Deep Learning for Human Activity Recognition
Neurocomputing(2020)
摘要
This chapter focuses on the problem of human activity recognition (HAR), in which inputs in the form of multichannel time series signals are acquired from a set of body-worn wearable sensors and outputs are predefined human activities. In this problem, extracting effective features for identifying activities is a critical but challenging task. Most existing work relies on heuristic hand-crafted feature design and shallow feature learning architectures, which cannot find very discriminative features to accurately classify different activities. In this chapter, we propose a systematic feature learning method for the HAR problem. This method adopts a deep convolutional neural network (CNN) to automate feature learning from the raw inputs in a systematic way. Through the deep architecture, higher level abstract representations of low level raw time series signals are learned as effective features without the need for hand-crafting features. By leveraging the labeled information via supervised learning, the learned features are endowed with more discriminative power. Such a unification of feature learning and classification results in mutual enhancements in both. These unique advantages of the CNN lead to a mutually enhanced outcome of HAR, as verified in the experiments on multiple HAR datasets and comparisons with several state-of-the-art techniques.
更多查看译文
AI 理解论文
溯源树
样例
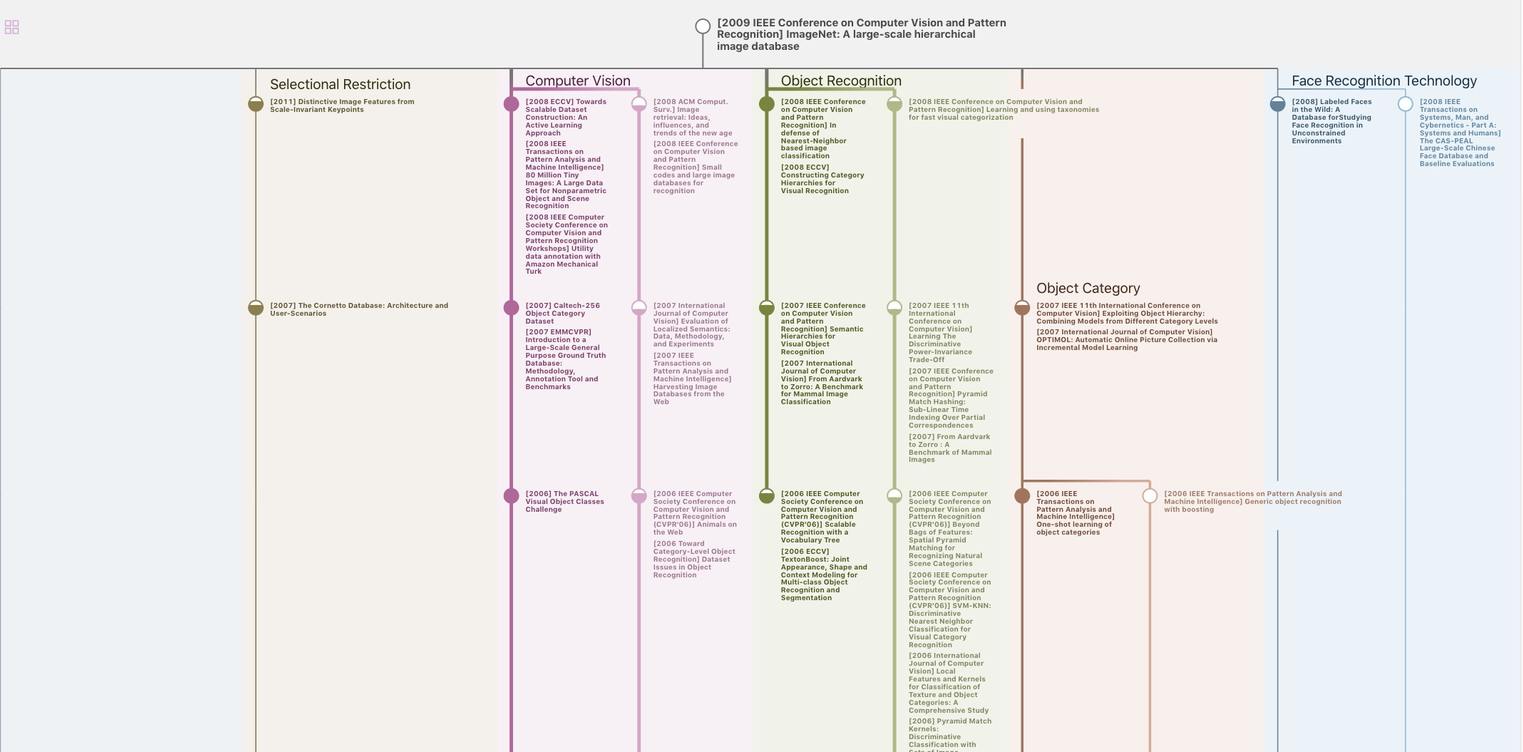
生成溯源树,研究论文发展脉络
Chat Paper
正在生成论文摘要