Density of States Estimation for Out of Distribution Detection.
AISTATS(2021)
摘要
Perhaps surprisingly, recent studies have shown probabilistic model likelihoods have poor specificity for out-of-distribution (OOD) detection and often assign higher likelihoods to OOD data than in-distribution data. To ameliorate this issue we propose DoSE, the density of states estimator. Drawing on the statistical physics notion of ``density of states,\u0027\u0027 the DoSE decision rule avoids direct comparison of model probabilities, and instead utilizes the ``probability of the model probability,\u0027\u0027 or indeed the frequency of any reasonable statistic. The frequency is calculated using nonparametric density estimators (e.g., KDE and one-class SVM) which measure the typicality of various model statistics given the training data and from which we can flag test points with low typicality as anomalous. Unlike many other methods, DoSE requires neither labeled data nor OOD examples. DoSE is modular and can be trivially applied to any existing, trained model. We demonstrate DoSE\u0027s state-of-the-art performance against other unsupervised OOD detectors on previously established ``hard\u0027\u0027 benchmarks.
更多查看译文
关键词
states estimation,detection,out-of-distribution
AI 理解论文
溯源树
样例
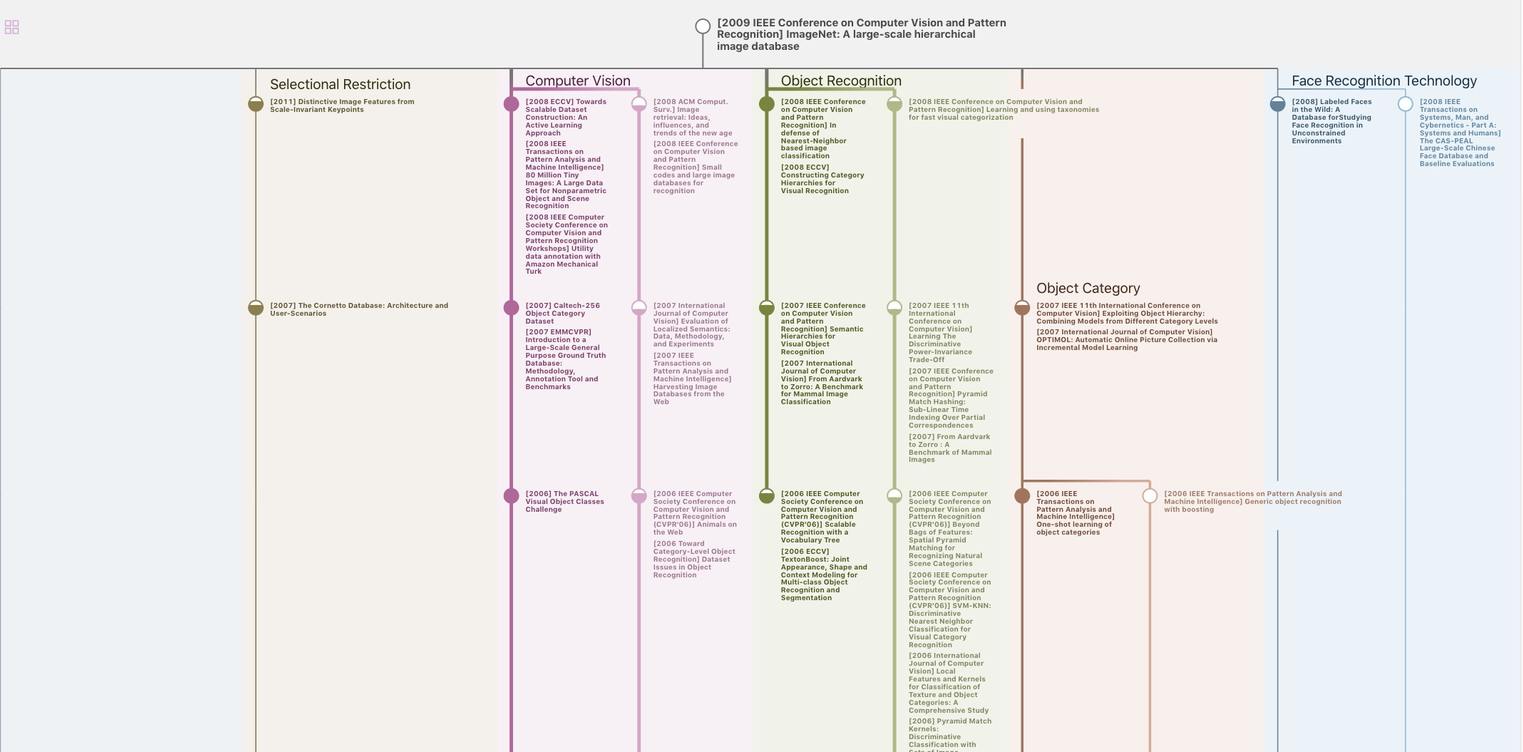
生成溯源树,研究论文发展脉络
Chat Paper
正在生成论文摘要