Hydra: Cancer Detection Leveraging Multiple Heads And Heterogeneous Datasets
2020 IEEE INTERNATIONAL CONFERENCE ON BIG DATA (BIG DATA)(2020)
摘要
We propose an approach combining layer freezing and fine-tuning steps alternatively to train a neural network over multiple and diverse datasets in the context of cancer detection from medical images. Our method explicitly splits the network into two distinct but complementary components: the feature extractor and the decision maker. While the former remains constant throughout training, a different decision maker is used on each new dataset. This enables end-to-end training of the feature extractor on heterogeneous datasets (here MRIs and CT scans) and organs (here prostate, lung and brain). The feature extractor learns features across all images, with two major benefits: (i) extended training data pool, and (ii) enforced generalization across different data. We show the effectiveness of our method by detecting cancerous masses in the SPIE-AAPM-NCI Prostate MR Classification data. Our training process integrates the SPIE-AAPM-NCI Lung CT Classification dataset as well as the Kaggle Brain MRI dataset, each paired with a separate decision maker, improving the AUC of the base network architecture on the Prostate MR dataset by 0.12 (18% relative increase) versus training on the prostate dataset alone. We also compare against standard end-to-end Transfer Learning over the same datasets for reference, which only improves the results by 0.04 (6% relative increase).
更多查看译文
关键词
Deep Learning, Transfer Learning, Prostate Cancer Detection
AI 理解论文
溯源树
样例
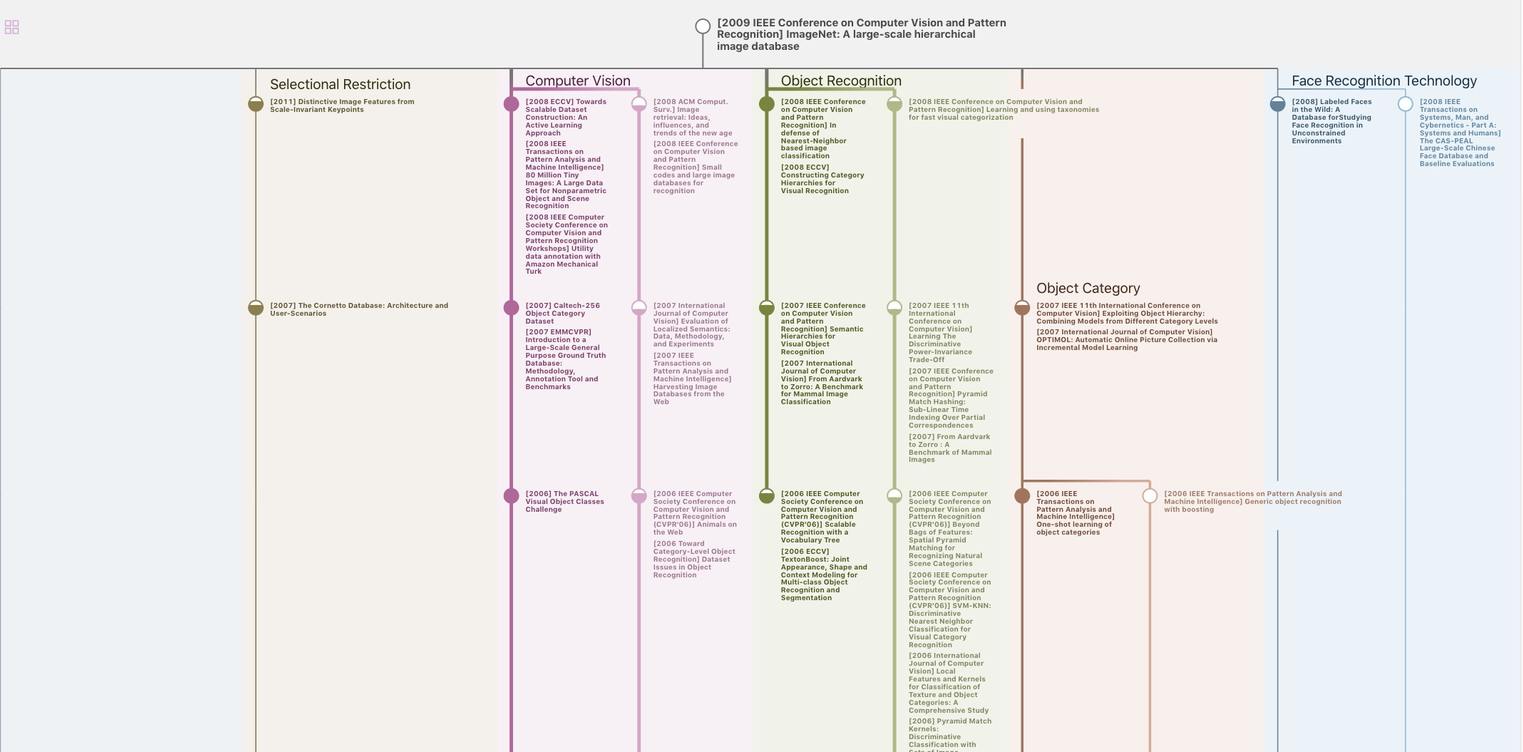
生成溯源树,研究论文发展脉络
Chat Paper
正在生成论文摘要