Debiasing Career Recommendations with Neural Fair Collaborative Filtering
International World Wide Web Conference(2021)
摘要
A growing proportion of human interactions are digitized on social media platforms and subjected to algorithmic decision-making, and it has become increasingly important to ensure fair treatment from these algorithms. In this work, we investigate gender bias in collaborative-filtering recommender systems trained on social media data. We develop neural fair collaborative filtering (NFCF), a practical framework for mitigating gender bias in recommending career-related sensitive items (e.g. jobs, academic concentrations, or courses of study) using a pre-training and fine-tuning approach to neural collaborative filtering, augmented with bias correction techniques. We show the utility of our methods for gender de-biased career and college major recommendations on the MovieLens dataset and a Facebook dataset, respectively, and achieve better performance and fairer behavior than several state-of-the-art models.
更多查看译文
关键词
Fairness in AI, AI & society, ethical issues, social media, recommender systems, collaborative filtering, career recommendation
AI 理解论文
溯源树
样例
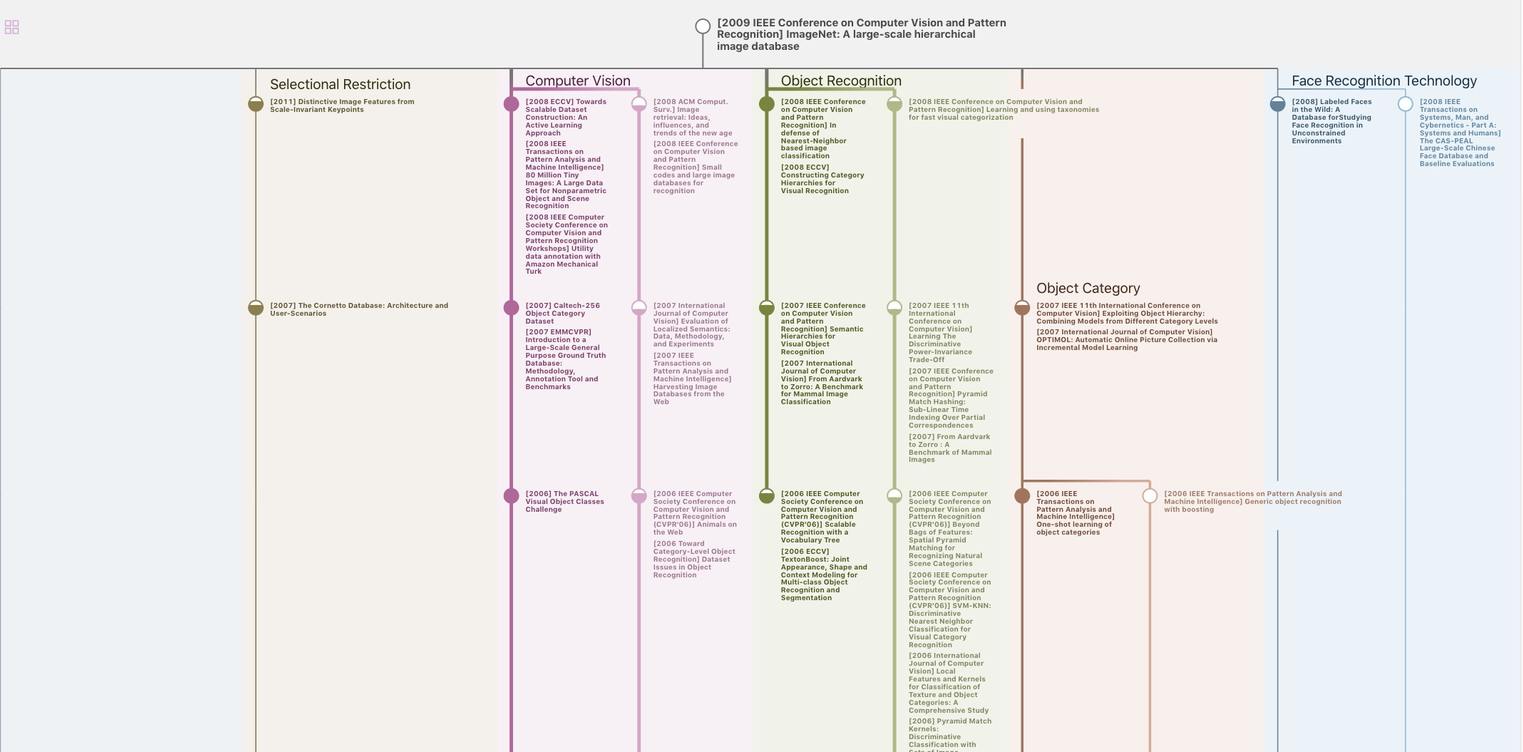
生成溯源树,研究论文发展脉络
Chat Paper
正在生成论文摘要