Object-Centric Representation Learning for Video Question Answering
2021 INTERNATIONAL JOINT CONFERENCE ON NEURAL NETWORKS (IJCNN)(2021)
摘要
Video question answering (Video QA) presents a powerful testbed for human-like intelligent behaviors. The task demands new capabilities to integrate video processing, language understanding, binding abstract linguistic concepts to concrete visual artifacts, and deliberative reasoning over space-time. Neural networks offer a promising approach to reach this potential through learning from examples rather than handcrafting features and rules. However, neural networks are predominantly feature-based - they map data to unstructured vectorial representation and thus can fall into the trap of exploiting shortcuts through surface statistics instead of true systematic reasoning seen in symbolic systems. To tackle this issue, we advocate for object-centric representation as a basis for constructing spatio-temporal structures from videos, essentially bridging the semantic gap between low-level pattern recognition and high-level symbolic algebra. To this end, we propose a new query-guided representation framework to turn a video into an evolving relational graph of objects, whose features and interactions are dynamically and conditionally inferred. The object lives are then summarized into resumes, lending naturally for deliberative relational reasoning that produces an answer to the query. The framework is evaluated on major Video QA datasets, demonstrating clear benefits of the object-centric approach to video reasoning.
更多查看译文
关键词
intelligent behaviors,video processing,language understanding,visual artifacts,deliberative reasoning,neural networks,unstructured vectorial representation,systematic reasoning,symbolic systems,object-centric representation,pattern recognition,symbolic algebra,query-guided representation framework,deliberative relational reasoning,video QA datasets,object-centric approach,video reasoning,abstract linguistic concepts,video question answering,relational graph,surface statistics
AI 理解论文
溯源树
样例
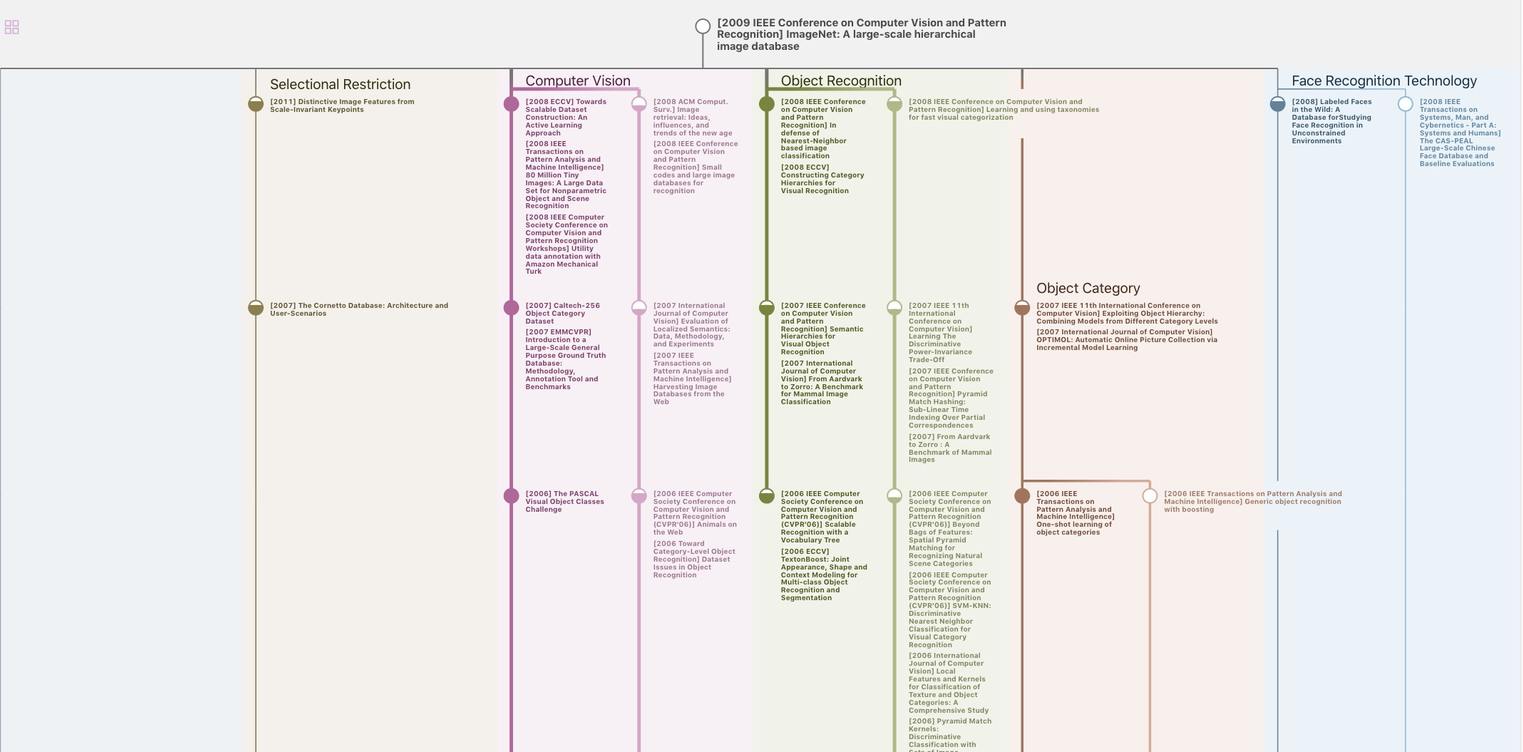
生成溯源树,研究论文发展脉络
Chat Paper
正在生成论文摘要