What do You Mean? Interpreting Image Classification with Crowdsourced Concept Extraction and Analysis
International World Wide Web Conference(2021)
摘要
ABSTRACT Global interpretability is a vital requirement for image classification applications. Existing interpretability methods mainly explain a model behavior by identifying salient image patches, which require manual efforts from users to make sense of, and also do not typically support model validation with questions that investigate multiple visual concepts. In this paper, we introduce a scalable human-in-the-loop approach for global interpretability. Salient image areas identified by local interpretability methods are annotated with semantic concepts, which are then aggregated into a tabular representation of images to facilitate automatic statistical analysis of model behavior. We show that this approach answers interpretability needs for both model validation and exploration, and provides semantically more diverse, informative, and relevant explanations while still allowing for scalable and cost-efficient execution.
更多查看译文
关键词
machine-learning interpretability, image classification, human computation, concept extraction
AI 理解论文
溯源树
样例
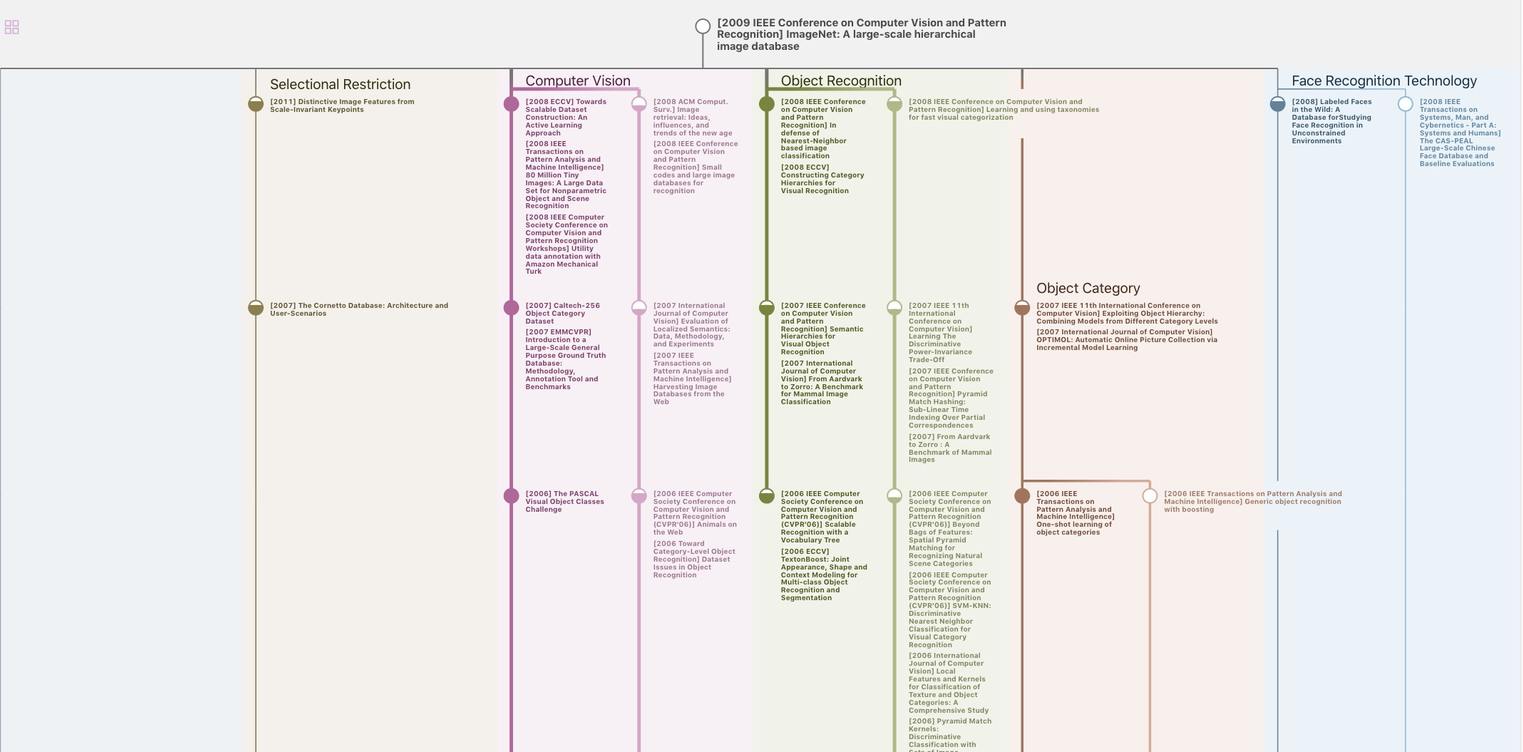
生成溯源树,研究论文发展脉络
Chat Paper
正在生成论文摘要