3D Reasoning for Unsupervised Anomaly Detection in Pediatric WbMRI
arxiv(2021)
摘要
Modern deep unsupervised learning methods have shown great promise for detecting diseases across a variety of medical imaging modalities. While previous generative modeling approaches successfully perform anomaly detection by learning the distribution of healthy 2D image slices, they process such slices independently and ignore the fact that they are correlated, all being sampled from a 3D volume. We show that incorporating the 3D context and processing whole-body MRI volumes is beneficial to distinguishing anomalies from their benign counterparts. In our work, we introduce a multi-channel sliding window generative model to perform lesion detection in whole-body MRI (wbMRI). Our experiments demonstrate that our proposed method significantly outperforms processing individual images in isolation and our ablations clearly show the importance of 3D reasoning. Moreover, our work also shows that it is beneficial to include additional patient-specific features to further improve anomaly detection in pediatric scans.
更多查看译文
关键词
3d reasoning,unsupervised anomaly detection,pediatric
AI 理解论文
溯源树
样例
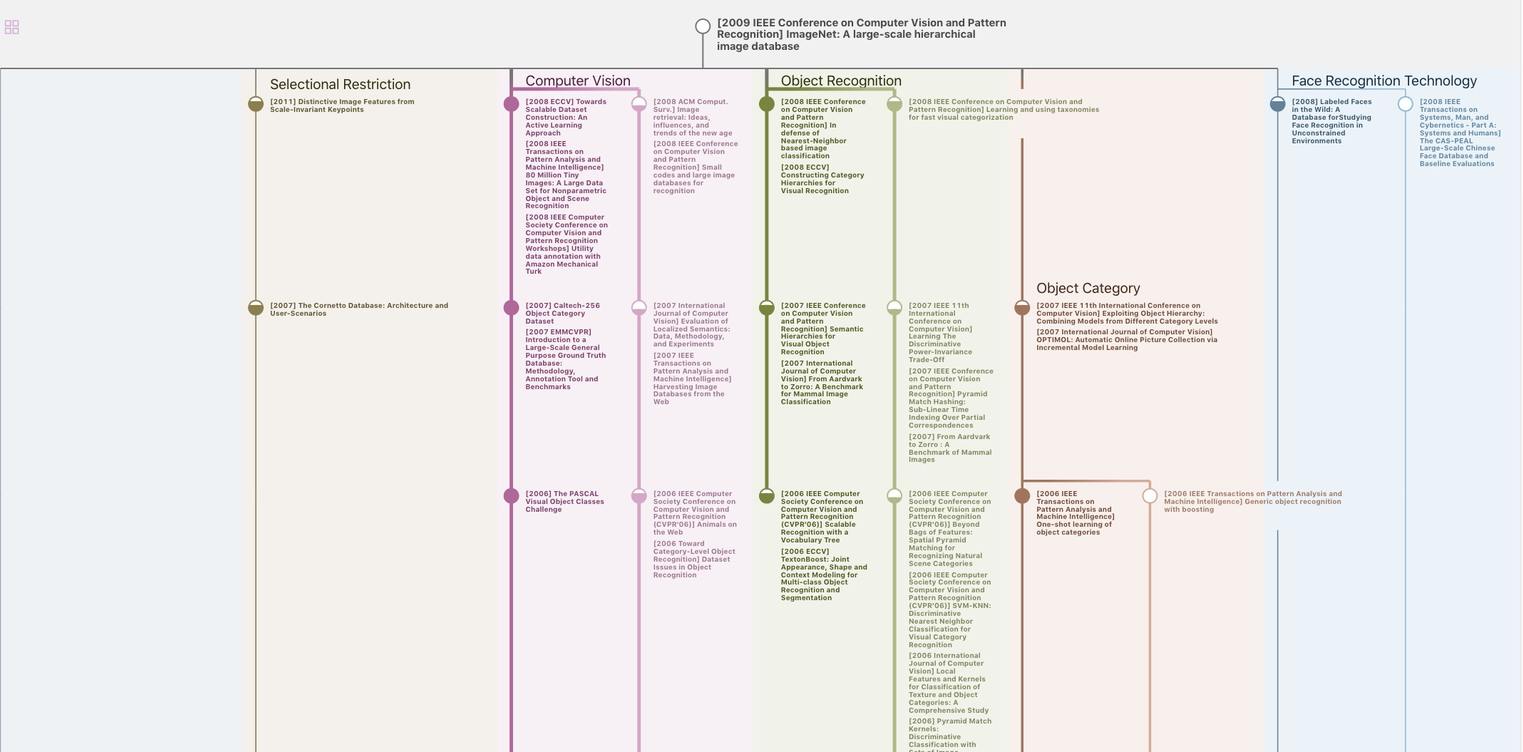
生成溯源树,研究论文发展脉络
Chat Paper
正在生成论文摘要