Robust generalised Bayesian inference for intractable likelihoods
JOURNAL OF THE ROYAL STATISTICAL SOCIETY SERIES B-STATISTICAL METHODOLOGY(2022)
摘要
Generalised Bayesian inference updates prior beliefs using a loss function, rather than a likelihood, and can therefore be used to confer robustness against possible mis-specification of the likelihood. Here we consider generalised Bayesian inference with a Stein discrepancy as a loss function, motivated by applications in which the likelihood contains an intractable normalisation constant. In this context, the Stein discrepancy circumvents evaluation of the normalisation constant and produces generalised posteriors that are either closed form or accessible using the standard Markov chain Monte Carlo. On a theoretical level, we show consistency, asymptotic normality, and bias-robustness of the generalised posterior, highlighting how these properties are impacted by the choice of Stein discrepancy. Then, we provide numerical experiments on a range of intractable distributions, including applications to kernel-based exponential family models and non-Gaussian graphical models.
更多查看译文
关键词
intractable likelihood, kernel methods, robust statistics, Stein's method
AI 理解论文
溯源树
样例
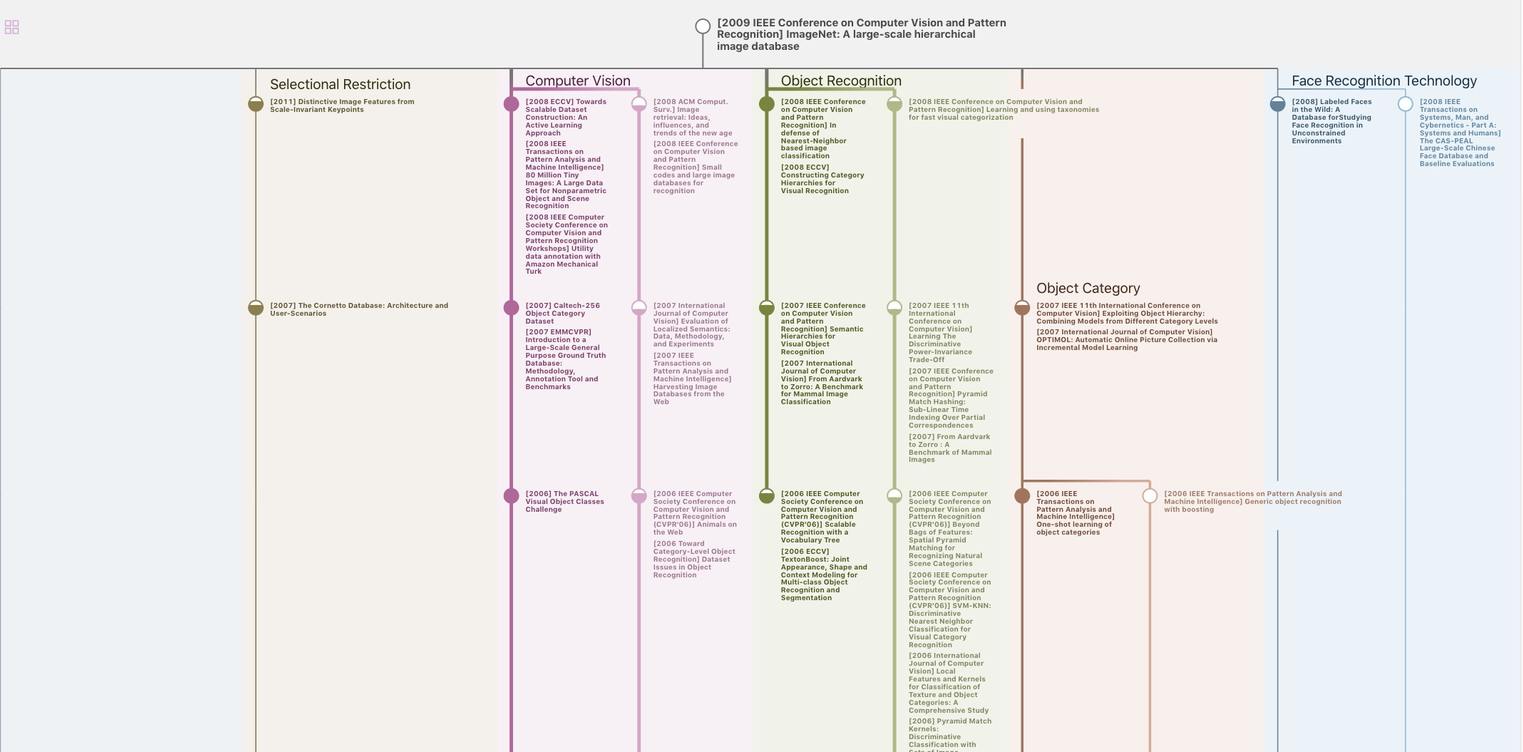
生成溯源树,研究论文发展脉络
Chat Paper
正在生成论文摘要