Continuous Conditional Generative Adversarial Networks (cGAN) with Generator Regularization
arxiv(2021)
摘要
Conditional Generative Adversarial Networks are known to be difficult to train, especially when the conditions are continuous and high-dimensional. To partially alleviate this difficulty, we propose a simple generator regularization term on the GAN generator loss in the form of Lipschitz penalty. Thus, when the generator is fed with neighboring conditions in the continuous space, the regularization term will leverage the neighbor information and push the generator to generate samples that have similar conditional distributions for each neighboring condition. We analyze the effect of the proposed regularization term and demonstrate its robust performance on a range of synthetic and real-world tasks.
更多查看译文
关键词
cgan,generator regularization,networks
AI 理解论文
溯源树
样例
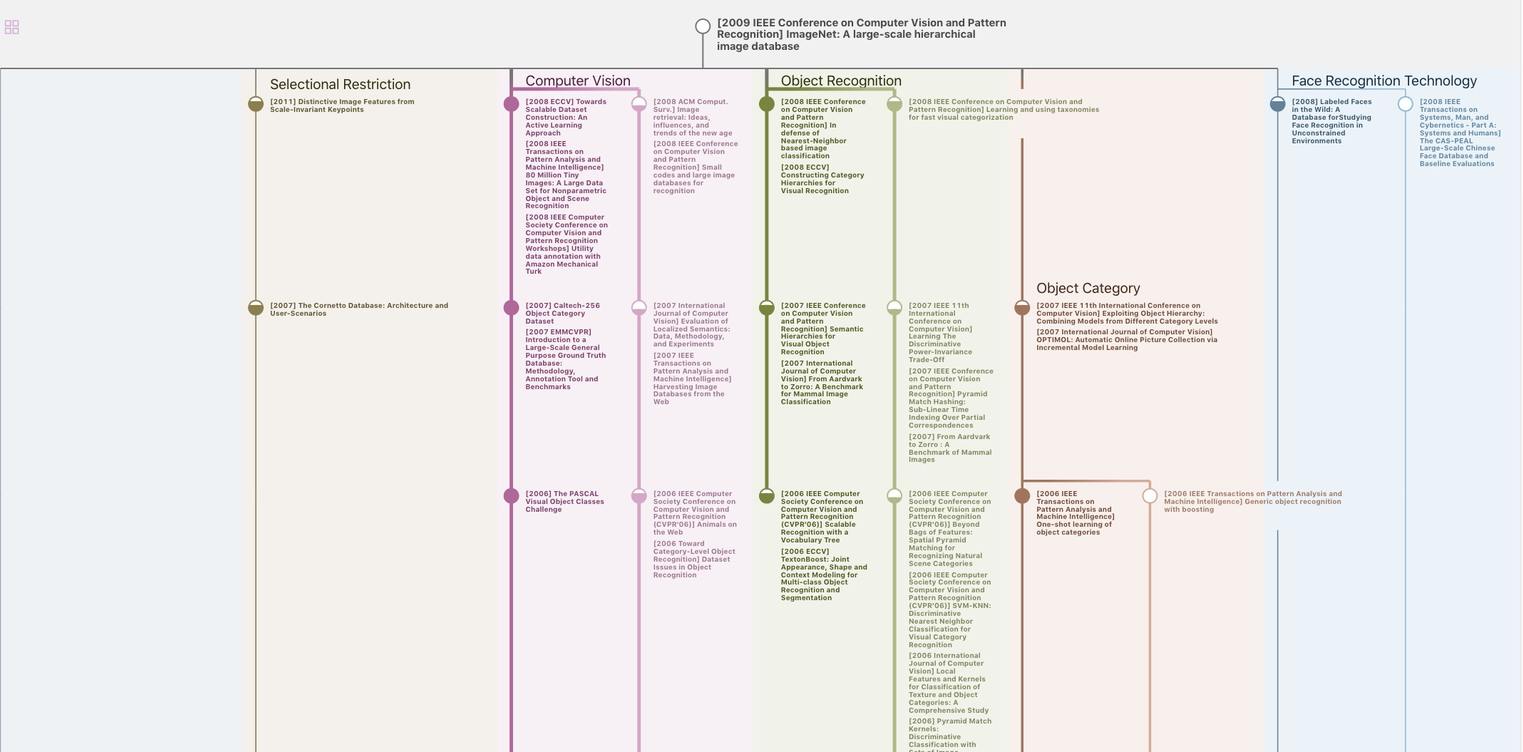
生成溯源树,研究论文发展脉络
Chat Paper
正在生成论文摘要