Leveraging polygenic enrichments of gene features to predict genes underlying complex traits and diseases
user-5f8cf7e04c775ec6fa691c92(2023)
摘要
Genome-wide association studies (GWASs) are a valuable tool for understanding the biology of complex human traits and diseases, but associated variants rarely point directly to causal genes. In the present study, we introduce a new method, polygenic priority score (PoPS), that learns trait-relevant gene features, such as cell-type-specific expression, to prioritize genes at GWAS loci. Using a large evaluation set of genes with fine-mapped coding variants, we show that PoPS and the closest gene individually outperform other gene prioritization methods, but observe the best overall performance by combining PoPS with orthogonal methods. Using this combined approach, we prioritize 10,642 unique gene–trait pairs across 113 complex traits and diseases with high precision, finding not only well-established gene–trait relationships but nominating new genes at unresolved loci, such as LGR4 for estimated glomerular filtration rate and CCR7 for deep vein thrombosis. Overall, we demonstrate that PoPS provides a powerful addition to the gene prioritization toolbox.
更多查看译文
关键词
Genome-wide association study,Gene,Genetic association,Computational biology,Gene expression,Biological pathway,Biology,Gene prioritization,Ldl cholesterol,Novel gene
AI 理解论文
溯源树
样例
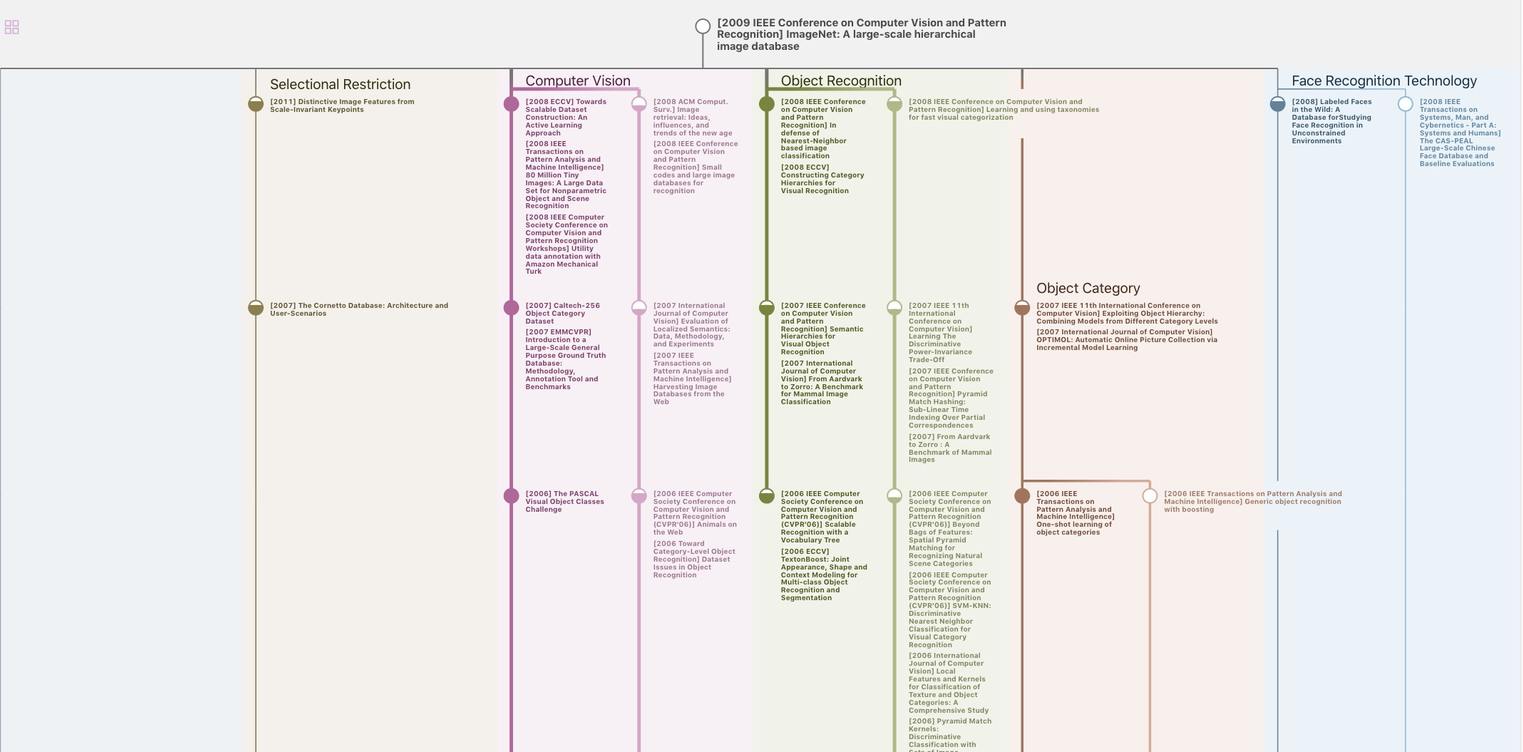
生成溯源树,研究论文发展脉络
Chat Paper
正在生成论文摘要