Creating Cybersecurity Knowledge Graphs From Malware After Action Reports
IEEE ACCESS(2020)
摘要
After Action Reports (AARs) provide incisive analysis of cyber-incidents. Extracting cyber-knowledge from these sources would provide security analysts with credible information, which they can use to detect or find patterns indicative of a cyber-attack. In this paper, we describe a system to extract information from AARs, aggregate the extracted information by fusing similar entities together, and represent that extracted information in a Cybersecurity Knowledge Graph (CKG). We extract entities by building a customized named entity recognizer called 'Malware Entity Extractor' (MEE). We then build a neural network to predict how pairs of 'malware entities' are related to each other. When we have predicted entity pairs and the relationship between them, we assert the 'entity-relationship set' in a CKG. Our next step in the process is to fuse similar entities, to improve our CKG. This fusion helps represent intelligence extracted from multiple documents and reports. The fused CKG has knowledge from multiple AARs, with relationships between entities extracted from separate reports. As a result of this fusion, a security analyst can execute queries and retrieve better answers on the fused CKG, than a knowledge graph with no fusion. We also showcase various reasoning capabilities that can be leveraged by a security analyst using our fused CKG.
更多查看译文
关键词
Computer security, Security, Malware, Data mining, Blogs, Pipelines, Companies, Artificial intelligence, computer security, cyber threat intelligence, after action reports, knowledge graphs, semantic web
AI 理解论文
溯源树
样例
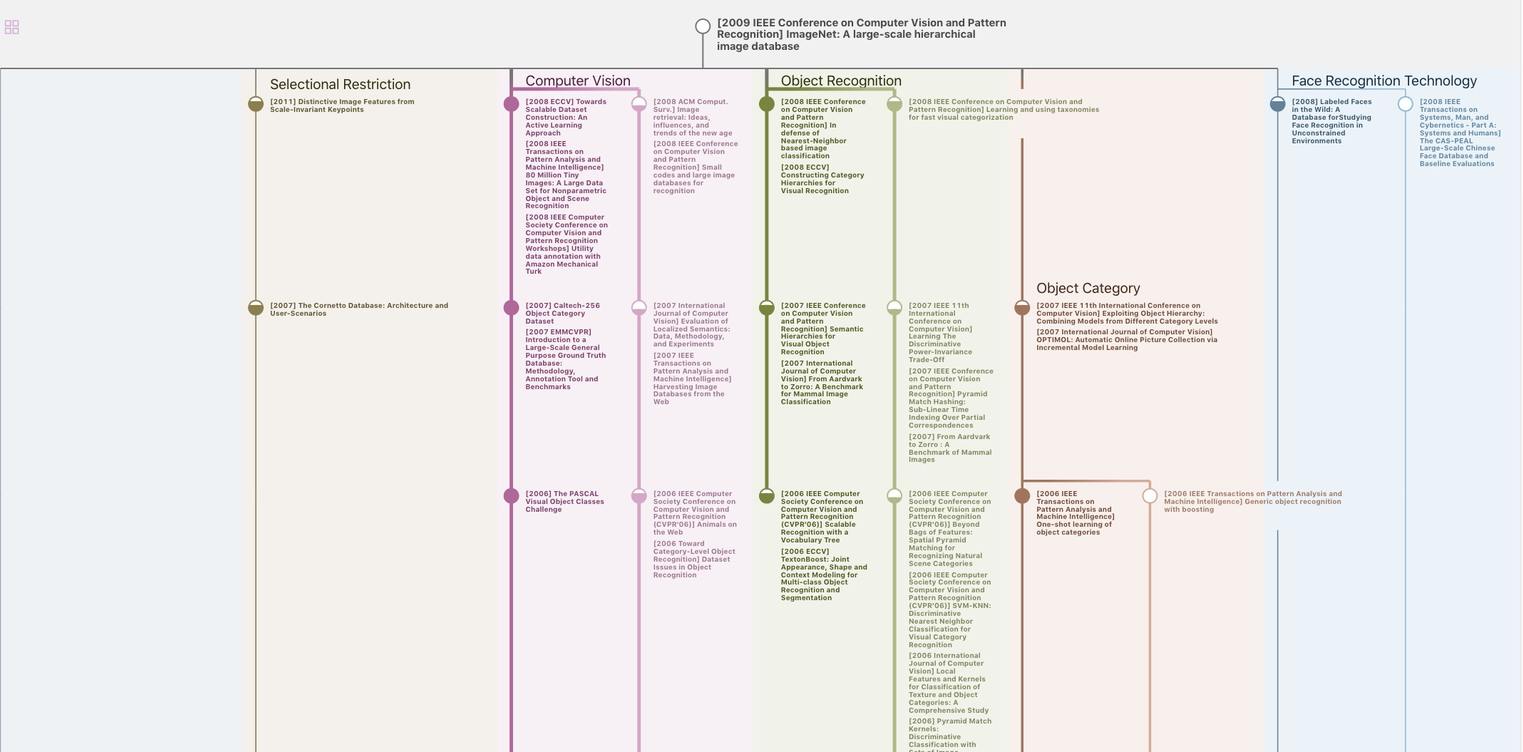
生成溯源树,研究论文发展脉络
Chat Paper
正在生成论文摘要