Scaling to Many Languages with a Triaged Multilingual Text-Dependent and Text-Independent Speaker Verification System
arxiv(2021)
摘要
In this work we study some of the challenges associated with scaling speaker recognition systems to multiple languages. To the best of our knowledge, this is the first study of speaker verification systems at the scale of 46 languages. Training models for each of the many languages can be time and energy demanding in addition to costly. Low resource languages present additional difficulties. The problem is framed from the perspective of using a smart speaker device with interactions consisting of a wake-up keyword (text-dependent) followed by a speech query (text-independent). We examine the use of a hybrid setup consisting of multilingual text-dependent and text-independent components. Experimental evidence suggests that training on multiple languages can generalize to unseen varieties while maintaining performance on seen varieties. We also found that it can reduce computational requirements for training models by an order of magnitude. Furthermore, during model inference on English data, we observe that leveraging a triage framework can reduce the number of calls to the more computationally expensive text-independent system by 73% (and reduce latency by 60%) while maintaining an EER no worse than the text-independent setup.
更多查看译文
AI 理解论文
溯源树
样例
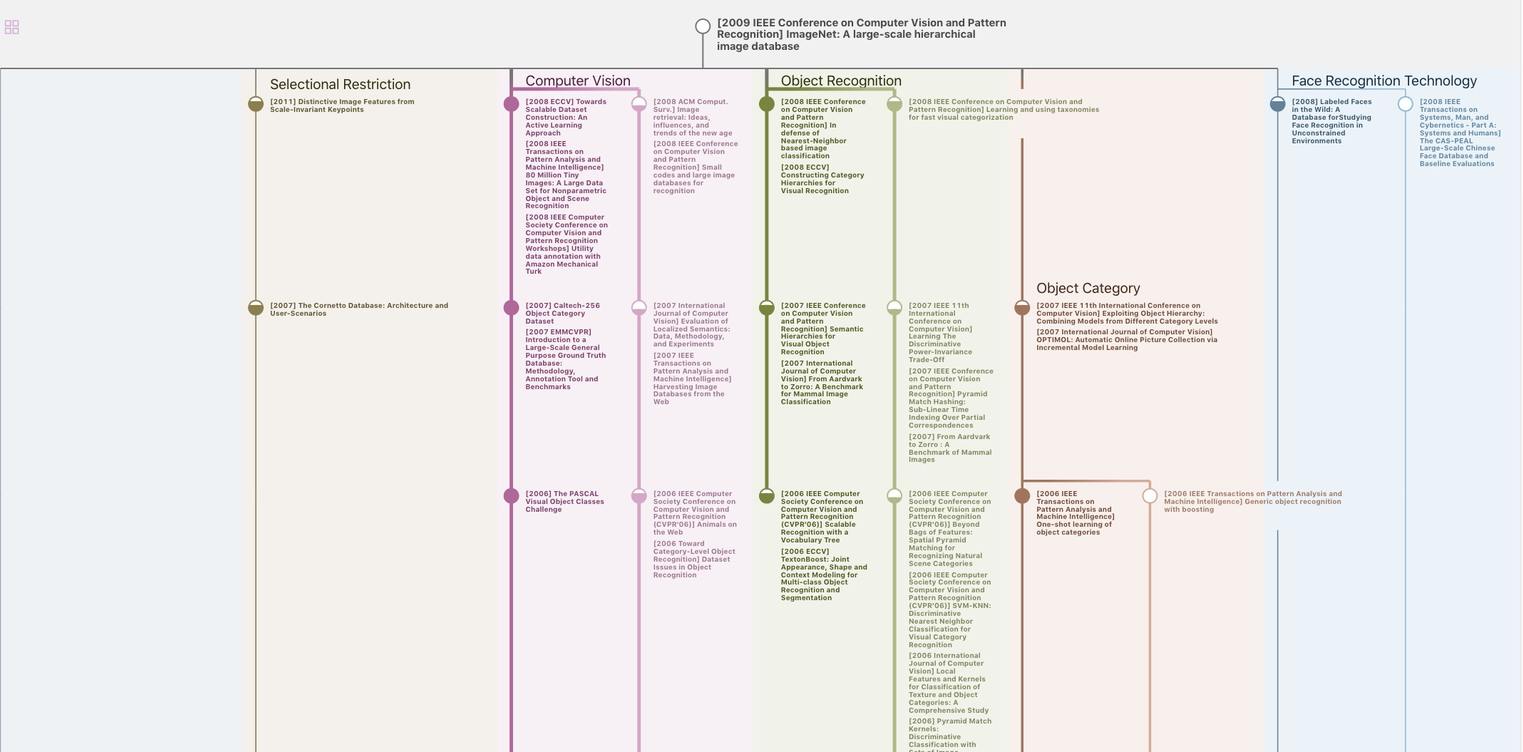
生成溯源树,研究论文发展脉络
Chat Paper
正在生成论文摘要