Learning Similarity-Preserving Meta-Embedding For Text Mining
2020 IEEE INTERNATIONAL CONFERENCE ON BIG DATA (BIG DATA)(2020)
摘要
Publicly available pre-trained word embeddings are rich sources for turning critical high-dimensional representations of huge text data repositories into meaningful compact vectors essential for text mining applications. With many of such pre trained embedding sources available, each faces limitations in the appropriateness of their language use for the downstream text-mining tasks. Meta-embeddings aim to tackle this ambiguity challenge by fusing multiple embedding sources into one feature space. However, current meta-embedding methods assume vocabularies across sources are similar or even identical; which unfortunately stands in sharp contrast to the fact that many sources barely overlap. Further, these methods encode a meta embedding for each word by reconstructing its actual embedding values (word-encoder), while valuable information of relationships (distances) among words within each source are not directly considered. In this work, we instead propose a novel relation-encoder learning approach called Similarity-Preserving Meta-Embedding (SimME) that directly integrates word-pair relationships from partially overlapping embedding sources. SimME embeds words such that their similarities are learned from those observed in multiple pre-trained sources. To handle relations between words that are not present in all sources, we introduce maskotn, a new loss term, that steers the learning selectively to the sources containing said relations. SimME consistently outperforms state-of-the-art methods by 10% on average and with up to 20% across several core metrics in 4 popular mining tasks on 23 datasets.
更多查看译文
关键词
Meta-Embeddings, Text Mining, Word Representations, Semantics Preserving
AI 理解论文
溯源树
样例
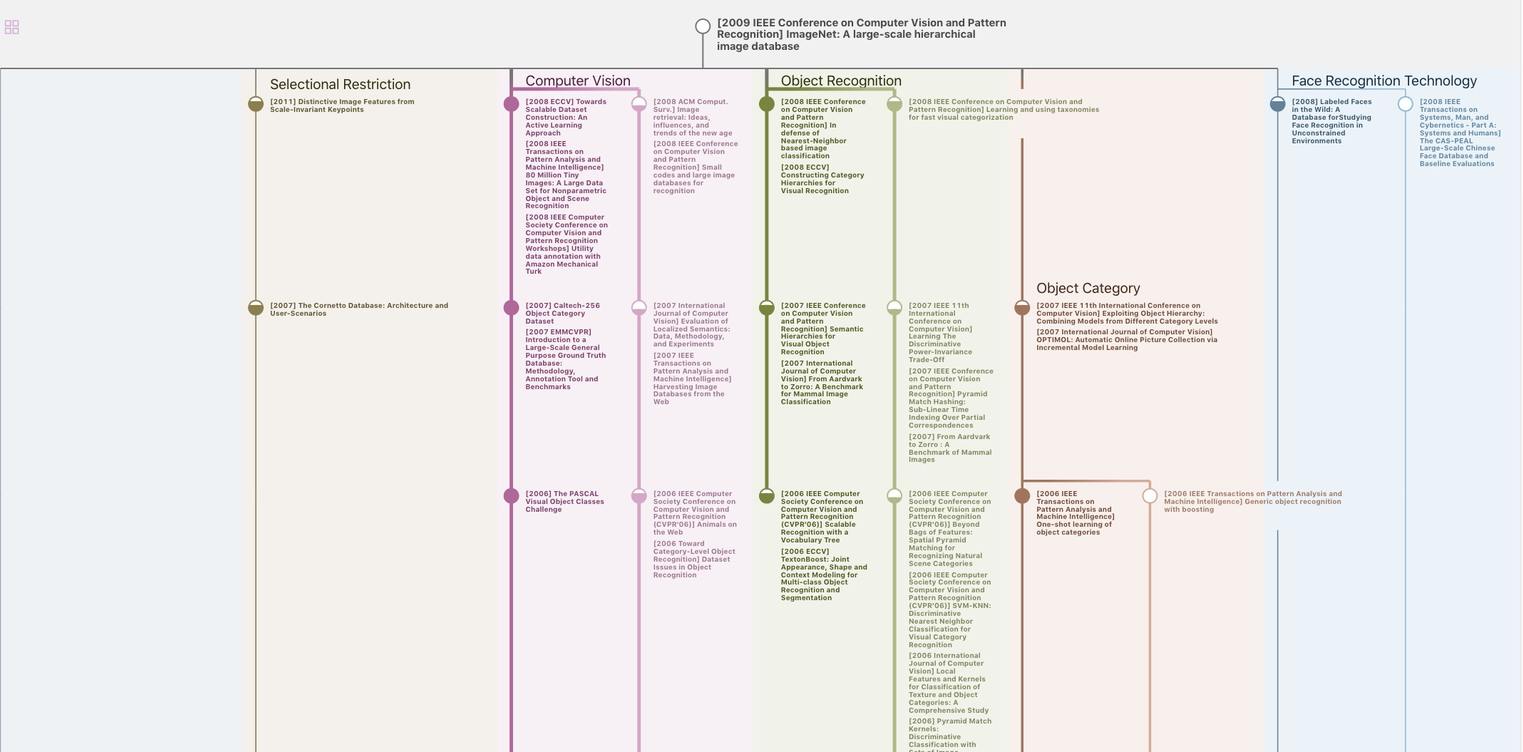
生成溯源树,研究论文发展脉络
Chat Paper
正在生成论文摘要