Cyclic Defense GAN Against Speech Adversarial Attacks
IEEE SIGNAL PROCESSING LETTERS(2021)
摘要
This letter proposes a new defense approach for counteracting state-of-the-art white and black-box adversarial attack algorithms. Our approach fits into the implicit reactive defense algorithm category since it does not directly manipulate the potentially malicious input signals. Instead, it reconstructs a similar signal with a synthesized spectrogram using a cyclic generative adversarial network. This cyclic framework helps to yield a stable generative model. Finally, we feed the reconstructed signal into the speech-to-text model for transcription. The conducted experiments on targeted and non-targeted adversarial attacks developed for attacking DeepSpeech, Kaldi, and Lingvo models demonstrate the proposed defense's effectiveness in adverse scenarios.
更多查看译文
关键词
Spectrogram, Discrete wavelet transforms, Generative adversarial networks, Generators, Signal processing algorithms, Training, Perturbation methods, Speech adversarial attack, Speech-to-text model, discrete wavelet transform, cyclic GAN, adversarial defense
AI 理解论文
溯源树
样例
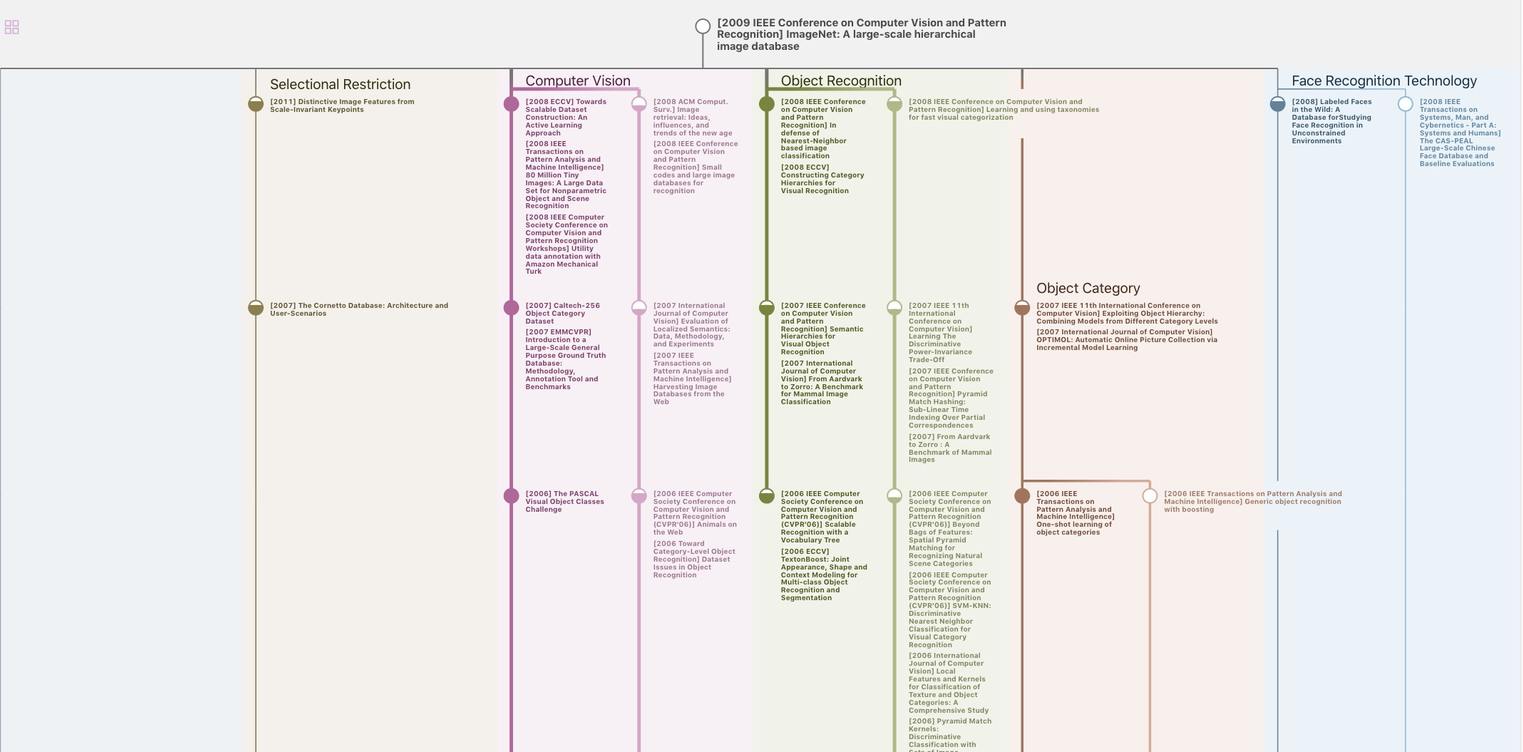
生成溯源树,研究论文发展脉络
Chat Paper
正在生成论文摘要