Modeling Of Peptides With Classical And Novel Machine Learning Force Fields: A Comparison
JOURNAL OF PHYSICAL CHEMISTRY B(2021)
摘要
The replacement of classical force fields (FFs) with novel neural-network-based frameworks is an emergent topic in molecular dynamics (MD) simulations. In contrast to classical FFs, which have proven their capability to provide insights into complex soft matter systems at an atomistic resolution, the machine learning (ML) potentials have yet to demonstrate their applicability for soft materials. However, the underlying philosophy, which is learning the energy of an atom in its surrounding chemical environment, makes this approach a promising tool. In particular for the exploration of novel chemical compounds, which have not been considered in the original parametrization of classical FFs. In this article, we study the performance of the ANI-2x ML model and compare the results with those of two classical FFs, namely, CHARMM27 and the GROMOS96 43a1 FF. We explore the performance of these FFs for bulk water and two model peptides, trialanine and a 9-mer of the a-aminoisobutyric acid, in vacuum and water. The results for water describe a highly ordered water structure, with a structure similar to those using ab initio molecular dynamics simulations. The energy landscape of the peptides described by Ramachandran maps show secondary structure basins similar to those of the classical FFs but differ in the position and relative stability of the basins. Details of the sampled structures show a divergent performance of the different models, which can be related either to the short-ranged nature of the ML potentials or to shortcomings of the underlying data set used for training. These findings highlight the current state of the applicability of ANI-2x ML potential for MD simulations of soft matter systems. Simultaneously, they provide insights for future improvements of current ML potentials.
更多查看译文
AI 理解论文
溯源树
样例
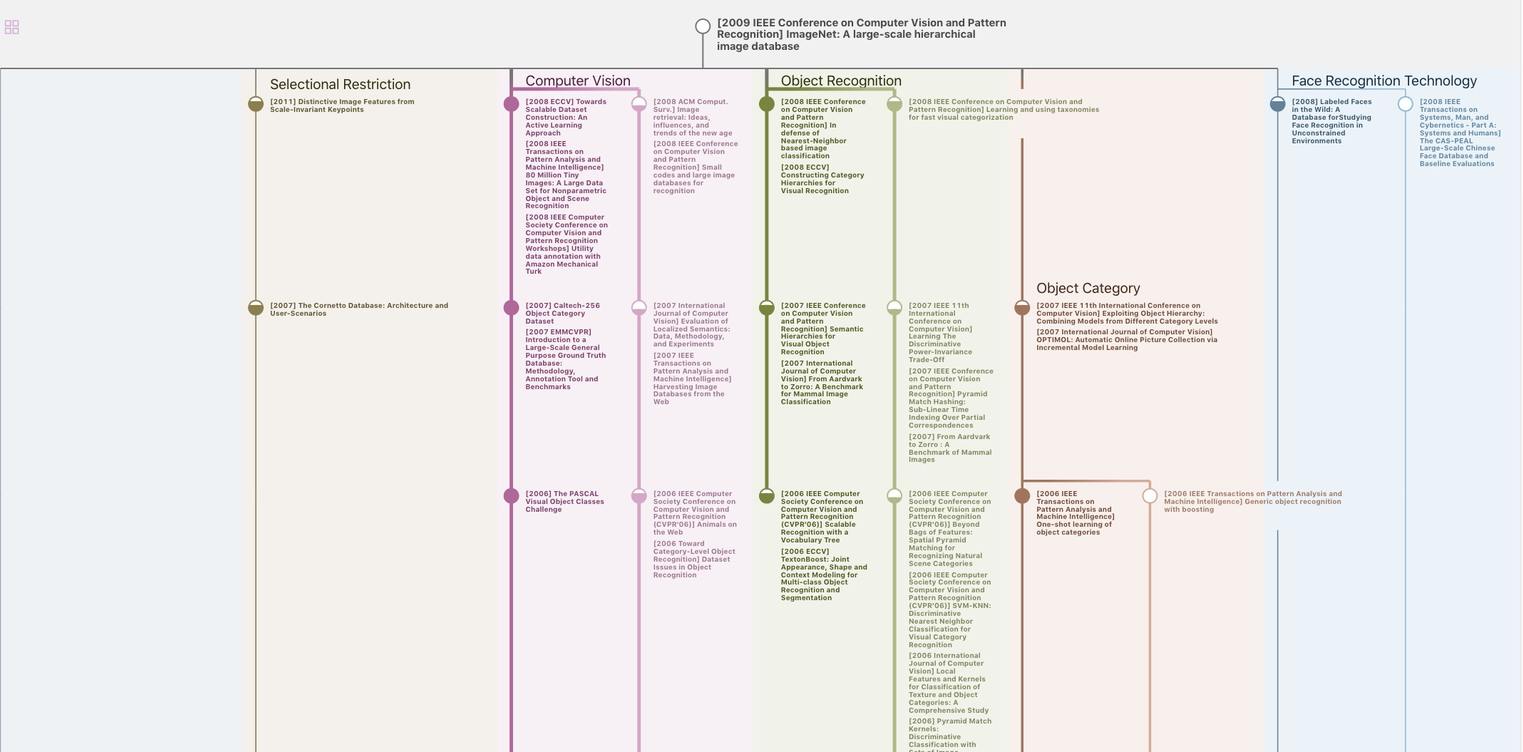
生成溯源树,研究论文发展脉络
Chat Paper
正在生成论文摘要