Deep Hedging of Derivatives Using Reinforcement Learning
arxiv(2021)
摘要
This paper shows how reinforcement learning can be used to derive optimal hedging strategies for derivatives when there are transaction costs. The paper illustrates the approach by showing the difference between using delta hedging and optimal hedging for a short position in a call option when the objective is to minimize a function equal to the mean hedging cost plus a constant times the standard deviation of the hedging cost. Two situations are considered. In the first, the asset price follows a geometric Brownian motion. In the second, the asset price follows a stochastic volatility process. The paper extends the basic reinforcement learning approach in a number of ways. First, it uses two different Q-functions so that both the expected value of the cost and the expected value of the square of the cost are tracked for different state/action combinations. This approach increases the range of objective functions that can be used. Second, it uses a learning algorithm that allows for continuous state and action space. Third, it compares the accounting P&L approach (where the hedged position is valued at each step) and the cash flow approach (where cash inflows and outflows are used). We find that a hybrid approach involving the use of an accounting P&L approach that incorporates a relatively simple valuation model works well. The valuation model does not have to correspond to the process assumed for the underlying asset price.
更多查看译文
关键词
reinforcement learning,derivatives
AI 理解论文
溯源树
样例
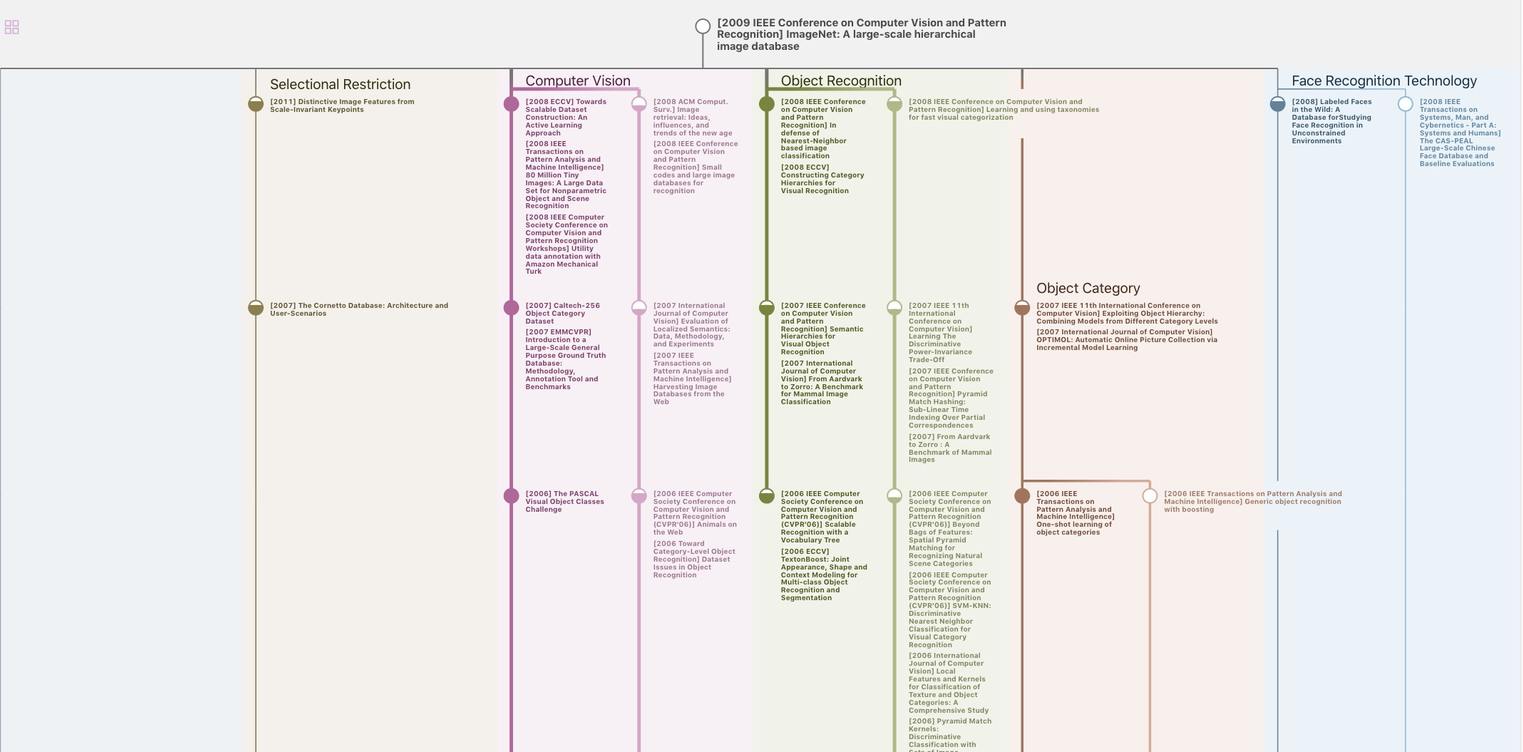
生成溯源树,研究论文发展脉络
Chat Paper
正在生成论文摘要