Understanding event-generation networks via uncertainties
SCIPOST PHYSICS(2022)
摘要
Following the growing success of generative neural networks in LHC simulations, the crucial question is how to control the networks and assign uncertainties to their event output. We show how Bayesian normalizing flows or invertible networks capture uncertainties from the training and turn them into an uncertainty on the event weight. Fundamentally, the interplay between density and uncertainty estimates indicates that these networks learn functions in analogy to parameter fits rather than binned event counts.
更多查看译文
关键词
uncertainties,networks,event-generation
AI 理解论文
溯源树
样例
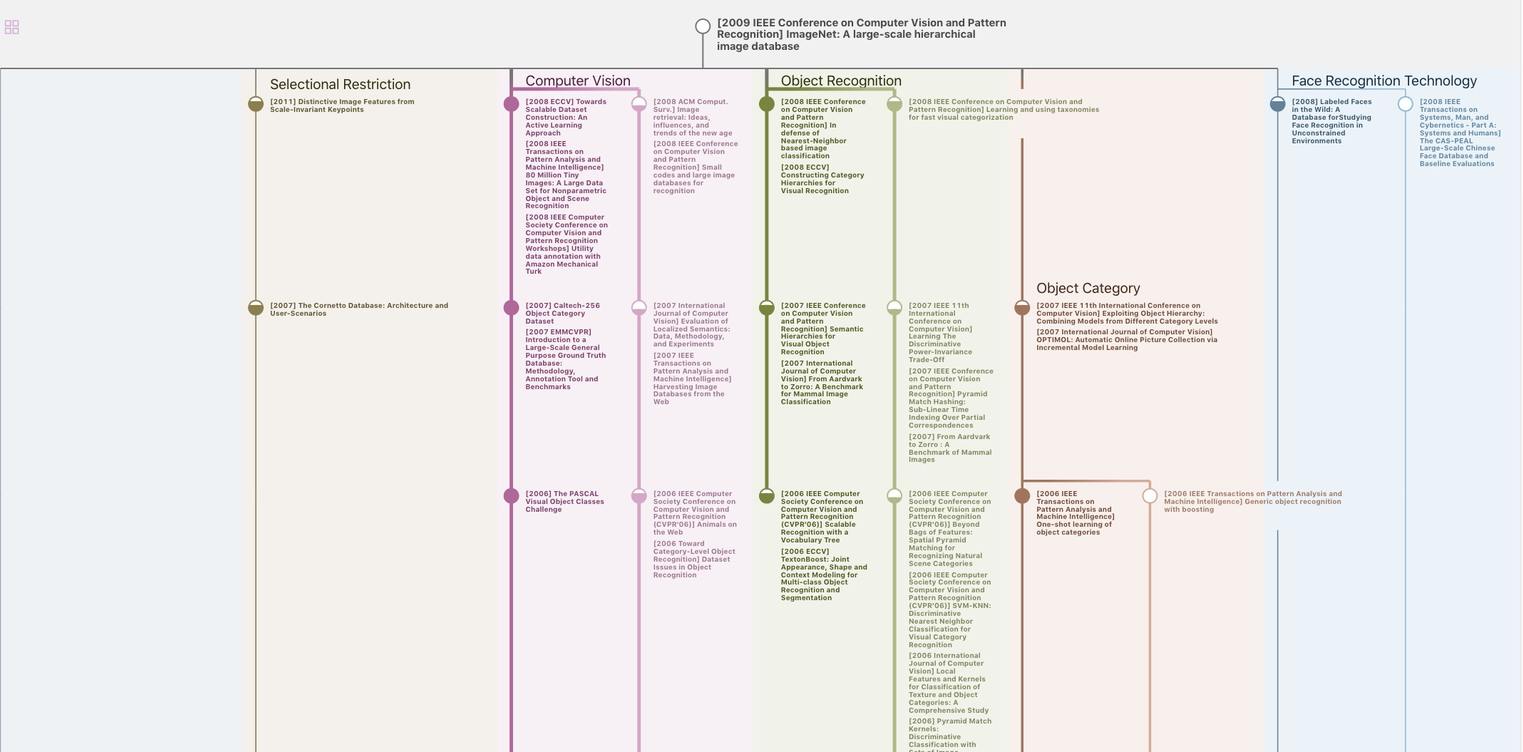
生成溯源树,研究论文发展脉络
Chat Paper
正在生成论文摘要