Global Context-Aware Cervical Cell Detection With Soft Scale Anchor Matching
COMPUTER METHODS AND PROGRAMS IN BIOMEDICINE(2021)
摘要
Background and Objective:Computer-aided cervical cancer screening based on an automated recognition of cervical cells has the potential to significantly reduce error rate and increase productivity compared to manual screening. Traditional methods often rely on the success of accurate cell segmentation and discriminative hand-crafted features extraction. Recently, detector based on convolutional neural network is applied to reduce the dependency on hand-crafted features and eliminate the necessary segmentation. However, these methods tend to yield too much false positive predictions.Methods: This paper proposes a global context-aware framework to deal with this problem, which in-tegrates global context information by an image-level classification branch and a weighted loss. And the prediction of this branch is merged into cell detection for filtering false positive predictions. Furthermore, a new ground truth assignment strategy in the feature pyramid called soft scale anchor matching is pro-posed, which matches ground truths with anchors across scales softly. This strategy searches the most appropriate representation of ground truths in each layer and add more positive samples with different scales, which facilitate the feature learning.Results: Our proposed methods finally get 5.7% increase in mean average precision and 18 . 5% increase in specificity with sacrifice of 2.6% delay in inference time.Conclusions: Our proposed methods which totally avoid the dependence on segmentation of cervical cells, show the great potential to reduce the workload for pathologists in automation-assisted cervical cancer screening. (C) 2021 Elsevier B.V. All rights reserved.
更多查看译文
关键词
Cervical cancer, Object detection, Convolutional neural network, Global context, Ground truth assignment
AI 理解论文
溯源树
样例
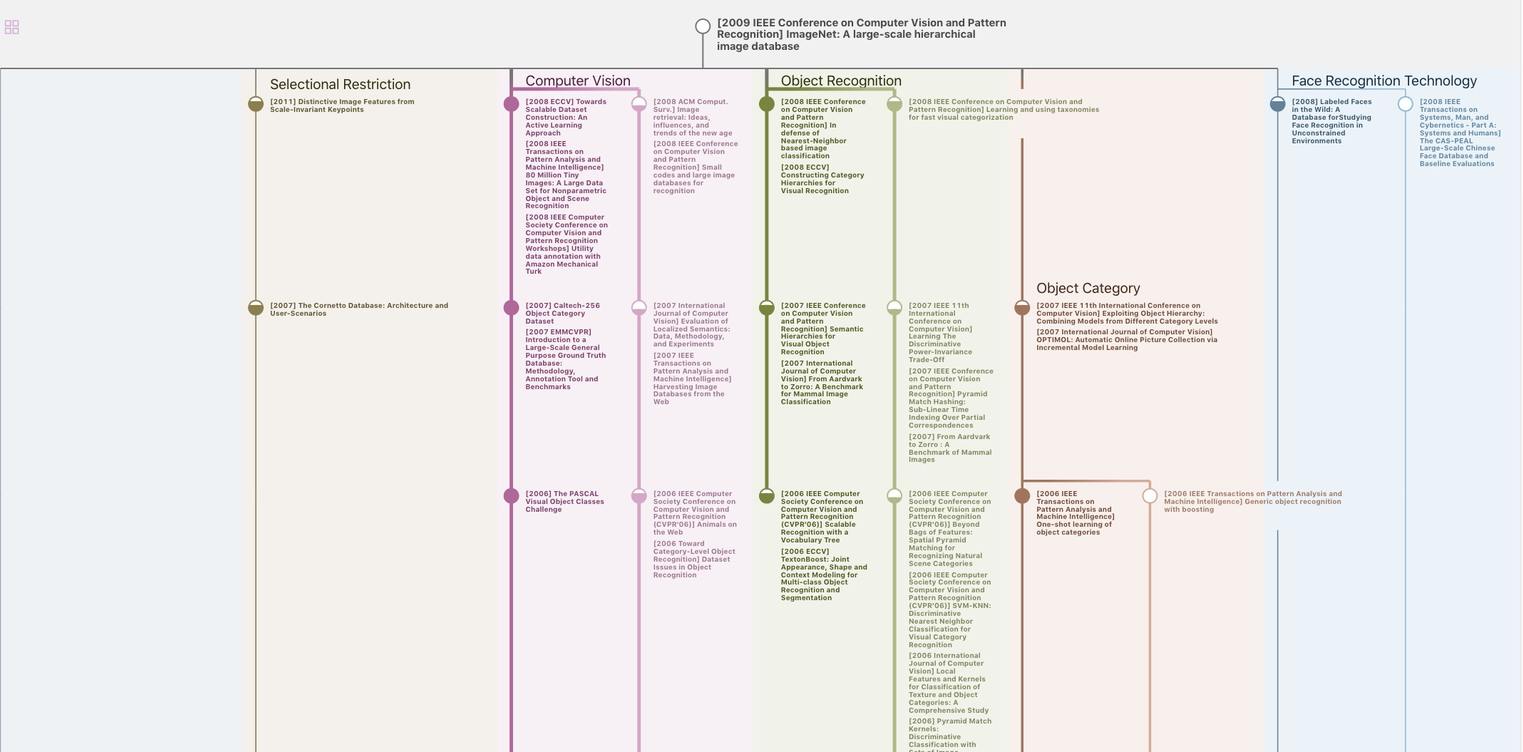
生成溯源树,研究论文发展脉络
Chat Paper
正在生成论文摘要