Learning And Exploring Motor Skills With Spacetime Bounds
COMPUTER GRAPHICS FORUM(2021)
摘要
Equipping characters with diverse motor skills is the current bottleneck of physics-based character animation. We propose a Deep Reinforcement Learning (DRL) framework that enables physics-based characters to learn and explore motor skills from reference motions. The key insight is to use loose space-time constraints, termed spacetime bounds, to limit the search space in an early termination fashion. As we only rely on the reference to specify loose spacetime bounds, our learning is more robust with respect to low quality references. Moreover, spacetime bounds are hard constraints that improve learning of challenging motion segments, which can be ignored by imitation-only learning. We compare our method with state-of-the-art tracking-based DRL methods. We also show how to guide style exploration within the proposed framework.
更多查看译文
关键词
<bold>CCS Concepts</bold>, center dot <bold>Computing methodologies -> Animation, </bold>, Physical simulation, center dot <bold>Theory of computation</bold> -> Reinforcement learning
AI 理解论文
溯源树
样例
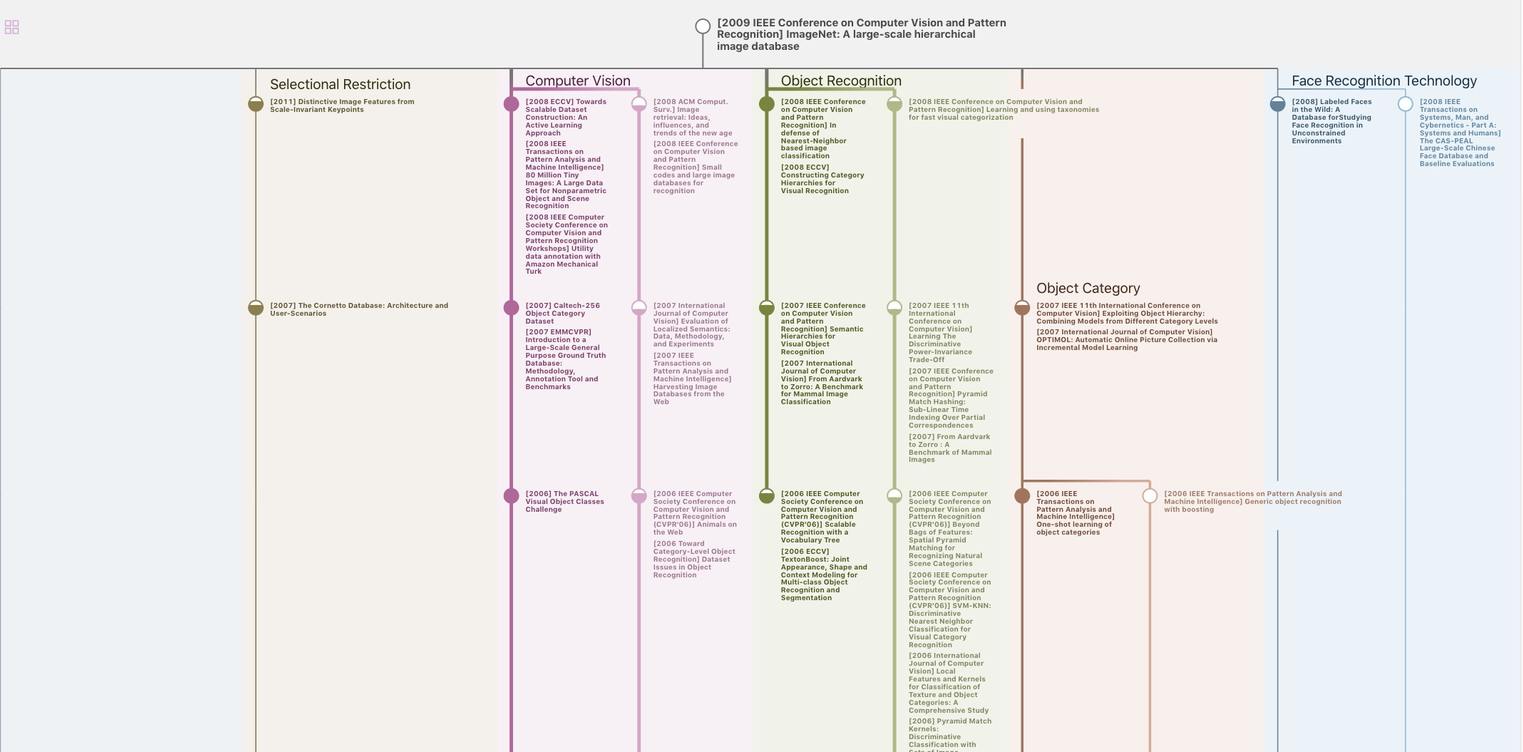
生成溯源树,研究论文发展脉络
Chat Paper
正在生成论文摘要