TransFill: Reference-guided Image Inpainting by Merging Multiple Color and Spatial Transformations
2021 IEEE/CVF CONFERENCE ON COMPUTER VISION AND PATTERN RECOGNITION, CVPR 2021(2021)
摘要
Image inpainting is the task of plausibly restoring missing pixels within a hole region that is to be removed from a target image. Most existing technologies exploit patch similarities within the image, or leverage large-scale training data to fill the hole using learned semantic and texture information. However, due to the ill-posed nature of the inpainting task, such methods struggle to complete larger holes containing complicated scenes. In this paper, we propose TransFill, a multi-homography transformed fusion method to fill the hole by referring to another source image that shares scene contents with the target image. We first align the source image to the target image by estimating multiple homographies guided by different depth levels. We then learn to adjust the color and apply a pixel-level warping to each homography-warped source image to make it more consistent with the target. Finally, a pixel-level fusion module is learned to selectively merge the different proposals. Our method achieves state-of-the-art performance on pairs of images across a variety of wide baselines and color differences, and generalizes to user-provided image pairs.
更多查看译文
关键词
TransFill,reference-guided image inpainting,merging multiple color,leverage large-scale training data,learned semantic texture information,multihomography transformed fusion method,multiple homographies,pixel-level warping,homography-warped source image,pixel-level fusion module,user-provided image pairs
AI 理解论文
溯源树
样例
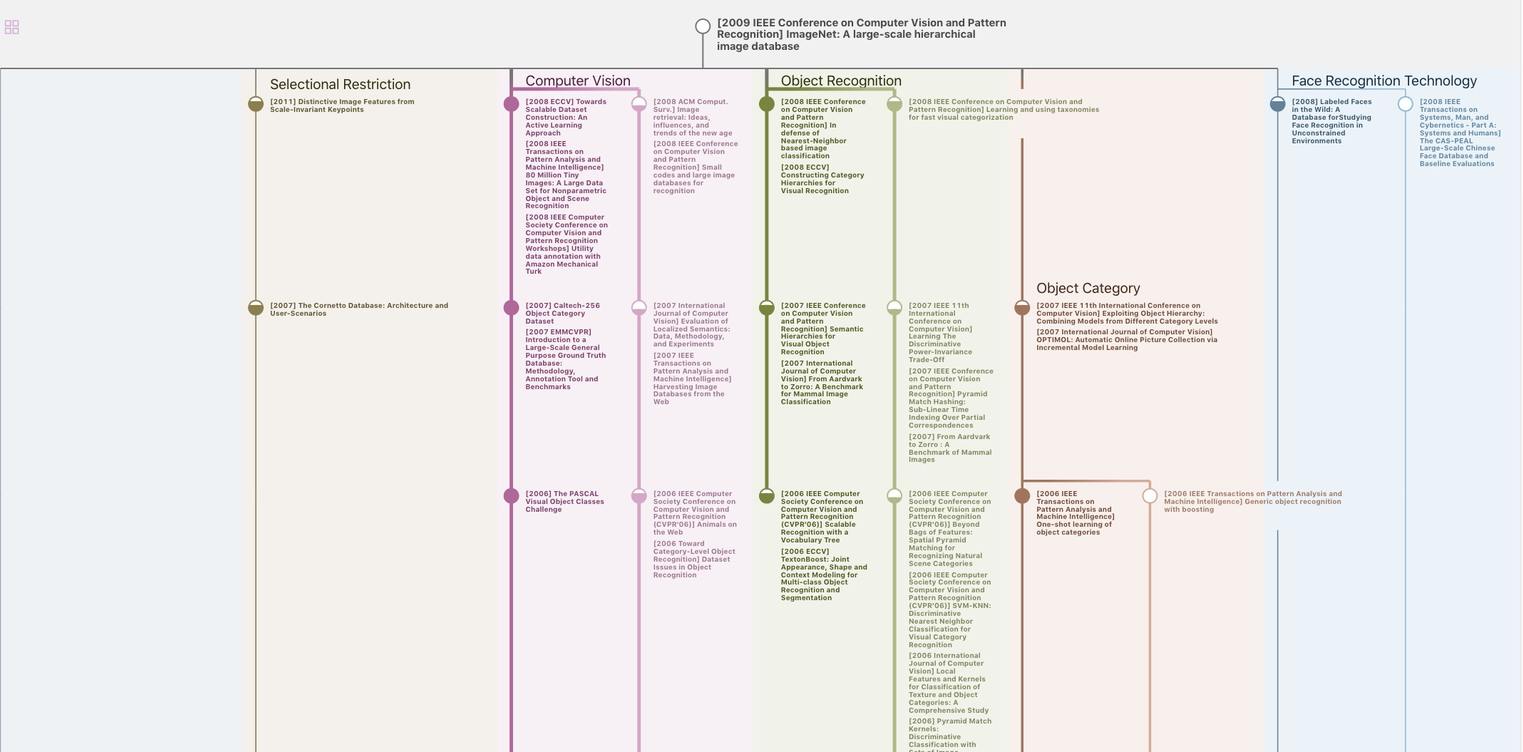
生成溯源树,研究论文发展脉络
Chat Paper
正在生成论文摘要