AN AUGMENTATION STRATEGY TO MIMIC MULTI-SCANNER VARIABILITY IN MRI
2021 IEEE 18TH INTERNATIONAL SYMPOSIUM ON BIOMEDICAL IMAGING (ISBI)(2021)
摘要
Most publicly available brain MRI datasets are very homogeneous in terms of scanner and protocols, and it is difficult for models that learn from such data to generalize to multi-center and multi-scanner data. We propose a novel data augmentation approach with the aim of approximating the variability in terms of intensities and contrasts present in real world clinical data. We use a Gaussian Mixture Model based approach to change tissue intensities individually, producing new contrasts while preserving anatomical information. We train a deep learning model on a single scanner dataset and evaluate it on a multi-center and multi-scanner dataset. The proposed approach improves the generalization capability of the model to other scanners not present in the training data.
更多查看译文
关键词
Multi-scanner, data augmentation, Gaussian Mixture Models
AI 理解论文
溯源树
样例
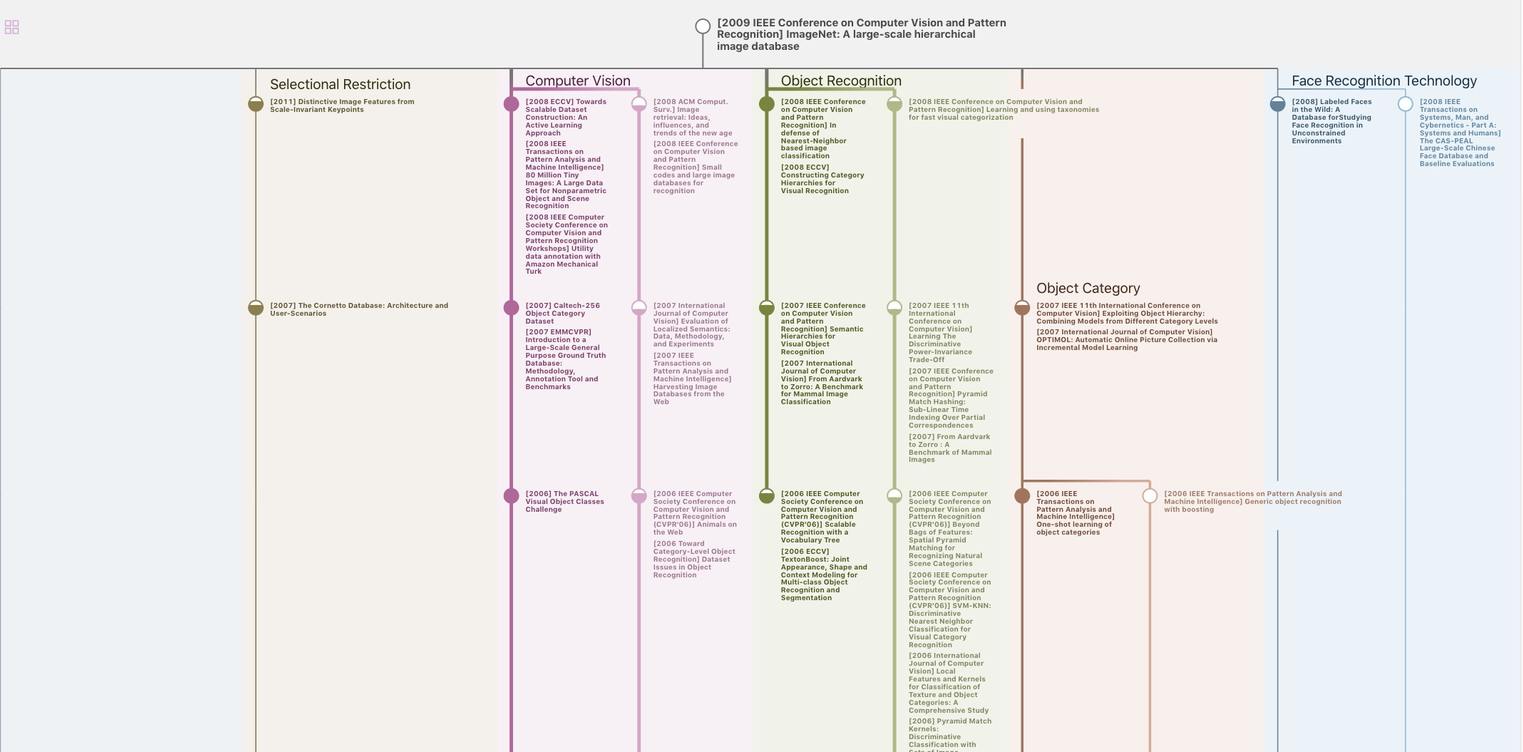
生成溯源树,研究论文发展脉络
Chat Paper
正在生成论文摘要