Improving Factual Consistency Between a Response and Persona Facts.
EACL(2021)
摘要
Neural models for response generation produce responses that are semantically plausible but not necessarily factually consistent with facts describing the speaker's persona. These models are trained with fully supervised learning where the objective function barely captures factual consistency. We propose to fine-tune these models by reinforcement learning and an efficient reward function that explicitly captures the consistency between a response and persona facts as well as semantic plausibility. Our automatic and human evaluations on the PersonaChat corpus confirm that our approach increases the rate of responses that are factually consistent with persona facts over its supervised counterpart while retaining the language quality of responses.
更多查看译文
关键词
Reinforcement learning,Supervised learning,Persona,Natural language processing,Computer science,Artificial intelligence,Response generation
AI 理解论文
溯源树
样例
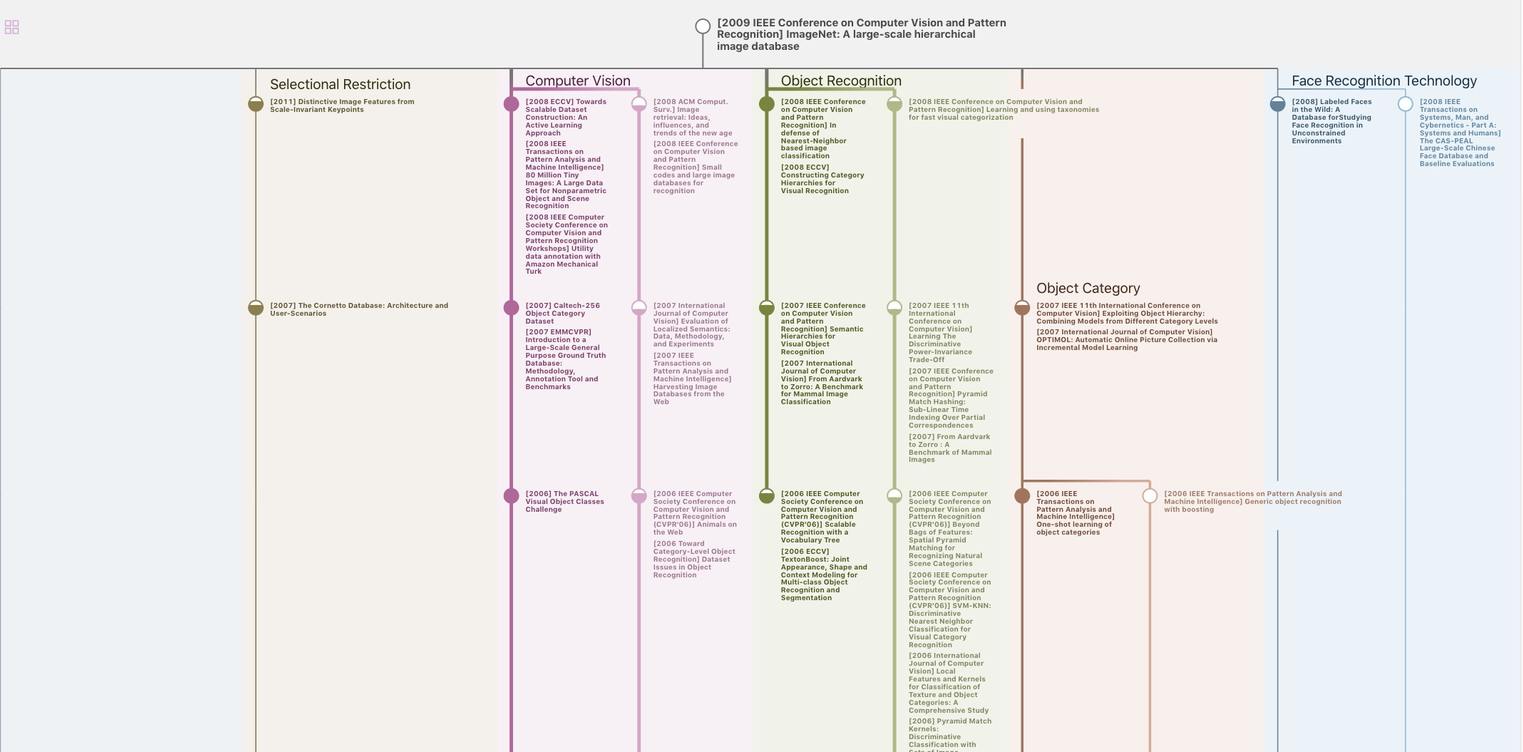
生成溯源树,研究论文发展脉络
Chat Paper
正在生成论文摘要