3D Shape Generation and Completion through Point-Voxel Diffusion
ICCV(2021)
摘要
We propose a novel approach for probabilistic generative modeling of 3D shapes. Unlike most existing models that learn to deterministically translate a latent vector to a shape, our model, Point-Voxel Diffusion (PVD), is a unified, probabilistic formulation for unconditional shape generation and conditional, multi-modal shape completion. PVD marries denoising diffusion models with the hybrid, point-voxel representation of 3D shapes. It can be viewed as a series of denoising steps, reversing the diffusion process from observed point cloud data to Gaussian noise, and is trained by optimizing a variational lower bound to the (conditional) likelihood function. Experiments demonstrate that PVD is capable of synthesizing high-fidelity shapes, completing partial point clouds, and generating multiple completion results from single-view depth scans of real objects.
更多查看译文
关键词
Neural generative models,3D from a single image and shape-from-x
AI 理解论文
溯源树
样例
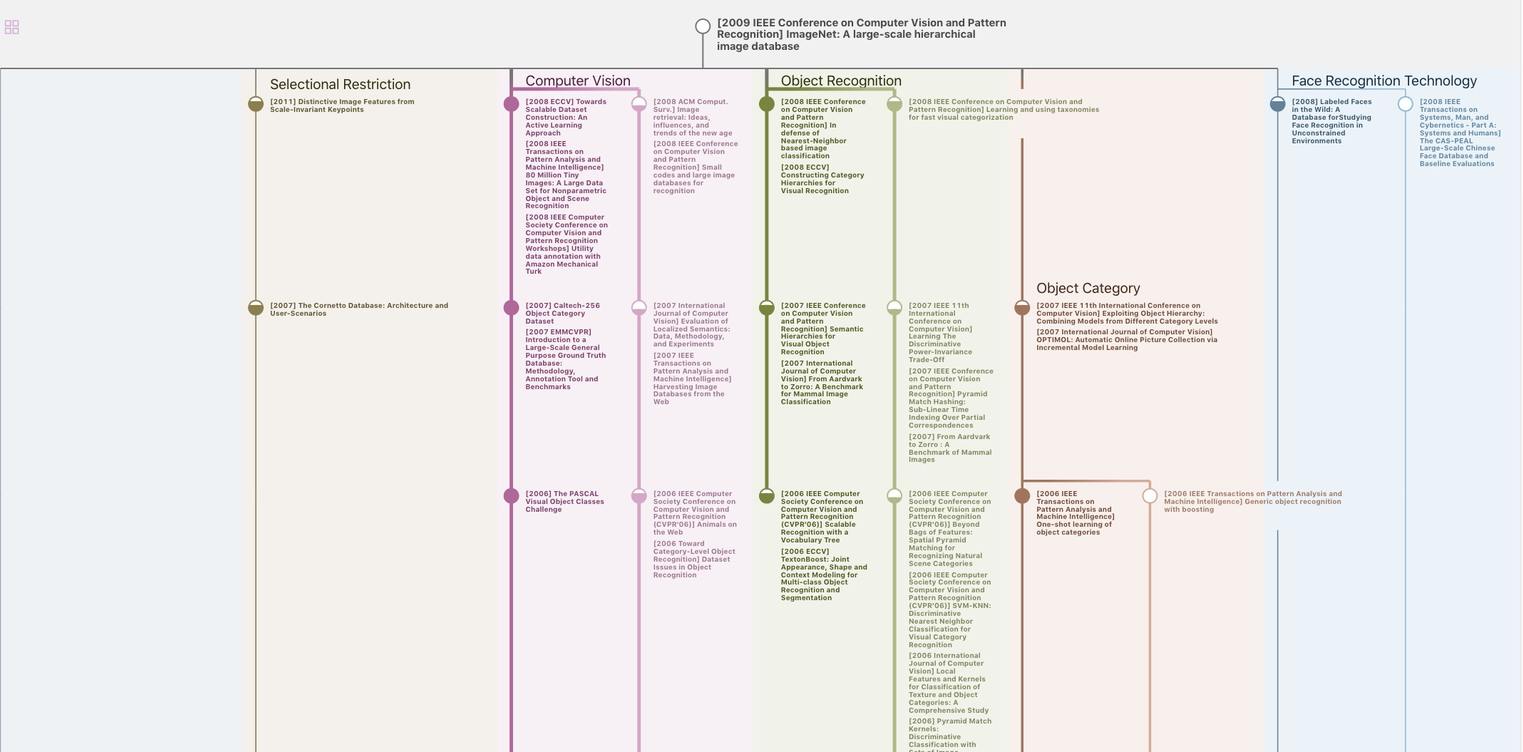
生成溯源树,研究论文发展脉络
Chat Paper
正在生成论文摘要