Inverse Design Of Acoustic Metamaterials Based On Machine Learning Using A Gauss-Bayesian Model
JOURNAL OF APPLIED PHYSICS(2020)
摘要
Acoustic metamaterials (AMs) have attracted a substantial amount of attention in recent decades where the parameter design plays an important role. However, conventional design methods generally rely on analytical physical models and require a very large number of evaluations of acoustic performance. Here, we propose and experimentally demonstrate an inverse-design method for AMs based on machine learning using a Gauss-Bayesian model. As a result of the cycle of training and prediction and the use of adaptive acquisition functions, this method allows the parameters of AMs to be efficiently designed for specific functionalities without the need for physical models. Considering the significance of low-frequency ventilated sound absorption, we present a design for a typical acoustic metamaterial absorber with multiple structural parameters that facilitate high sound absorption at low frequencies. In the design process, the parameters were adaptively adjusted to improve the sound absorption performance at low frequencies using only 37 evaluations, and this high absorption performance was verified by the agreement of numerical and experimental results. Because of its low cost, high flexibility, and independence from physical models, this method paves the way for tremendous opportunities in the design of various AMs for particular desired functionalities.
更多查看译文
关键词
acoustic metamaterials,inverse design,machine learning,gauss–bayesian
AI 理解论文
溯源树
样例
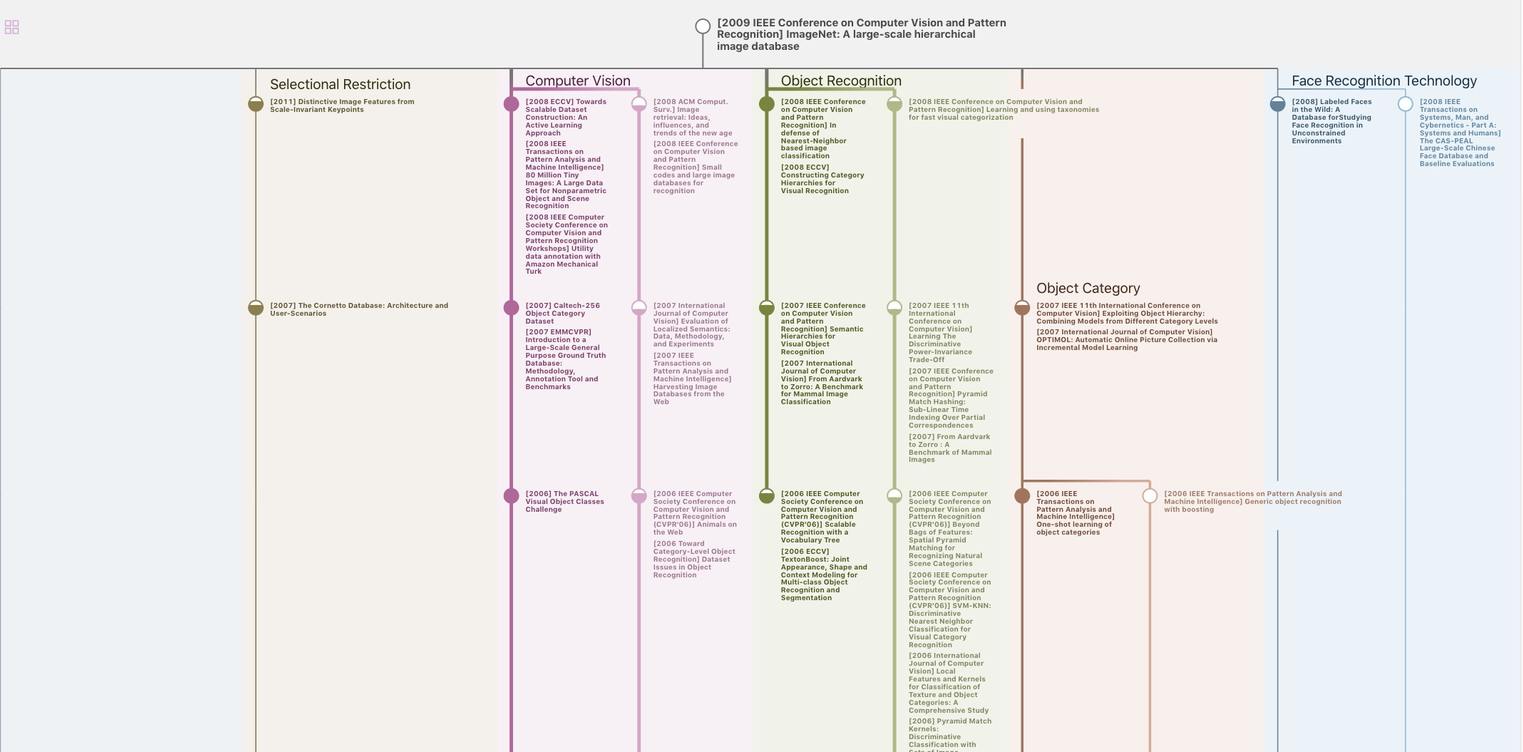
生成溯源树,研究论文发展脉络
Chat Paper
正在生成论文摘要