Camouflaged Instance Segmentation In-the-Wild: Dataset, Method, and Benchmark Suite.
IEEE Transactions on Image Processing(2021)
摘要
This paper pushes the envelope on decomposing camouflaged regions in an image into meaningful components, namely, camouflaged instances. To promote the new task of camouflaged instance segmentation of in-the-wild images, we introduce a dataset, dubbed CAMO++, that extends our preliminary CAMO dataset (camouflaged object segmentation) in terms of quantity and diversity. The new dataset substantially increases the number of images with hierarchical pixel-wise ground truths. We also provide a benchmark suite for the task of camouflaged instance segmentation. In particular, we present an extensive evaluation of state-of-the-art instance segmentation methods on our newly constructed CAMO++ dataset in various scenarios. We also present a camouflage fusion learning (CFL) framework for camouflaged instance segmentation to further improve the performance of state-of-the-art methods. The dataset, model, evaluation suite, and benchmark will be made publicly available on our project page.
更多查看译文
关键词
Camouflaged instance segmentation,in-the-wild image,camouflage dataset,benchmark suite,multimodal learning
AI 理解论文
溯源树
样例
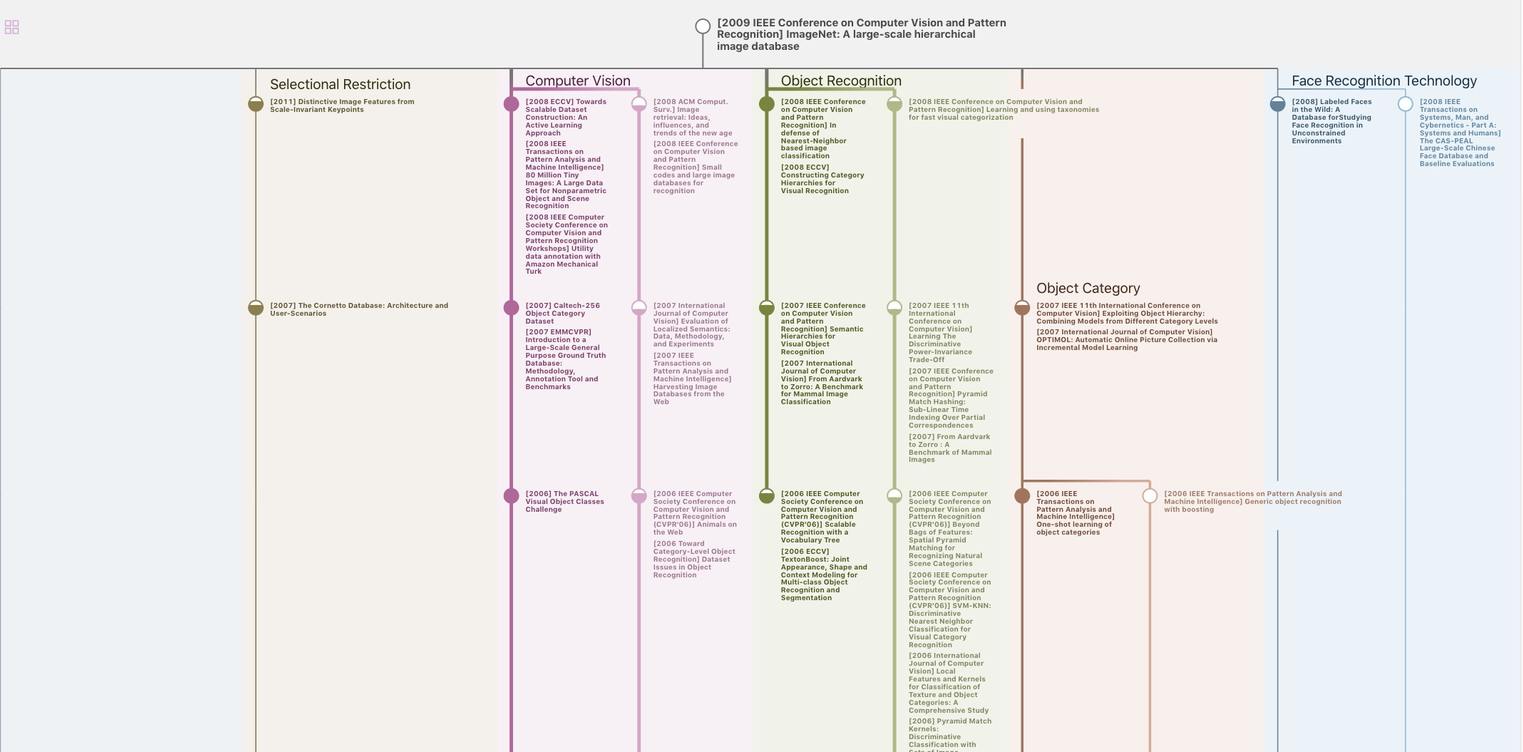
生成溯源树,研究论文发展脉络
Chat Paper
正在生成论文摘要