Learning with self-supervision on EEG data
2021 9th International Winter Conference on Brain-Computer Interface (BCI)(2021)
摘要
Supervised learning paradigms are often limited by the amount of labeled data that is available. This phenomenon is particularly problematic in clinically-relevant data, such as electroencephalography (EEG), where labeling can be costly in terms of specialized expertise and human processing time. Consequently, deep learning architectures designed to learn on EEG data have yielded relatively shallow models and performances at best similar to those of traditional feature-based approaches. However, in most situations, unlabeled data is available in abundance. By extracting information from this unlabeled data, it might be possible to reach competitive performance with deep neural networks despite limited access to labels. Here we report results using self-supervised learning (SSL), a promising technique for discovering structure in unlabeled data, to learn representations of EEG signals. Specifically, we consider a contrastive approach and provide results on two clinically-relevant problems: EEG-based sleep staging and pathology detection. Results report that linear classifiers trained on SSL-learned features consistently outperformed purely supervised deep neural networks in low-labeled data regimes while reaching competitive performance when all labels were available. Our results suggest that self-supervision may pave the way to a wider use of deep learning models on EEG data.
更多查看译文
关键词
Deep learning,Sleep,Supervised learning,Brain modeling,Electroencephalography,Data models,Biological neural networks
AI 理解论文
溯源树
样例
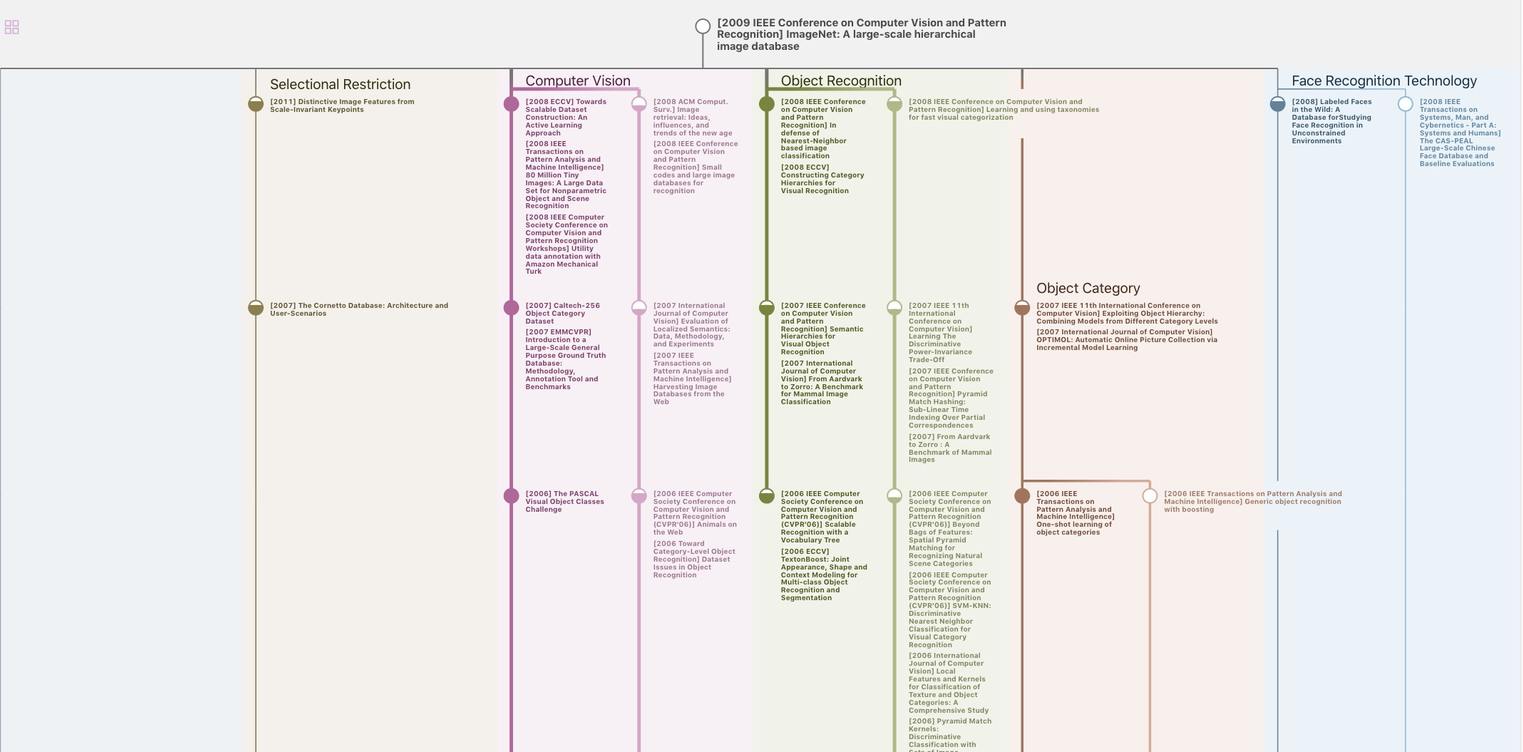
生成溯源树,研究论文发展脉络
Chat Paper
正在生成论文摘要