What's in Your Head? Emergent Behaviour in Multi-Task Transformer Models.
EMNLP(2021)
摘要
The primary paradigm for multi-task training in natural language processing is to represent the input with a shared pre-trained language model, and add a small, thin network (head) per task. Given an input, a target head is the head that is selected for outputting the final prediction. In this work, we examine the behaviour of non-target heads, that is, the output of heads when given input that belongs to a different task than the one they were trained for. We find that non-target heads exhibit emergent behaviour, which may either explain the target task, or generalize beyond their original task. For example, in a numerical reasoning task, a span extraction head extracts from the input the arguments to a computation that results in a number generated by a target generative head. In addition, a summarization head that is trained with a target question answering head, outputs query-based summaries when given a question and a context from which the answer is to be extracted. This emergent behaviour suggests that multi-task training leads to non-trivial extrapolation of skills, which can be harnessed for interpretability and generalization.
更多查看译文
关键词
transformer,emergent behaviour,models,multi-task
AI 理解论文
溯源树
样例
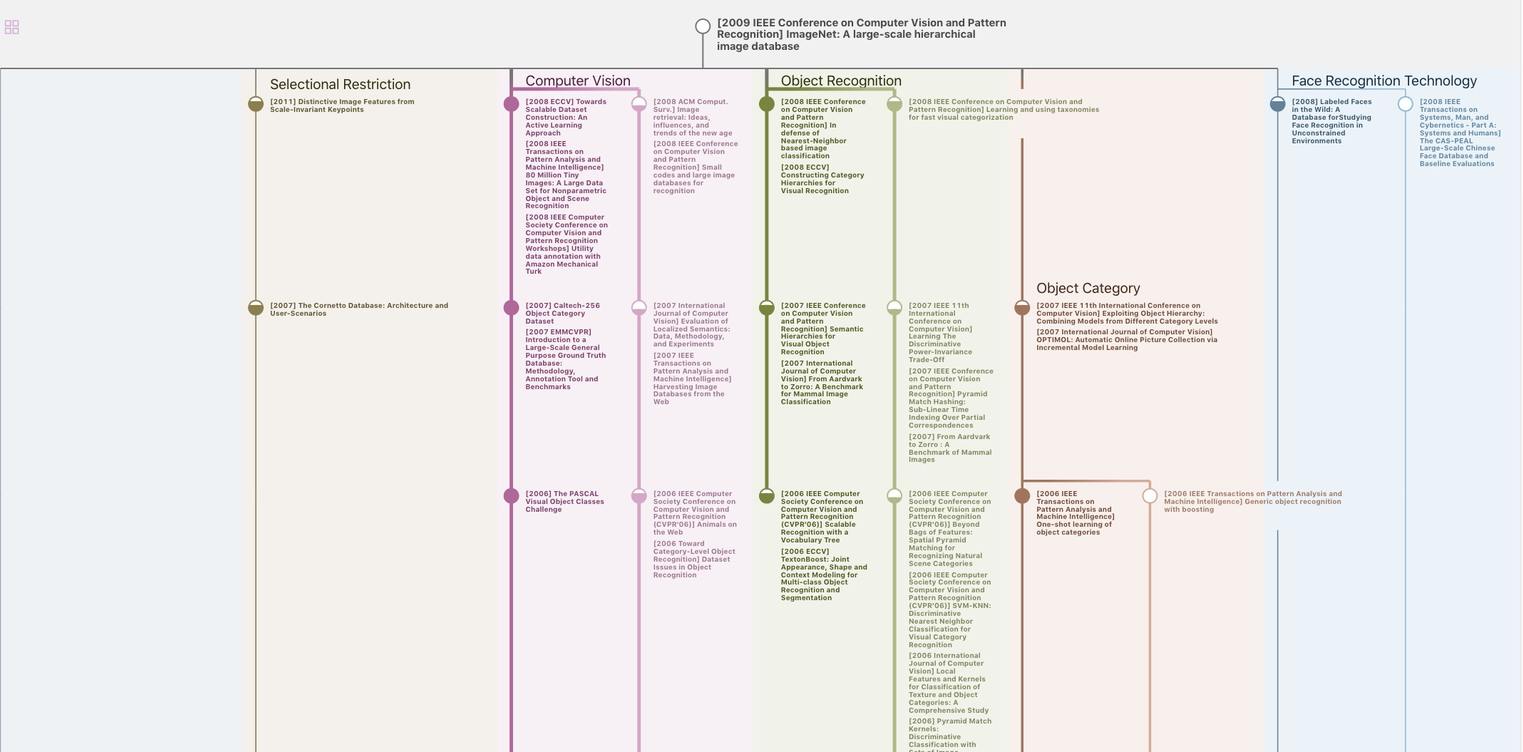
生成溯源树,研究论文发展脉络
Chat Paper
正在生成论文摘要