Composite Surrogate for Likelihood-Free Bayesian Optimisation in High-Dimensional Settings of Activity-Based Transportation Models
ADVANCES IN INTELLIGENT DATA ANALYSIS XIX, IDA 2021(2021)
摘要
Activity-based transportation models simulate demand and supply as a complex system and therefore large set of parameters need to be adjusted. One such model is Preday activity-based model that requires adjusting a large set of parameters for its calibration on new urban environments. Hence, the calibration process is time demanding, and due to costly simulations, various optimisation methods with dimensionality reduction and stochastic approximation are adopted. This study adopts Bayesian Optimisation for Likelihood-free Inference (BOLFI) method for calibrating the Preday activity-based model to a new urban area. Unlike the traditional variant of the method that uses Gaussian Process as a surrogate model for approximating the likelihood function through modelling discrepancy, we apply a composite surrogate model that encompasses Random Forest surrogate model for modelling the discrepancy and Gaussian Mixture Model for estimating the its density. The results show that the proposed method benefits the extension and improves the general applicability to high-dimensional settings without losing the efficiency of the Bayesian Optimisation in sampling new samples towards the global optima.
更多查看译文
关键词
Transportation model, High-dimensional data, Bayesian optimisation, Likelihood-free inference, Random forest
AI 理解论文
溯源树
样例
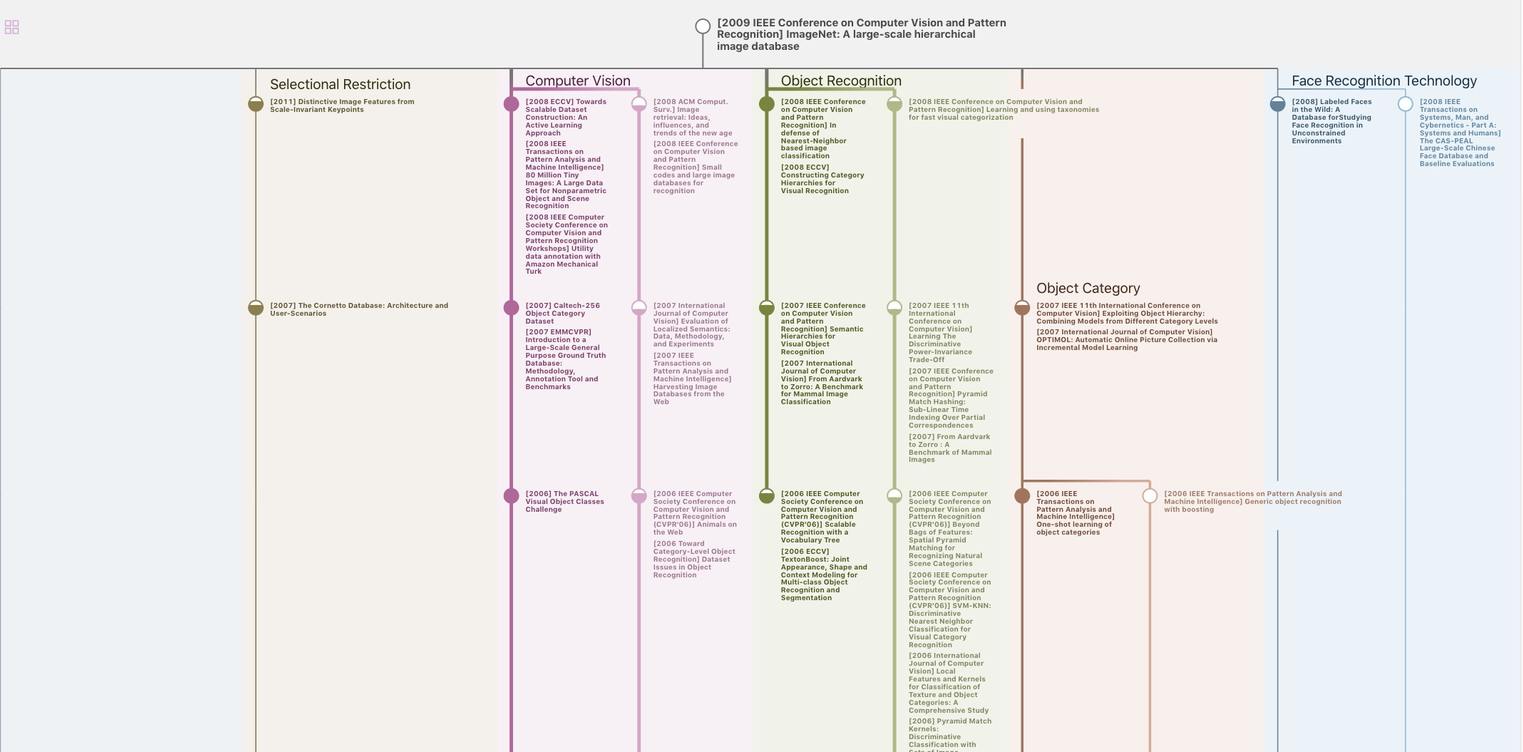
生成溯源树,研究论文发展脉络
Chat Paper
正在生成论文摘要