Improved Sampling And Feature Selection To Support Extreme Gradient Boosting For Pcos Diagnosis
2021 IEEE 11TH ANNUAL COMPUTING AND COMMUNICATION WORKSHOP AND CONFERENCE (CCWC)(2021)
摘要
PolyCystic Ovary Syndrome (PCOS) is one of the most common causes of female infertility, affecting a large number of women of reproductive age, even continuing far beyond the childbearing years. This hormonal disorder may further lead to the risk of other long-term complications. Considering the powerful recognition abilities of the probabilistic nature of ensemble-based gradient boosting algorithms, particularly in the field of the medical domain, we propose the use of Extreme Gradient Boosting, XGBoost, for early detection of PCOS. To strongly support an effective classification performance, we have resampled our data using a combination of SMOTE(Synthetic Minority Oversampling Techniques) & ENN (Edited Nearest Neighbour), to solve class imbalance and data outliers issues. Also, by exploiting popular statistical correlation methods, ANOVA Test Chi-Square Test, we have identified 23 most significant metabolic and clinical parameters that best classify PCOS conditions. Finally, we experimented with our model on a benchmark dataset collected from Kaggle to justify the effectiveness of our proposed findings where the Extreme Gradient Boosting classifier outperformed all other classifiers with a 10 Fold Cross-validation score of 96.03 % all over, along with a 98% Recall in the detection of patients not having PCOS, which outperforms all the existing recent methods where the numerical data-driven diagnosis of PCOS have been studied on this particular dataset.
更多查看译文
关键词
PCOS, Probabilitic Approach, XGBoost, Sampling
AI 理解论文
溯源树
样例
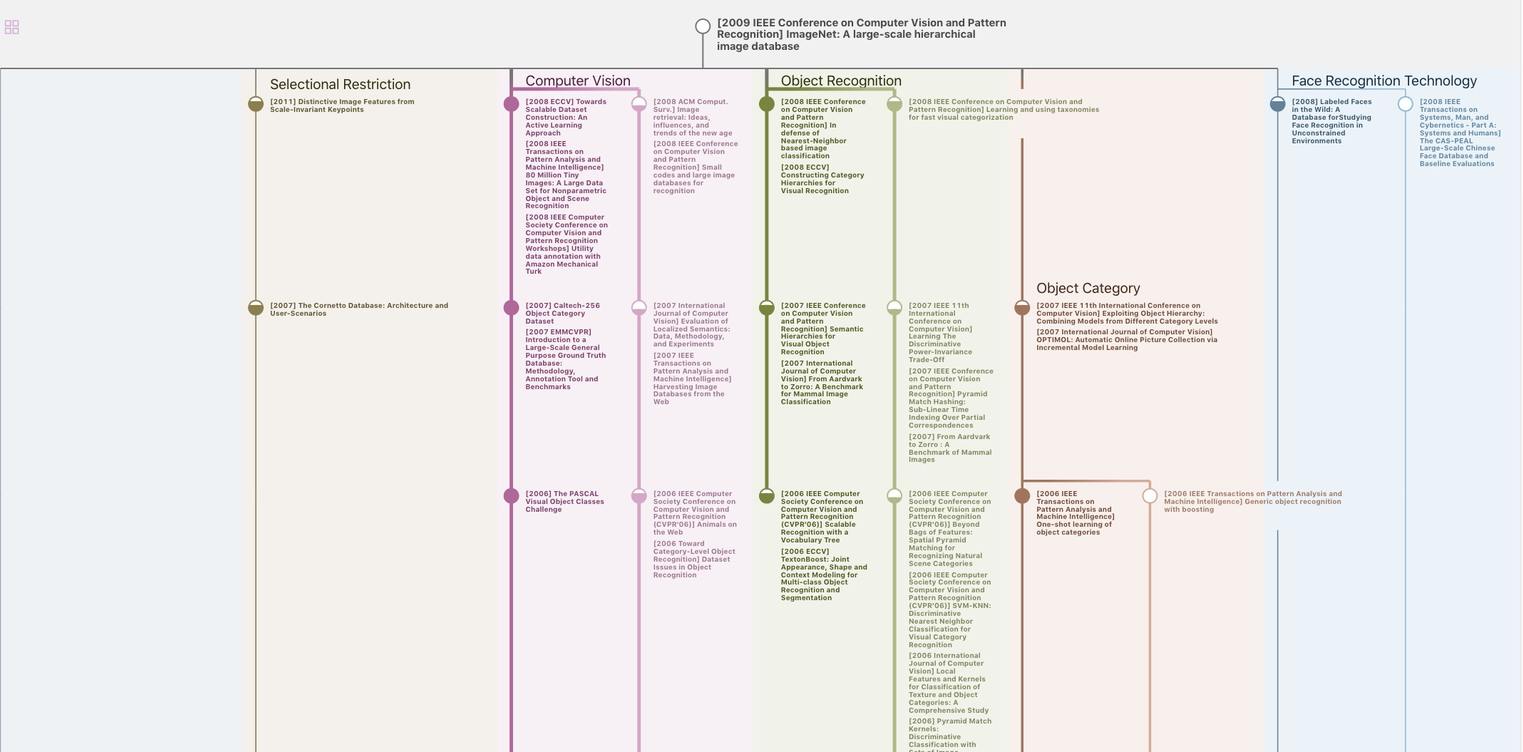
生成溯源树,研究论文发展脉络
Chat Paper
正在生成论文摘要