MBead: Semi-supervised Multilabel Behaviour Anomaly Detection on Multivariate Temporal Sensory Data
2020 IEEE INTERNATIONAL CONFERENCE ON BIG DATA (BIG DATA)(2020)
摘要
Human abnormal physical and psychological behaviors, such as high level of stress, may result in negative impacts on work and life, if not handled efficiently. However, the continuous collection of behavioral data from questionnaires is not feasible, as is often the case for the natural downside of survey data gathering. Thanks to the proliferation of mobile sensors, it brings compelling opportunities for us to more deeply analyze human behavior. In this work, we ask the question of detecting anomalies in human physical and psychological behaviors from multivariate temporal data from multi-modal sensors. In the past decades, many efforts have been made in developing anomaly detection methods, but there remain several challenges in this specific domain problem: 1) data contains missing values at random positions. 2) data from multiple sensors is of multi-resolution and multivariate. 3) human behaviors are correlated to each other, thus it poses a multi-label problem. 4) the available labeled instances are limited, which requires the semi-supervised learning setting. 5) the frequency of anomaly occurrence is much smaller than that of normal instances, leading to imbalance problems. We propose a novel framework MBead to resolve these concerns. MBead consists of three key components: reweighted autoencoder to capture the dependency across temporal domain and multiple modalities, relevance learning module to learn the pairwise relations among labeled instances, and temporal prediction module to detect the anomalies while trained in semi-supervised settings. Extensive experiments show our MBead outperforms seven state-of-art baselines on three tasks of behavior anomaly detection: stress, affect, and work performance.
更多查看译文
关键词
anomaly detection, behavior anomaly, multivariate time series, semi-supervised learning
AI 理解论文
溯源树
样例
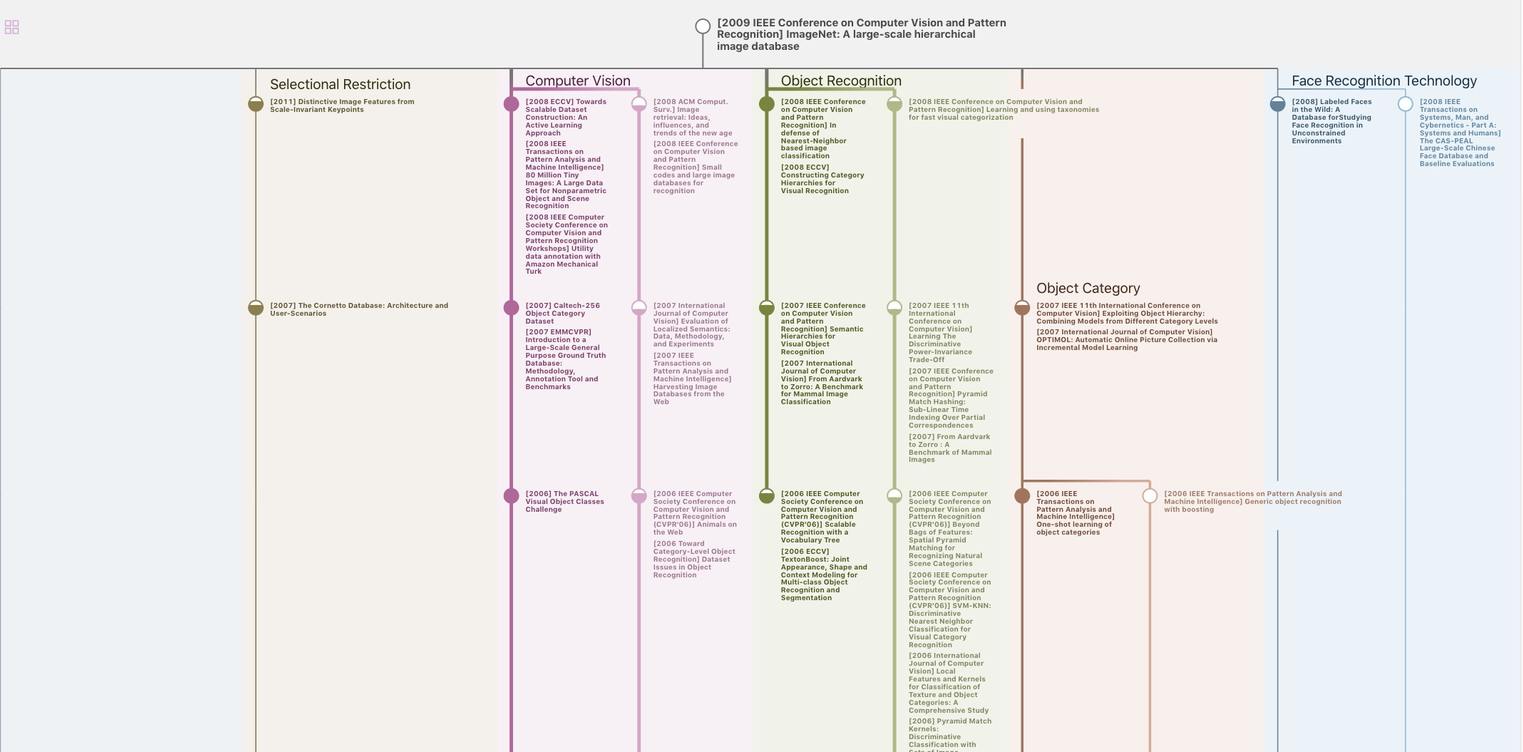
生成溯源树,研究论文发展脉络
Chat Paper
正在生成论文摘要