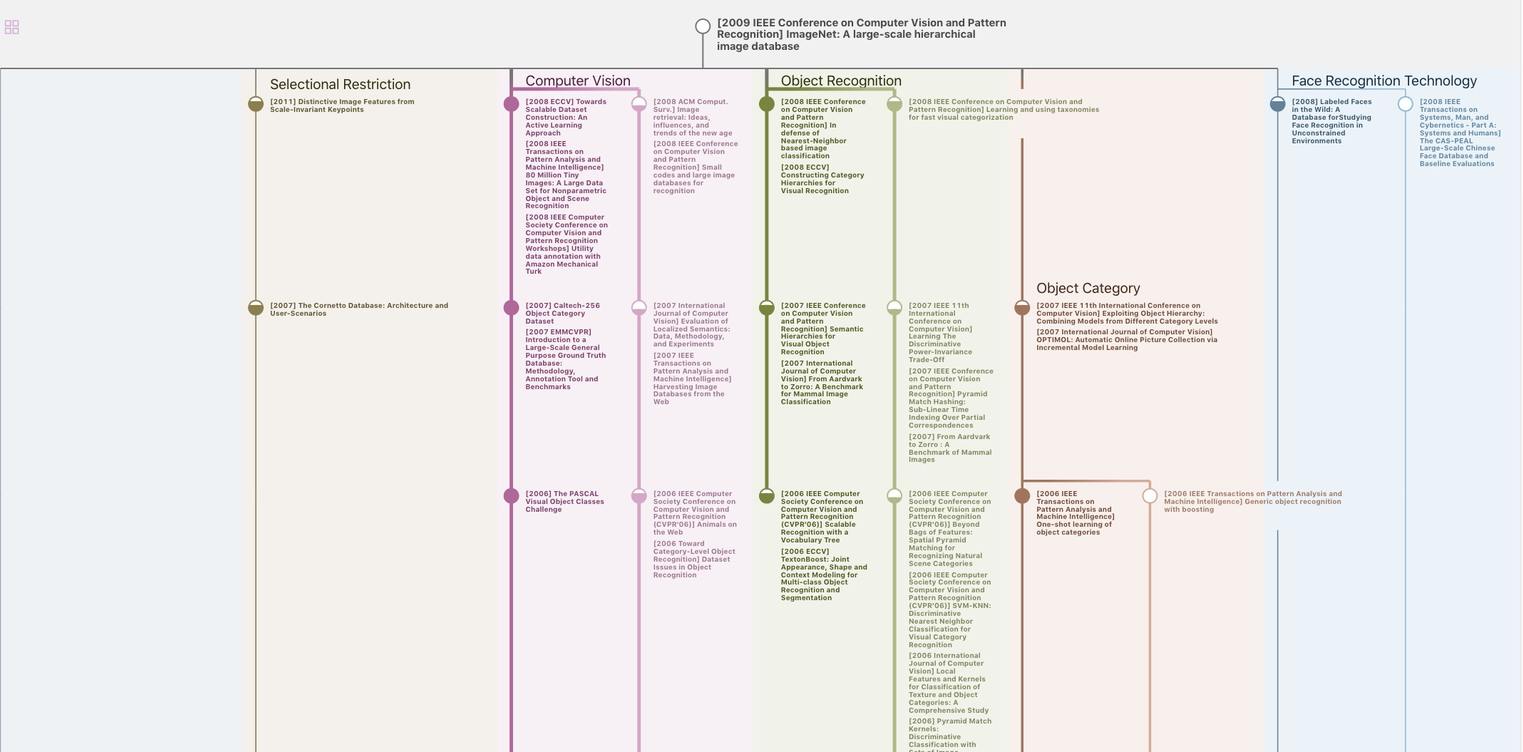
生成溯源树,研究论文发展脉络
Chat Paper
正在生成论文摘要