Semantic Distance - A New Metric for ASR Performance Analysis Towards Spoken Language Understanding.
Interspeech(2021)
摘要
Word Error Rate (WER) has been the predominant metric used to evaluate the performance of automatic speech recognition (ASR) systems. However, WER is sometimes not a good indicator for downstream Natural Language Understanding (NLU) tasks, such as intent recognition, slot filling, and semantic parsing in task-oriented dialog systems. This is because WER takes into consideration only literal correctness instead of semantic correctness, the latter of which is typically more important for these downstream tasks. In this study, we propose a novel Semantic Distance (SemDist) measure as an alternative evaluation metric for ASR systems to address this issue. We define SemDist as the distance between a reference and hypothesis pair in a sentence-level embedding space. To represent the reference and hypothesis as a sentence embedding, we exploit RoBERTa, a state-of-the-art pre-trained deep contextualized language model based on the transformer architecture. We demonstrate the effectiveness of our proposed metric on various downstream tasks, including intent recognition, semantic parsing, and named entity recognition.
更多查看译文
关键词
ASR evaluation metric,spoken language understanding,natural language understanding
AI 理解论文
溯源树
样例
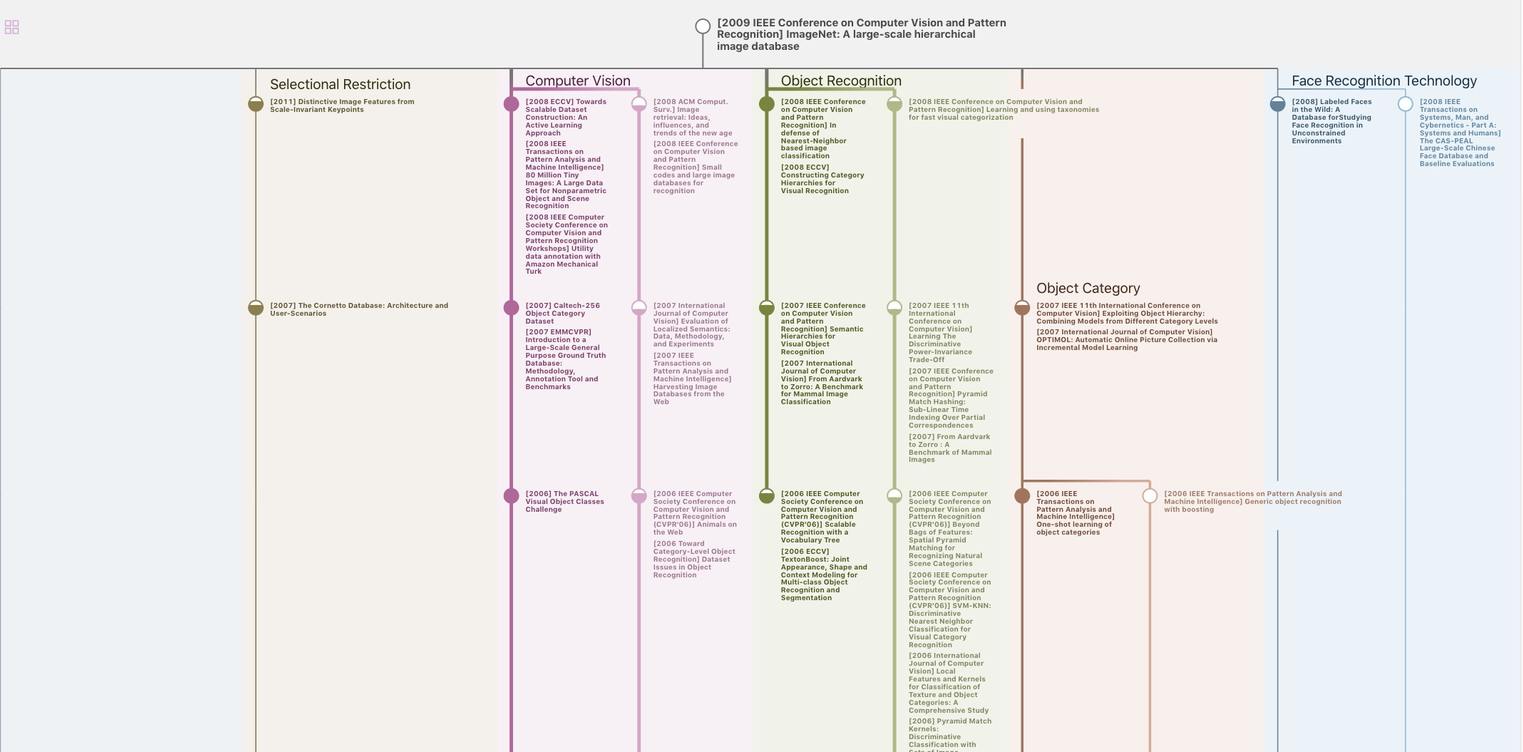
生成溯源树,研究论文发展脉络
Chat Paper
正在生成论文摘要