Evaluating Model Robustness And Stability To Dataset Shift
24TH INTERNATIONAL CONFERENCE ON ARTIFICIAL INTELLIGENCE AND STATISTICS (AISTATS)(2021)
摘要
As the use of machine learning in high impact domains becomes widespread, the importance of evaluating safety has increased. An important aspect of this is evaluating how robust a model is to changes in setting or population, which typically requires applying the model to multiple, independent datasets. Since the cost of collecting such datasets is often prohibitive, in this paper, we propose a framework for analyzing this type of stability using the available data. We use the original evaluation data to determine distributions under which the algorithm performs poorly, and estimate the algorithm's performance on the "worst-case" distribution. We consider shifts in user defined conditional distributions, allowing some distributions to shift while keeping other portions of the data distribution fixed. For example, in a healthcare context, this allows us to consider shifts in clinical practice while keeping the patient population fixed. To address the challenges associated with estimation in complex, high-dimensional distributions, we derive a "debiased" estimator which maintains root N-consistency even when machine learning methods with slower convergence rates are used to estimate the nuisance parameters. In experiments on a real medical risk prediction task, we show this estimator can be used to analyze stability and accounts for realistic shifts that could not previously be expressed. The proposed framework allows practitioners to proactively evaluate the safety of their models without requiring additional data collection.
更多查看译文
关键词
model robustness,stability
AI 理解论文
溯源树
样例
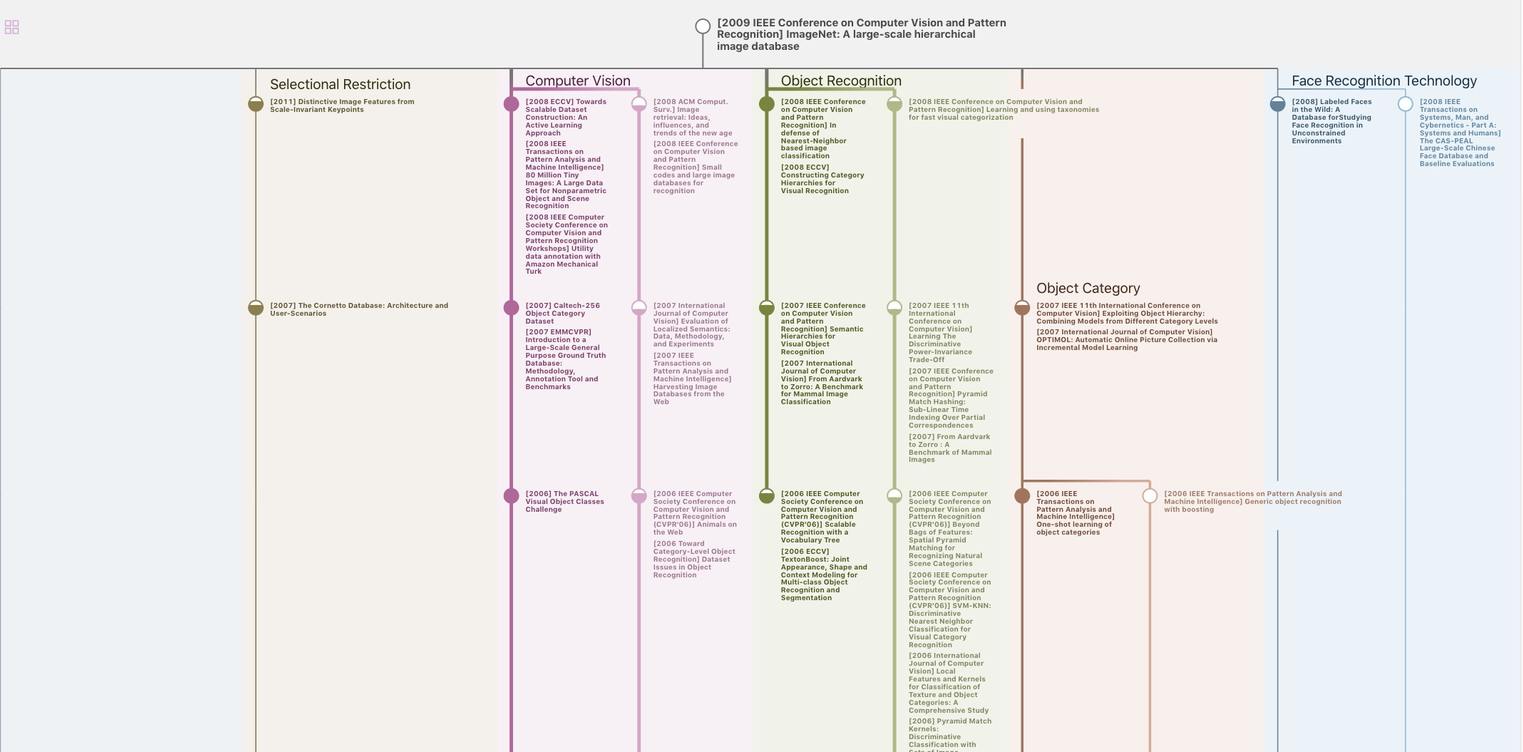
生成溯源树,研究论文发展脉络
Chat Paper
正在生成论文摘要