TenSEAL: A Library for Encrypted Tensor Operations Using Homomorphic Encryption
arxiv(2021)
摘要
Machine learning algorithms have achieved remarkable results and are widely applied in a variety of domains. These algorithms often rely on sensitive and private data such as medical and financial records. Therefore, it is vital to draw further attention regarding privacy threats and corresponding defensive techniques applied to machine learning models. In this paper, we present TenSEAL, an open-source library for Privacy-Preserving Machine Learning using Homomorphic Encryption that can be easily integrated within popular machine learning frameworks. We benchmark our implementation using MNIST and show that an encrypted convolutional neural network can be evaluated in less than a second, using less than half a megabyte of communication.
更多查看译文
关键词
encrypted tensor operations,homomorphic
AI 理解论文
溯源树
样例
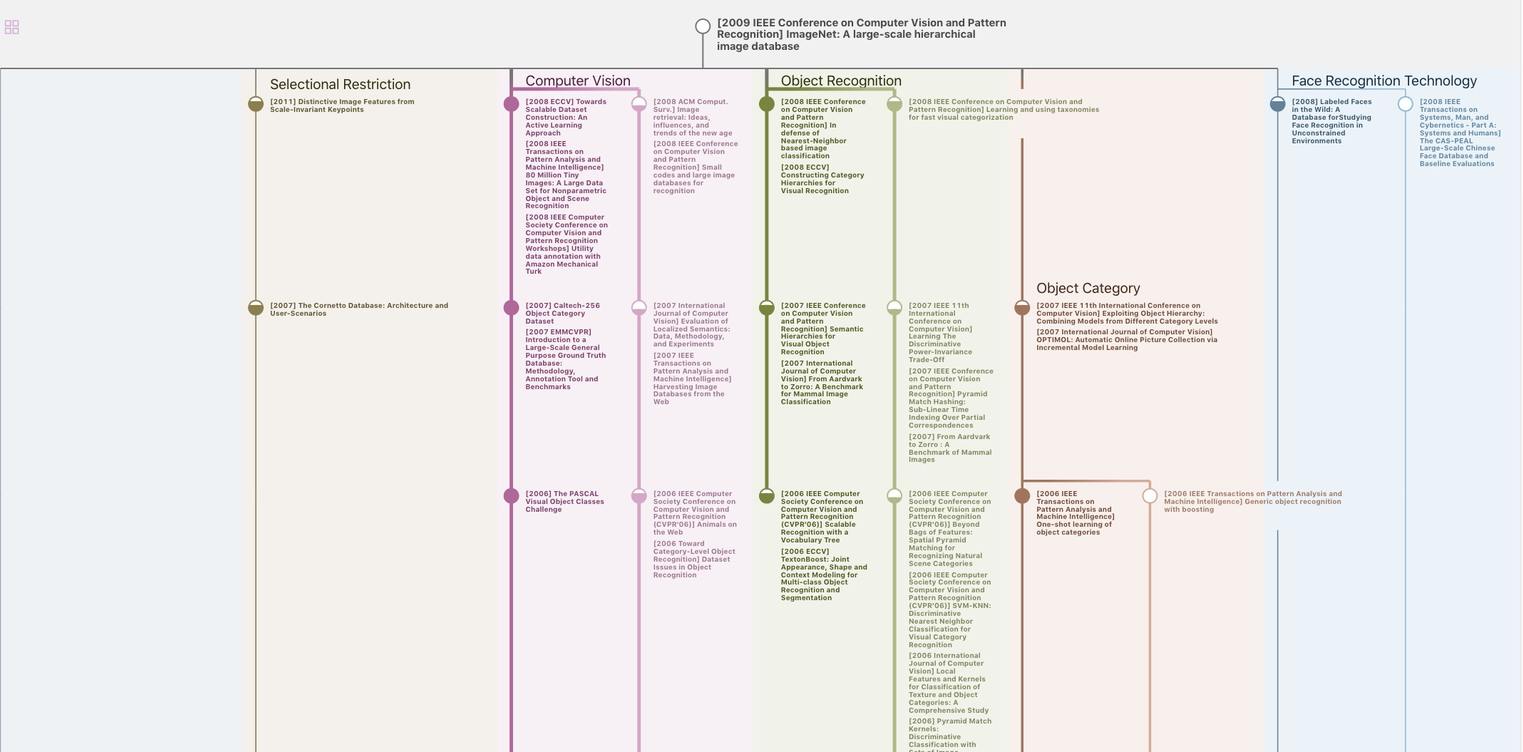
生成溯源树,研究论文发展脉络
Chat Paper
正在生成论文摘要