Zeus: Efficiently Localizing Actions in Videos using Reinforcement Learning
PROCEEDINGS OF THE 2022 INTERNATIONAL CONFERENCE ON MANAGEMENT OF DATA (SIGMOD '22)(2022)
摘要
Detection and localization of actions in videos is an important problem in practice. State-of-the-art video analytics systems are unable to efficiently and effectively answer such action queries because actions often involve a complex interaction between objects and are spread across a sequence of frames; detecting and localizing them requires computationally expensive deep neural networks. It is also important to consider the entire sequence of frames to answer the query effectively. In this paper, we present ZEUS, a video analytics system tailored for answering action queries. We present a novel technique for efficiently answering these queries using deep reinforcement learning. ZEUS trains a reinforcement learning agent that learns to adaptively modify the input video segments that are subsequently sent to an action classification network. The agent alters the input segments along three dimensions - sampling rate, segment length, and resolution. To meet the user-specified accuracy target, ZEUS'S query optimizer trains the agent based on an accuracy-aware, aggregate reward function. Evaluation on three diverse video datasets shows that ZEUS outperforms state-of-the-art frame- and window-based filtering techniques by up to 22.1x and 4.7x, respectively. It also consistently meets the user-specified accuracy target across all queries.
更多查看译文
关键词
video analytics, video database management systems, action localization, reinforcement learning
AI 理解论文
溯源树
样例
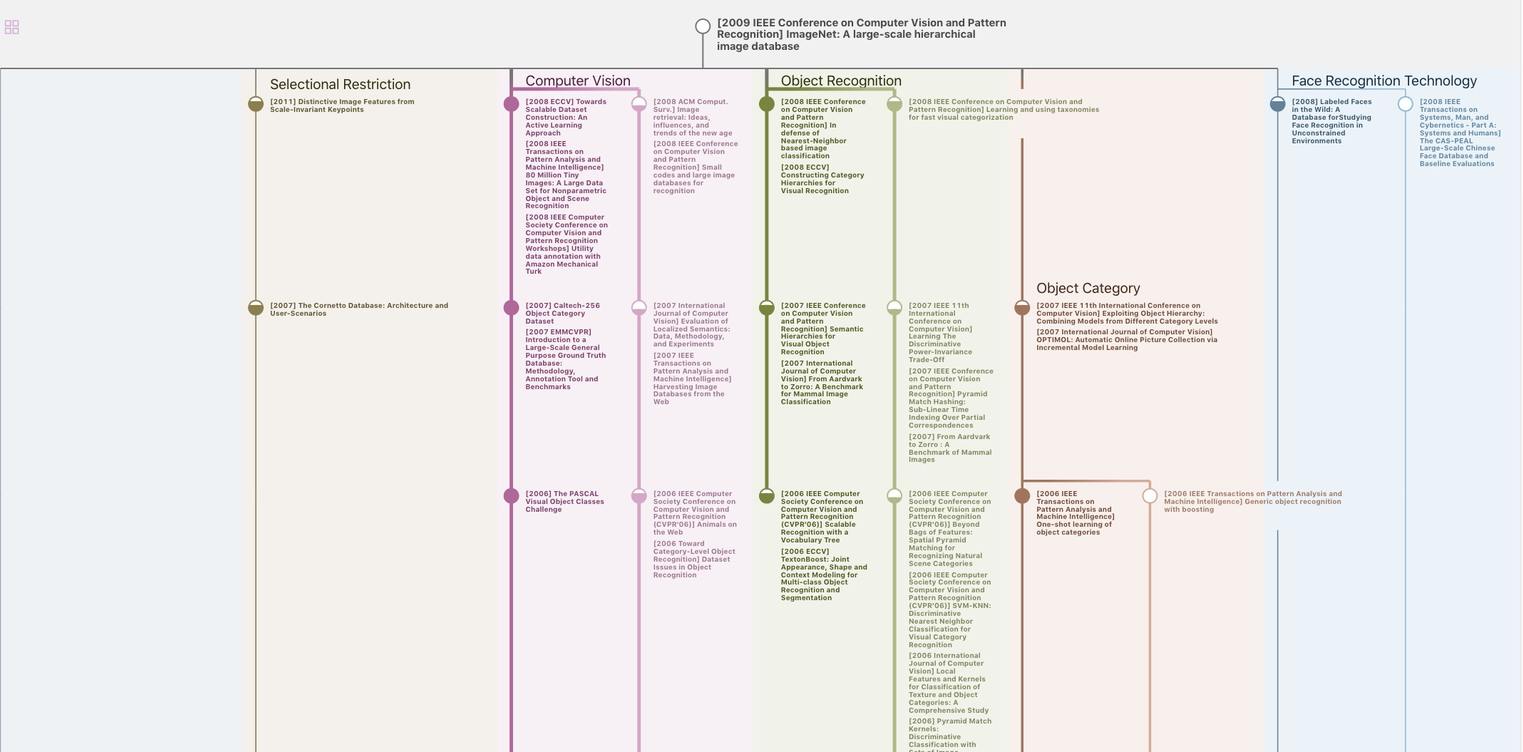
生成溯源树,研究论文发展脉络
Chat Paper
正在生成论文摘要