CondenseNet V2: Sparse Feature Reactivation for Deep Networks
2021 IEEE/CVF CONFERENCE ON COMPUTER VISION AND PATTERN RECOGNITION, CVPR 2021(2021)
摘要
Reusing features in deep networks through dense connectivity is an effective way to achieve high computational efficiency. The recent proposed CondenseNet [14] has shown that this mechanism can befiirther improved if redundant features are removed. In this paper, we propose an alternative approach named sparse feature reactivation (SFR), aiming at actively increasing the utility of features for reusing. In the proposed network, named CondenseNetV2, each layer can simultaneously learn to 1) selectively reuse a set of most important features from preceding layers; and 2) actively update a set of preceding features to increase their utility for later layers. Our experiments show that the proposed models achieve promising performance on image classification (ImageNet and CIFAR) and object detection (MS COCO) in terms of both theoretical efficiency and practical speed.
更多查看译文
关键词
sparse feature reactivation,deep networks,v2
AI 理解论文
溯源树
样例
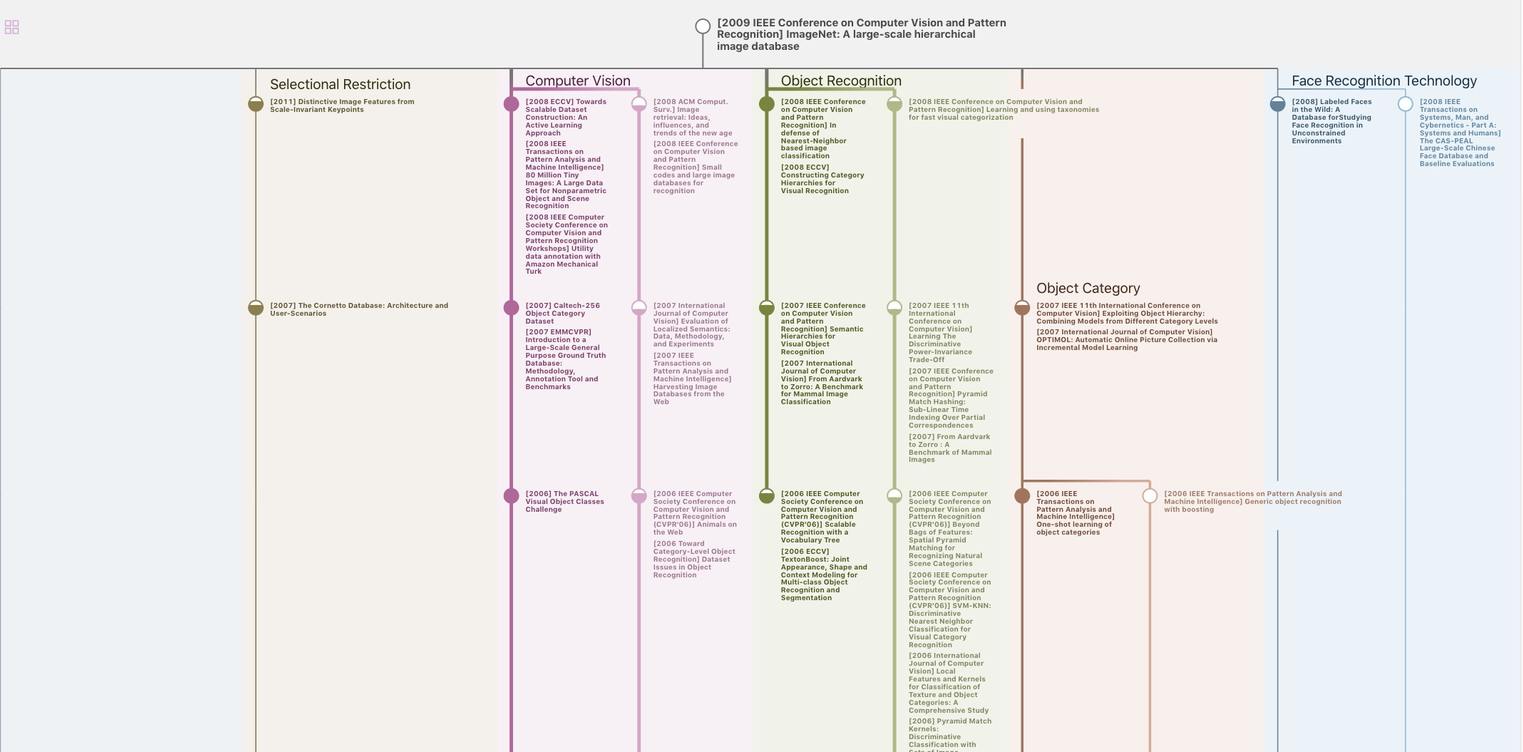
生成溯源树,研究论文发展脉络
Chat Paper
正在生成论文摘要