Field-Aware Matrix Factorization for Recommender Systems.
IEEE ACCESS(2018)
摘要
Predicting user response is one of the core machine learning tasks in recommender systems (RS). The matrix factorization (MF)-based model has been proved to be a useful tool to improve the performance of recommendation. Many existing matrix factorization-based models mainly rely on adding some side information into basic MF to enable the model to fully express the data. However, most of the side information is measured based on the statistics or empirical formula. Also, the latent features of side information cannot be deeply mined. In this paper, we focus on mining the influence of field information (useful side information) to improve the performance of prediction. Based on the MF framework, we propose a field-aware matrix factorization (FMF) model. In FMF, the interactions between user/item and field can be captured and learned in the latent vector spaces. We propose efficient implementations to train FMF. Then, we comprehensively analyze FMF and compare this model with the state-of-the-art models. The analysis of experiments on two large data sets demonstrates that our method is very useful in RS.
更多查看译文
关键词
Recommender systems,matrix factorization,machine learning,field
AI 理解论文
溯源树
样例
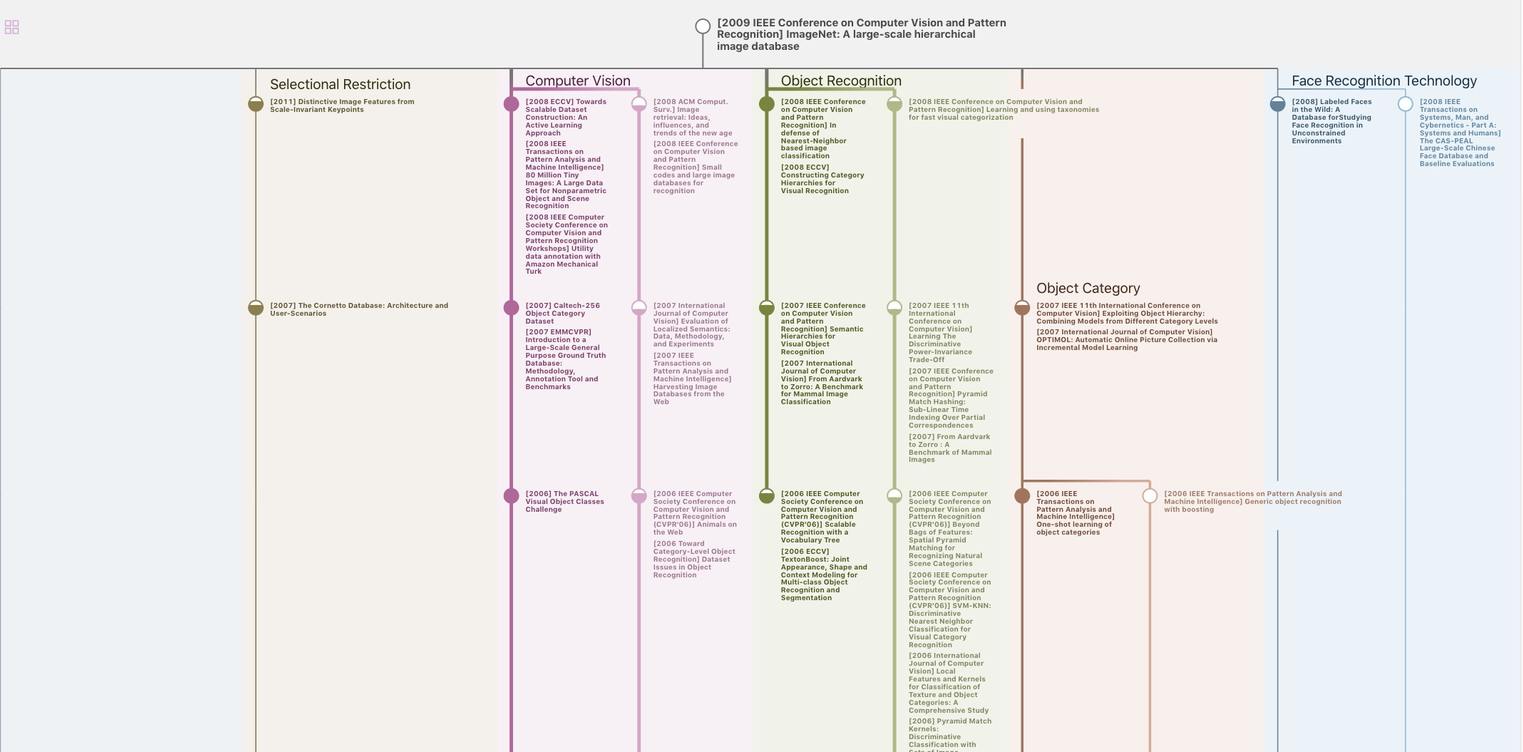
生成溯源树,研究论文发展脉络
Chat Paper
正在生成论文摘要