Convolutional Neural Networks For The Detection Of The Early Inspiral Of A Gravitational-Wave Signal
PHYSICAL REVIEW D(2021)
摘要
GW170817 has led to the first example of multimessenger astronomy with observations from gravitational wave interferometers and electromagnetic telescopes combined to characterize the source. However, detections of the early inspiral phase by the gravitational wave detectors would allow the observation of the earlier stages of the merger in the electromagnetic band, improving multimessenger astronomy and giving access to new information. In this paper, we introduce a new machine-learning-based approach to produce early-warning alerts for an inspiraling binary neutron star system, based only on the early inspiral part of the signal. We give a proof of concept to show the possibility to use a combination of small convolutional neural networks trained on the whitened detector strain in the time domain to detect and classify early inspirals. Each of those is targeting a specific range of chirp masses dividing the binary neutron star category into three subclasses: light, intermediate, and heavy. In this work, we focus on one LIGO detector at design sensitivity and generate noise from the design power spectral density. We show that within this setup it is possible to produce an early alert up to 100 seconds before the merger for the best-case scenario. We also present some future upgrades that will enhance the detection capabilities of our convolutional neural networks. Finally, we also show that the current number of detections for a realistic binary neutron star population is comparable to that of matched filtering and that there is a high probability to detect GW170817- and GW190425-like events at design sensitivity.
更多查看译文
AI 理解论文
溯源树
样例
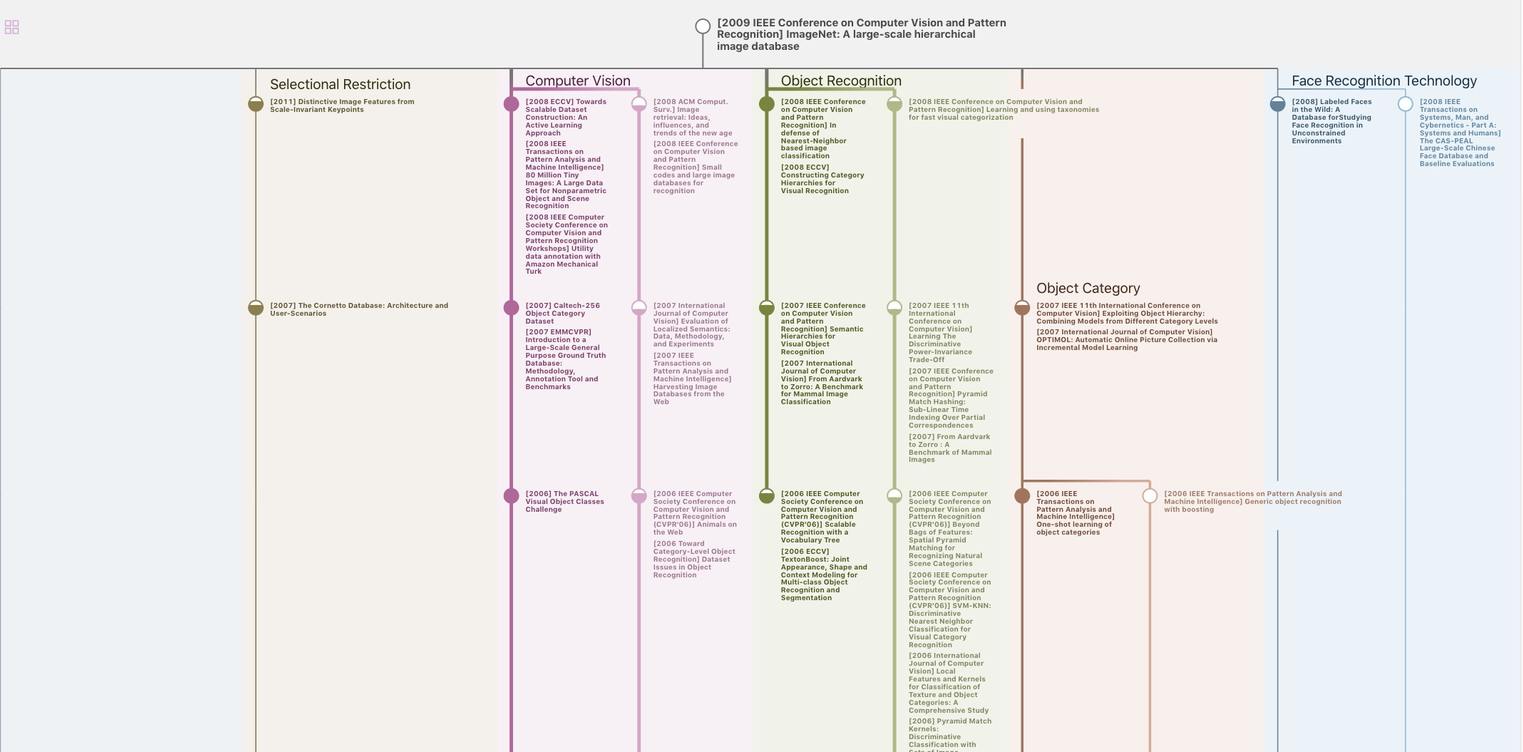
生成溯源树,研究论文发展脉络
Chat Paper
正在生成论文摘要