Graph Stacked Hourglass Networks for 3D Human Pose Estimation
2021 IEEE/CVF CONFERENCE ON COMPUTER VISION AND PATTERN RECOGNITION, CVPR 2021(2021)
摘要
In this paper, we propose a novel graph convolutional network architecture, Graph Stacked Hourglass Networks, for 2D-to-3D human pose estimation tasks. The proposed architecture consists of repeated encoder-decoder, in which graph-structured features are processed across three different scales of human skeletal representations. This multi-scale architecture enables the model to learn both local and global feature representations, which are critical for 3D human pose estimation. We also introduce a multi-level feature learning approach using different-depth intermediate features and show the performance improvements that result from exploiting multi-scale, multi-level feature representations. Extensive experiments are conducted to validate our approach, and the results show that our model outperforms the state-of-the-art.
更多查看译文
关键词
repeated encoder-decoder,graph-structured features,human skeletal representations,multiscale architecture,local feature representations,global feature representations,3D human pose estimation,different-depth intermediate features,multilevel feature representations,graph convolutional network architecture,graph stacked hourglass networks
AI 理解论文
溯源树
样例
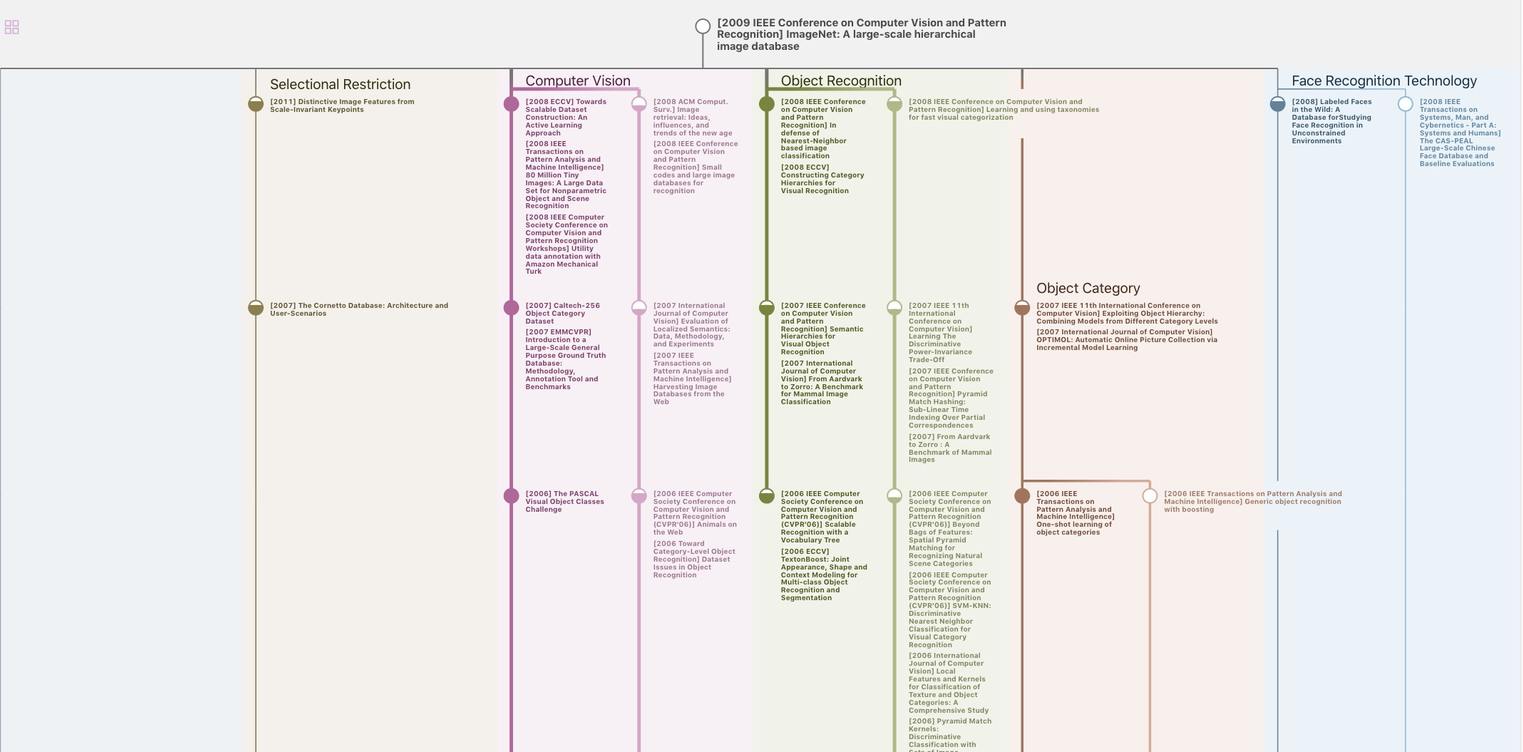
生成溯源树,研究论文发展脉络
Chat Paper
正在生成论文摘要