A study of latent monotonic attention variants
arxiv(2021)
摘要
End-to-end models reach state-of-the-art performance for speech recognition, but global soft attention is not monotonic, which might lead to convergence problems, to instability, to bad generalisation, cannot be used for online streaming, and is also inefficient in calculation. Monotonicity can potentially fix all of this. There are several ad-hoc solutions or heuristics to introduce monotonicity, but a principled introduction is rarely found in literature so far. In this paper, we present a mathematically clean solution to introduce monotonicity, by introducing a new latent variable which represents the audio position or segment boundaries. We compare several monotonic latent models to our global soft attention baseline such as a hard attention model, a local windowed soft attention model, and a segmental soft attention model. We can show that our monotonic models perform as good as the global soft attention model. We perform our experiments on Switchboard 300h. We carefully outline the details of our training and release our code and configs.
更多查看译文
关键词
latent monotonic attention variants
AI 理解论文
溯源树
样例
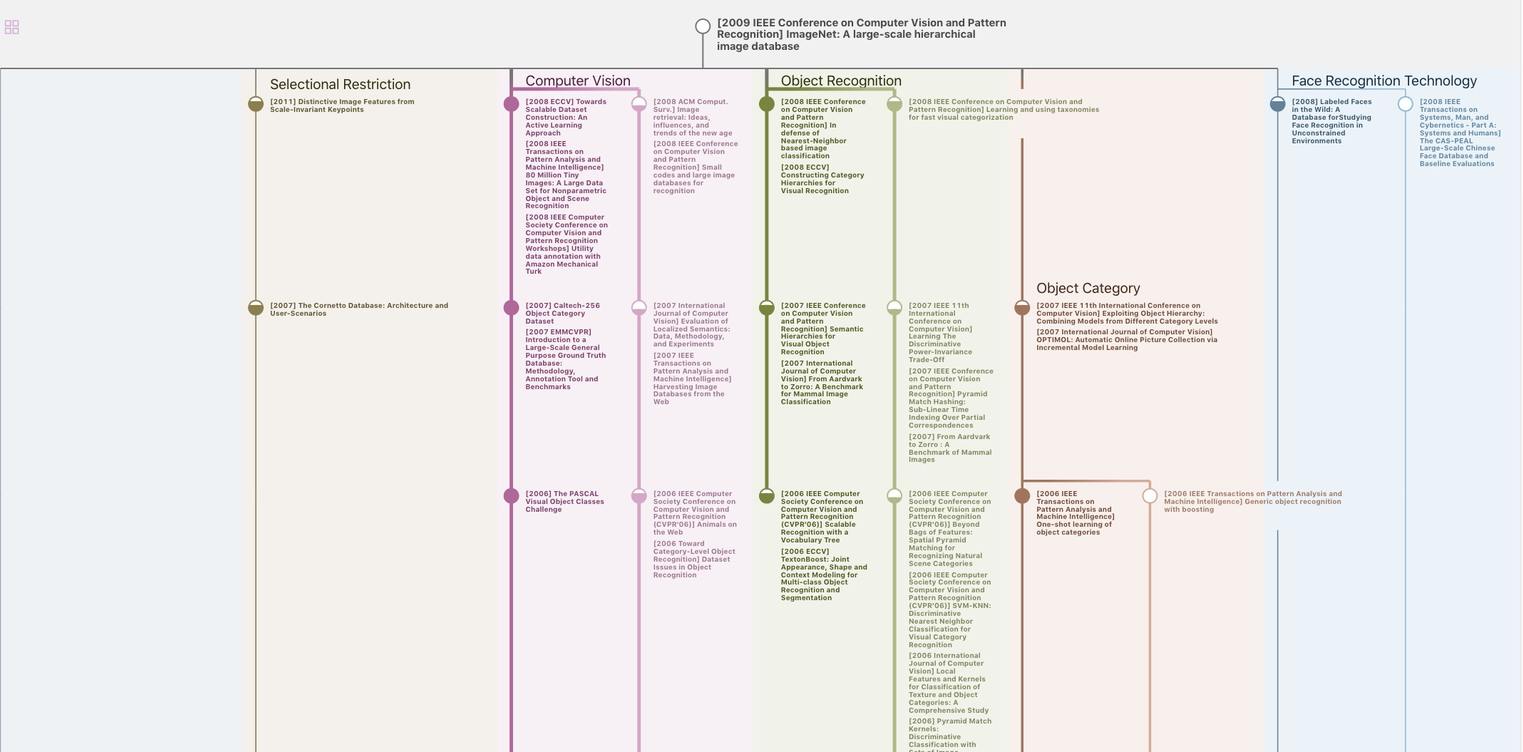
生成溯源树,研究论文发展脉络
Chat Paper
正在生成论文摘要