Correlation-Based Analytics Of Time Series Data
2020 IEEE INTERNATIONAL CONFERENCE ON BIG DATA (BIG DATA)(2020)
摘要
Correlation-based analytics of time series data is important for a wide range of applications from stock analysis to weather forecasting. Yet performing these analytics at the granularity of subsequences of time series is prohibitively costly for large data sets. Our proposed framework CORAL (CORrelation based AnaLytics) tackles this challenge by adopting a preprocess-once and query-many-times paradigm. In a preprocessing step, CORAL compresses time series data into compact clusters, each identified by a unique representative, taking advantage of the Euclidean distance triangle inequality property. Using a mapping between the metric Euclidean distance and non-metric Pearson correlation, we establish inter- and intra-cluster correlation bounds as foundation for CORAL processing. In the CORAL model, inter-cluster correlation relationships are captured as a compact overlay graph with representatives as nodes and the correlation between cluster representatives as edges. The two CORAL bounds support time series matching by realizing the comparison among the cluster representatives at the abstract level in the CORAL graph model in place of the underlying huge collection of raw time series. The resulting CORAL model effectively supports a rich set of analytics operations including retrieval of the best-correlated subsequence, self-correlation, and detection of groups of correlated subsequences. Our comprehensive experimental evaluation on 85 real benchmark datasets demonstrates that CORAL is many fold faster than state-of-the-art systems while returning highly accurate results.
更多查看译文
关键词
Data mining, Time series, Nearest neighbor
AI 理解论文
溯源树
样例
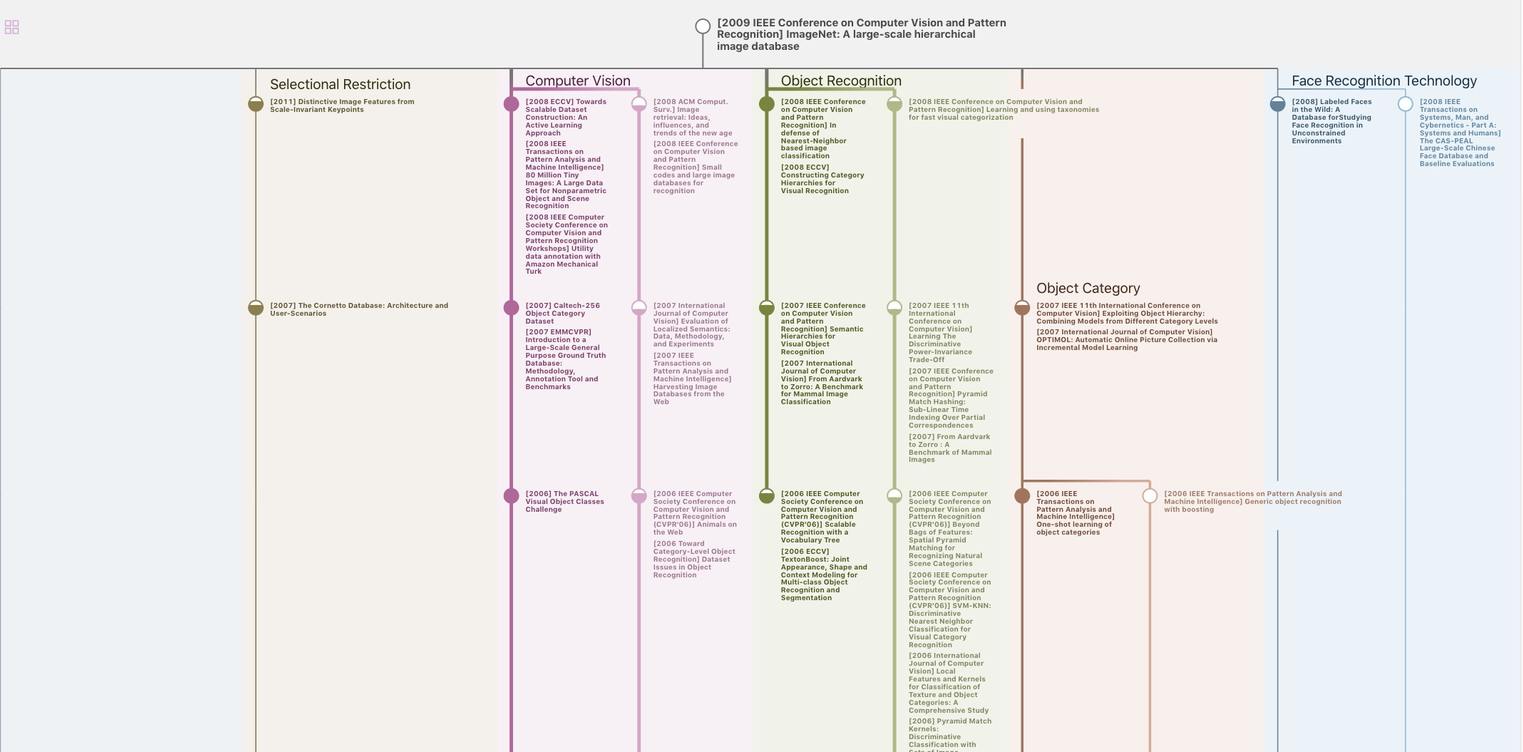
生成溯源树,研究论文发展脉络
Chat Paper
正在生成论文摘要