RobustNet: Improving Domain Generalization in Urban-Scene Segmentation via Instance Selective Whitening
2021 IEEE/CVF CONFERENCE ON COMPUTER VISION AND PATTERN RECOGNITION, CVPR 2021(2021)
摘要
Enhancing the generalization capability of deep neural networks to unseen domains is crucial for safety-critical applications in the real world such as autonomous driving. To address this issue, this paper proposes a novel instance selective whitening loss to improve the robustness of the segmentation networks for unseen domains. Our approach disentangles the domain-specific style and domain-invariant content encoded in higher-order statistics (i.e., feature covariance) of the feature representations and selectively removes only the style information causing domain shift. As shown in Fig. 1, our method provides reasonable predictions for (a) low-illuminated, (b) rainy, and (c) unseen structures. These types of images are not included in the training dataset, where the baseline shows a significant performance drop, contrary to ours. Being simple yet effective, our approach improves the robustness of various backbone networks without additional computational cost. We conduct extensive experiments in urban-scene segmentation and show the superiority of our approach to existing work. Our code is available at this link(1).
更多查看译文
关键词
domain generalization,urban-scene segmentation,generalization capability,deep neural networks,unseen domains,safety-critical applications,autonomous driving,segmentation networks,domain-specific style,domain-invariant content,higher-order statistics,feature covariance,feature representations,style information,domain shift,unseen structures,backbone networks,instance selective whitening loss,feature representation
AI 理解论文
溯源树
样例
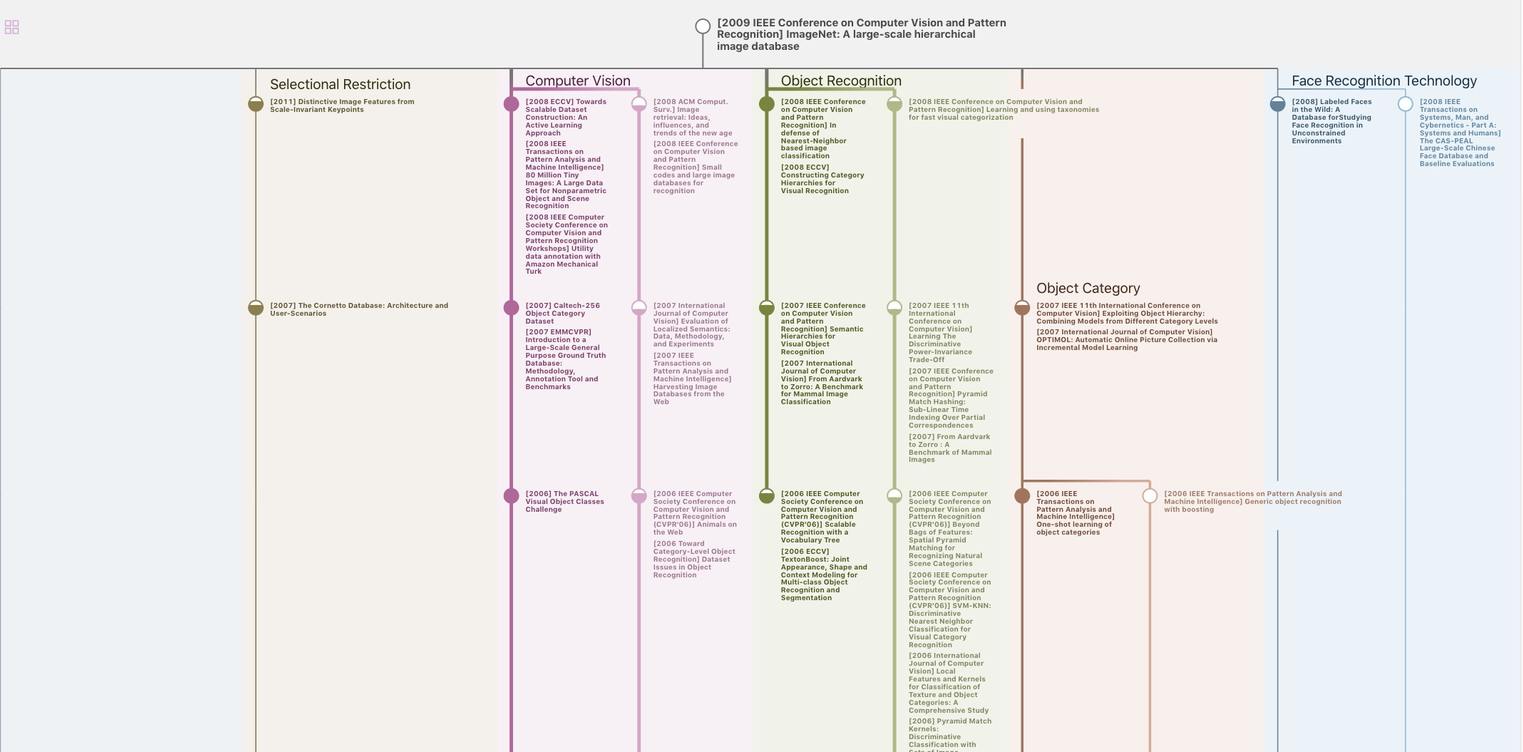
生成溯源树,研究论文发展脉络
Chat Paper
正在生成论文摘要