Anomaly Detection Under Multiplicative Noise Model Uncertainty
IEEE CONTROL SYSTEMS LETTERS(2022)
摘要
State estimators are crucial components of anomaly detectors that are used to monitor cyber-physical systems. Many frequently-used state estimators are susceptible to model risk as they rely critically on the availability of an accurate state-space model. Modeling errors make it more difficult to distinguish whether deviations from expected behavior are due to anomalies or simply a lack of knowledge about the system dynamics. In this letter, we account for model uncertainty through a multiplicative noise framework. Specifically, we propose to use the multiplicative noise LQG based compensator in this setting to hedge against the model uncertainty risk. The size of the residual from the estimator can then be compared against a threshold to detect anomalies. Finally, the proposed detector is validated using numerical simulations. Extension of state-of-the-art anomaly detection in cyber-physical systems to handle model uncertainty represents the main novel contribution of the present work.
更多查看译文
关键词
Uncertainty, Detectors, Computational modeling, Additive noise, Covariance matrices, Stochastic processes, Kalman filters, Anomaly detection, multiplicative noise, coupled riccatti, LQG, vulnerable CPS
AI 理解论文
溯源树
样例
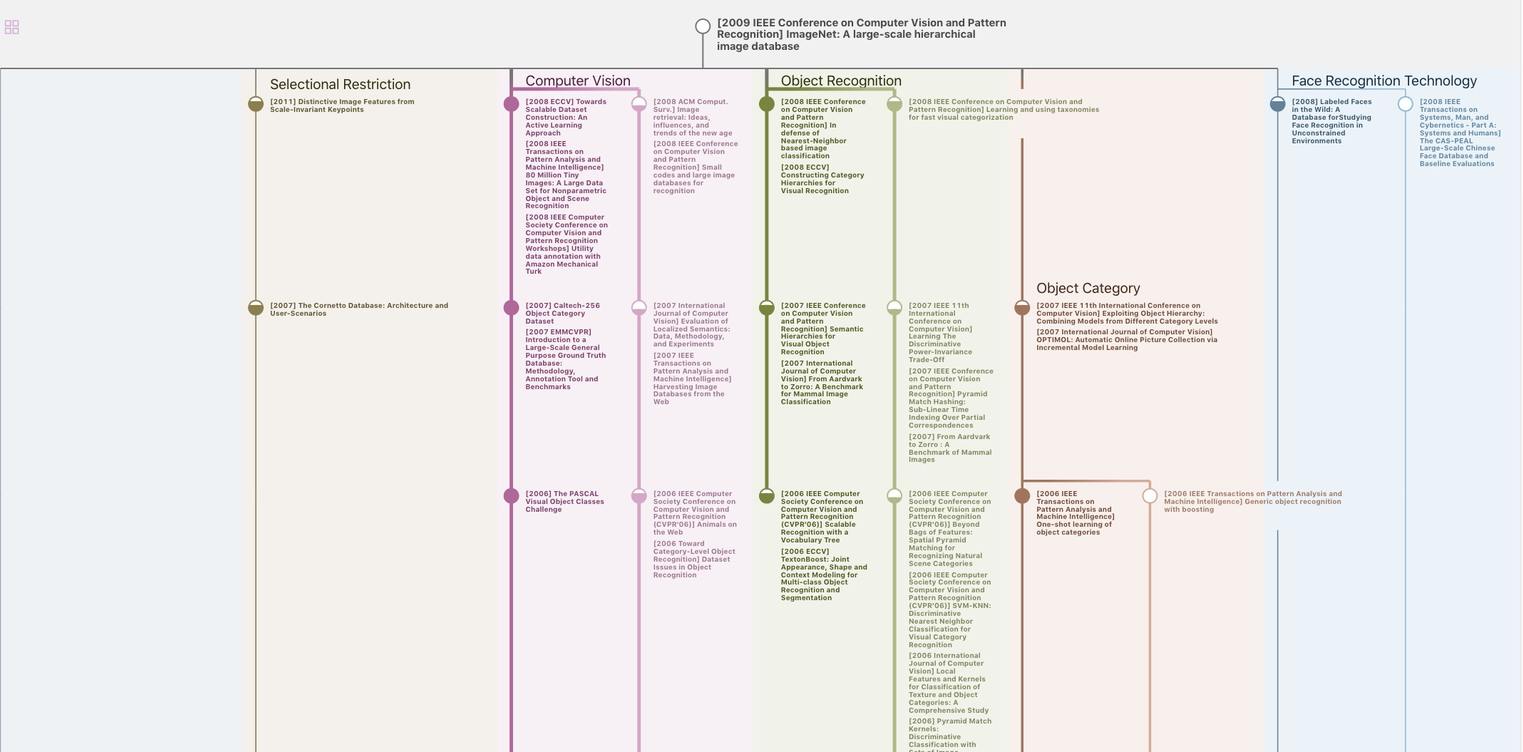
生成溯源树,研究论文发展脉络
Chat Paper
正在生成论文摘要