Facial Representation Extraction by Mutual Information Maximization and Correlation Minimization.
ACIIDS(2021)
摘要
Facial expression recognition and face recognition are two amusing and practical research orientations in computer vision. Multi-task joint learning can improve each other's performance, which has rarely been studied in the past. This work proposes a joint learning framework to enhance emotional representation and identity representation extraction by incorporating a multi-loss training strategy. Specifically, we propose mutual information loss to ensure that the facial representation is unique and complete and offer correlation loss to extract identity representation using orthogonality constraints. Classification loss is used to learn emotional representation. As a result, we can obtain an unsupervised learning framework to reduce the identity annotation bottleneck using large-scale labelled emotional data for the face verification task. Our algorithm is verified on an artificially synthesized face database: Large-scale Synthesized Facial Expression Dataset (LSFED) and its variants. The identity representation obtained by the algorithm is used for face verification. The performance is comparable to some existing supervised face verification methods.
更多查看译文
关键词
Facial representation extraction, Mutual information, Correlation constraint
AI 理解论文
溯源树
样例
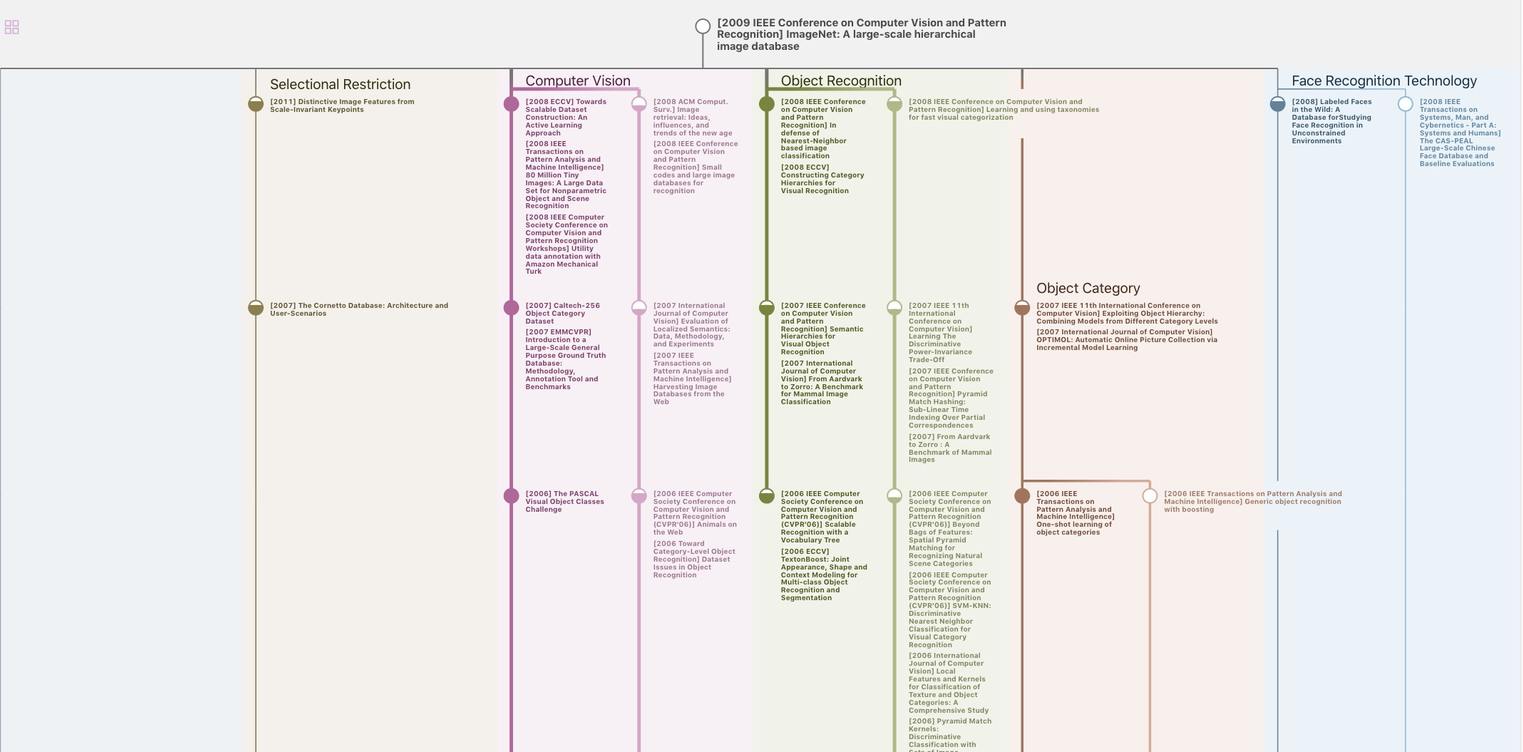
生成溯源树,研究论文发展脉络
Chat Paper
正在生成论文摘要