Communication Efficient Primal-Dual Algorithm For Nonconvex Nonsmooth Distributed Optimization
24TH INTERNATIONAL CONFERENCE ON ARTIFICIAL INTELLIGENCE AND STATISTICS (AISTATS)(2021)
摘要
Decentralized optimization frequently appears in large scale machine learning problems. However, few works have been focused on solving the decentralized optimization problems under the difficult nonconvex nonsmooth setting. In this paper, we propose a distributed primal-dual algorithm to solve this type of problems in a decentralized manner and the proposed algorithm can achieve an O (1/epsilon(2)) iteration complexity to attain an epsilon-solution, which is the well-known lower iteration complexity bound for nonconvex optimization. Furthermore, to reduce communication overhead, we also modify our algorithm by compressing the vectors exchanged between nodes. The iteration complexity of the algorithm with compression is still O(1/epsilon(2)). To our knowledge, it is the first algorithm achieving this rate under a nonconvex, nonsmooth decentralized setting with compression. Besides, we apply the proposed algorithm to solve nonconvex linear regression problem and train a deep learning model, both of which demonstrate the efficacy of the proposed algorithms.
更多查看译文
AI 理解论文
溯源树
样例
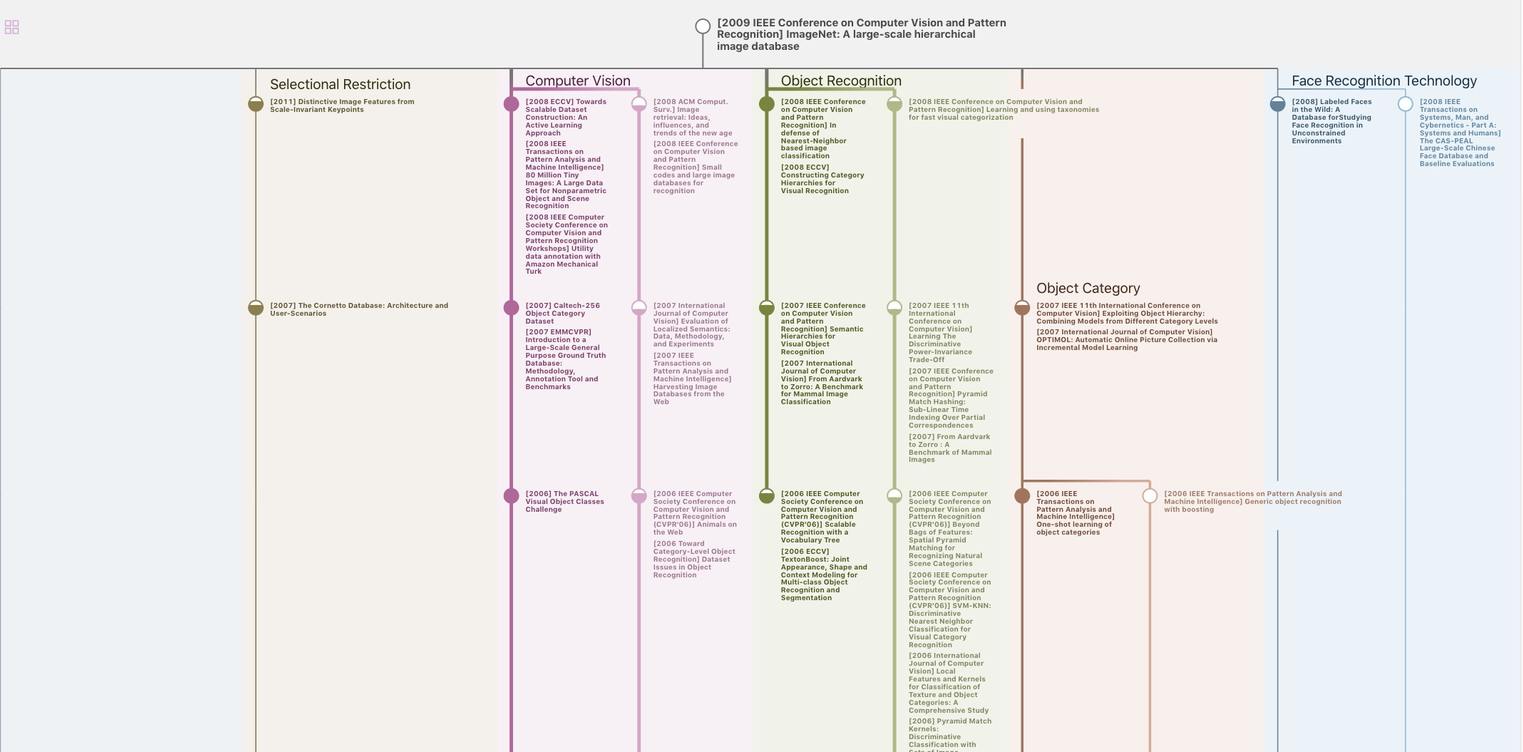
生成溯源树,研究论文发展脉络
Chat Paper
正在生成论文摘要