Data-Driven Template Discovery Using Graph Convolutional Neural Networks
2020 IEEE INTERNATIONAL CONFERENCE ON BIG DATA (BIG DATA)(2020)
摘要
Modeling adversarial activities is a critical component of developing high-confidence indicators of efforts to acquire, fabricate, proliferate, and/or deploy weapons of mass terror (WMTs). Current approaches to generating representative patterns of interest (a.k.a templates) from the real-world domains involve a Subject Matter Expert (SME)-guided manual process. The goal of Data-Driven Template Discovery (DDTD) is to use a (potentially small) set of SME generated templates to discover other previously unknown and interesting templates in an attributed graph. A template is an activity pattern describing a set of interactions among a group of nodes in the graph. The motivation behind DDTD is to expand the original set of templates, without having SMEs craft all the templates by hand. DDTD also provides seed templates to SMEs, to help them construct larger, high-fidelity, and scenario-oriented templates. In these cases, obtaining a larger set of templates that are related (contain similar signals) to the original set is of great value. In this work, we propose to use Graph Convolutional Neural Networks (GCNs) to discover new templates that are heavily related to the original set. GCNs are a family of Neural Network (NN) architectures especially designed to work directly on graphs. In contrast to the traditional NNs, that require considerable amounts of labeled data, GCNs do not require a big labeled training set because they can directly leverage the graph structure instead. This property makes GCNs the perfect tool for creating activity templates.
更多查看译文
关键词
GNN, Knowledge Graph, Template
AI 理解论文
溯源树
样例
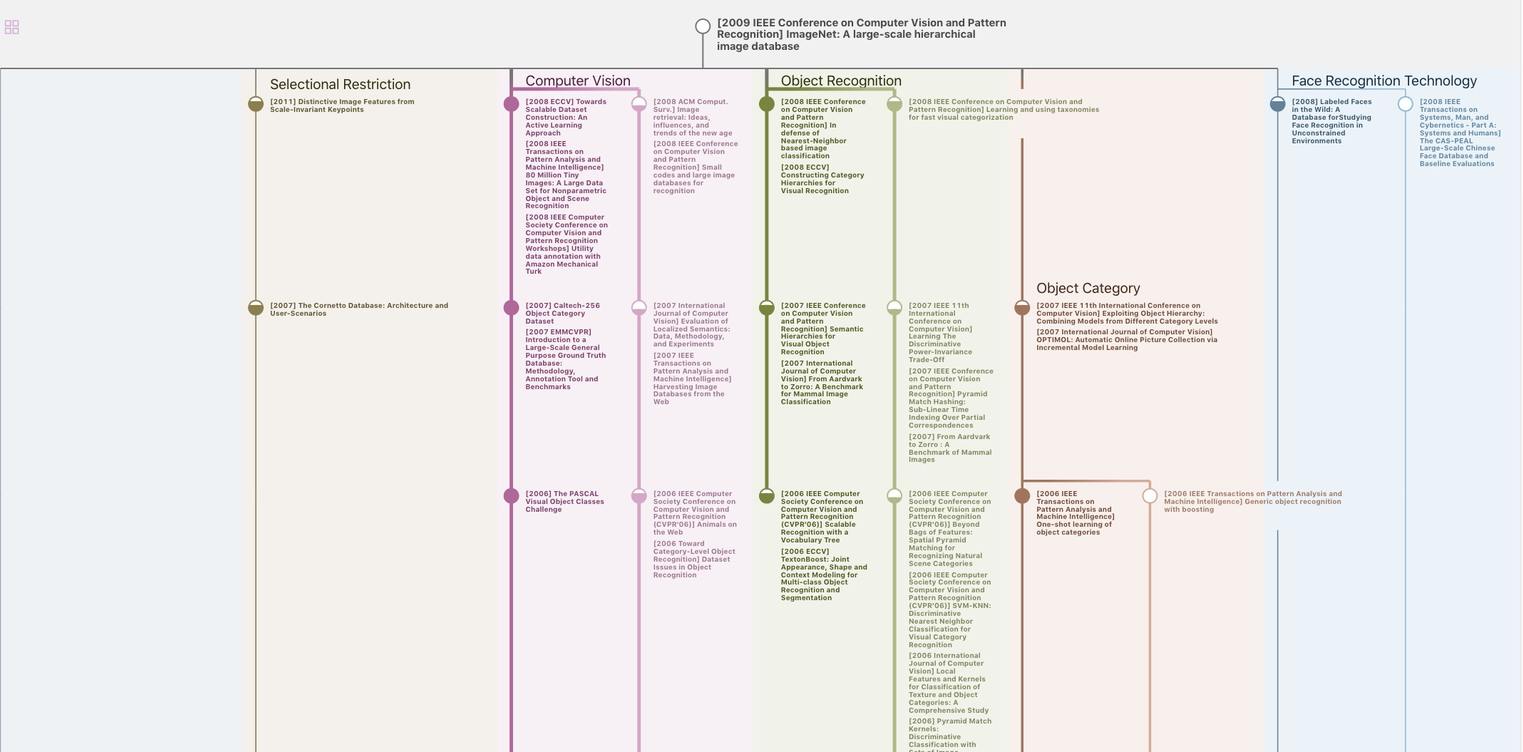
生成溯源树,研究论文发展脉络
Chat Paper
正在生成论文摘要