Using a deep convolutional network to predict the longitudinal dispersion coefficient
Journal of Contaminant Hydrology(2021)
摘要
Given the interest in accurately predicting the Longitudinal Dispersion Coefficient (Dx) within the fields of hydraulic and water quality modeling, a wide range of methods have been used to estimate this parameter. In order to improve the accuracy of Dx predictions, this paper proposes the use of a Deep Convolutional Network (DCN), a sub-field of machine learning. The proposed deep neural network architecture consists of two parts; first, a one-dimensional convolutional neural network (CNN) to build informative feature maps, and second, a stack of deep, fully connected layers to estimate pollution dispersion (as Dx) in streams. To accurately predict Dx the developed model draws upon a large and diverse array of datasets in the form of three dimensionless parameters: Width/Depth (W/H), Velocity/Shear Velocity (U/u*), and Longitudinal Dispersion Coefficient/(Depth * Shear Velocity)
更多查看译文
关键词
Longitudinal dispersion coefficient,Machine learning,Deep convolutional network,Water quality modeling,Uncertainty analysis,Five-fold cross-validation
AI 理解论文
溯源树
样例
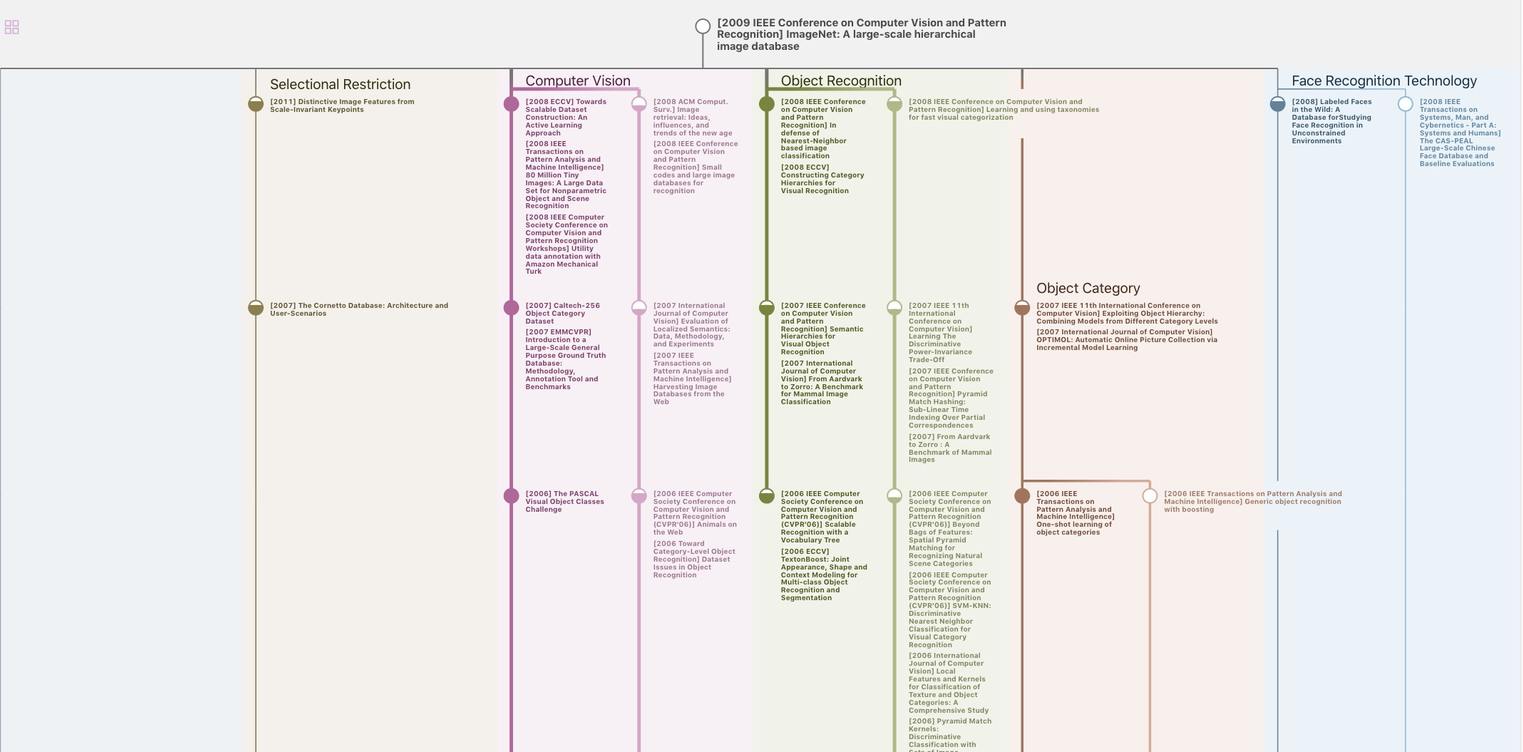
生成溯源树,研究论文发展脉络
Chat Paper
正在生成论文摘要