A/B Testing With Fat Tails
JOURNAL OF POLITICAL ECONOMY(2020)
摘要
We propose a new framework for optimal experimentation, which we term the "A/B testing problem." Our model departs from the existing literature by allowing for fat tails. Our key insight is that the optimal strategy depends on whether most gains accrue from typical innovations or from rare, unpredictable large successes. If the tails of the unobserved distribution of innovation quality are not too fat, the standard approach of using a few high-powered "big" experiments is optimal. However, if the distribution is very fat tailed, a "lean" strategy of trying more ideas, each with possibly smaller sample sizes, is preferred. Our theoretical results, along with an empirical analysis of Microsoft Bing's EXP platform, suggest that simple changes to business practices could increase innovation productivity.
更多查看译文
AI 理解论文
溯源树
样例
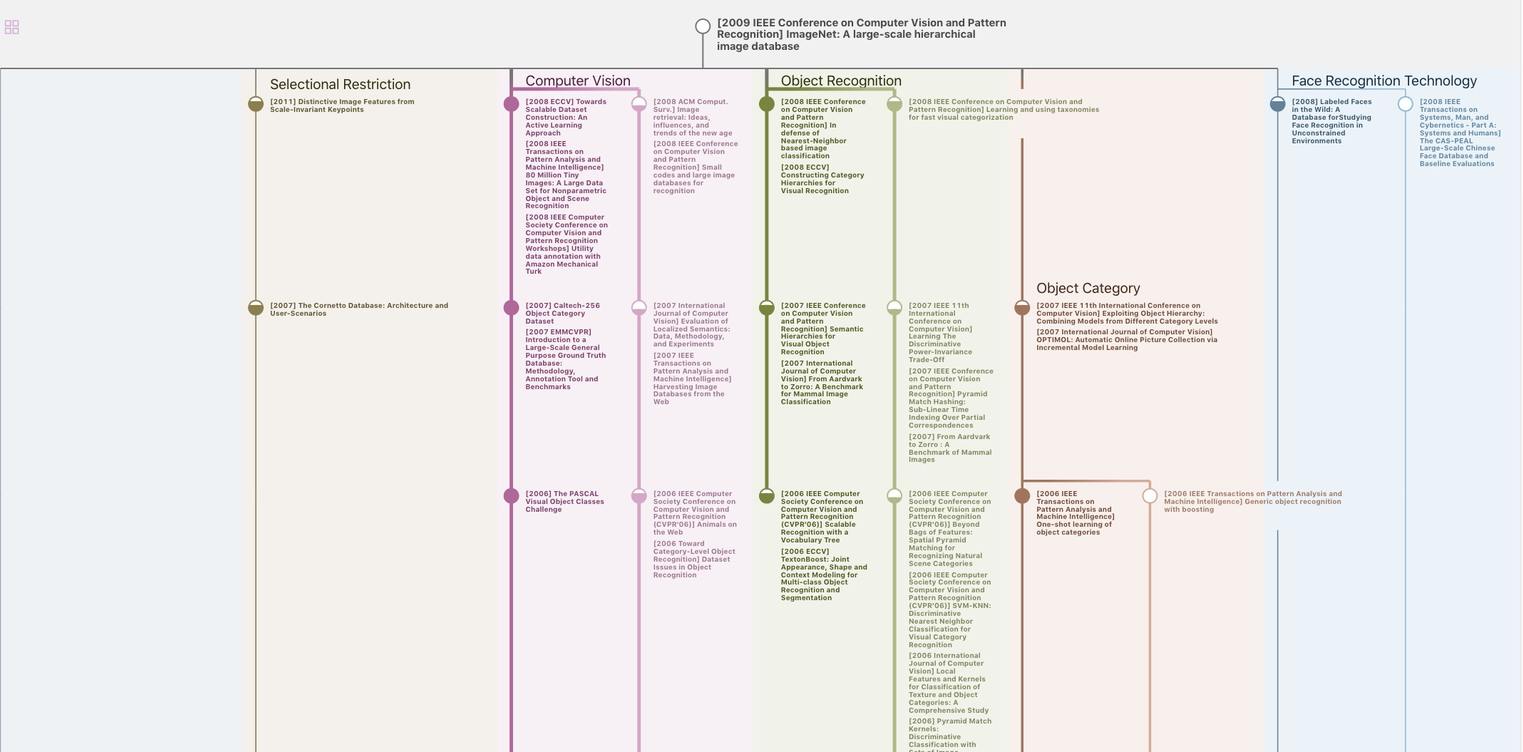
生成溯源树,研究论文发展脉络
Chat Paper
正在生成论文摘要