Deep Learning In Automatic Sleep Staging With A Single Channel Electroencephalography
FRONTIERS IN PHYSIOLOGY(2021)
摘要
This study centers on automatic sleep staging with a single channel electroencephalography (EEG), with some significant findings for sleep staging. In this study, we proposed a deep learning-based network by integrating attention mechanism and bidirectional long short-term memory neural network (AT-BiLSTM) to classify wakefulness, rapid eye movement (REM) sleep and non-REM (NREM) sleep stages N1, N2 and N3. The AT-BiLSTM network outperformed five other networks and achieved an accuracy of 83.78%, a Cohen's kappa coefficient of 0.766 and a macro F1-score of 82.14% on the PhysioNet Sleep-EDF Expanded dataset, and an accuracy of 81.72%, a Cohen's kappa coefficient of 0.751 and a macro F1-score of 80.74% on the DREAMS Subjects dataset. The proposed AT-BiLSTM network even achieved a higher accuracy than the existing methods based on traditional feature extraction. Moreover, better performance was obtained by the AT-BiLSTM network with the frontal EEG derivations than with EEG channels located at the central, occipital or parietal lobe. As EEG signal can be easily acquired using dry electrodes on the forehead, our findings might provide a promising solution for automatic sleep scoring without feature extraction and may prove very useful for the screening of sleep disorders.
更多查看译文
关键词
deep learning, single channel electroencephalography, automatic sleep staging, bidirectional long short-term memory, attention mechanism
AI 理解论文
溯源树
样例
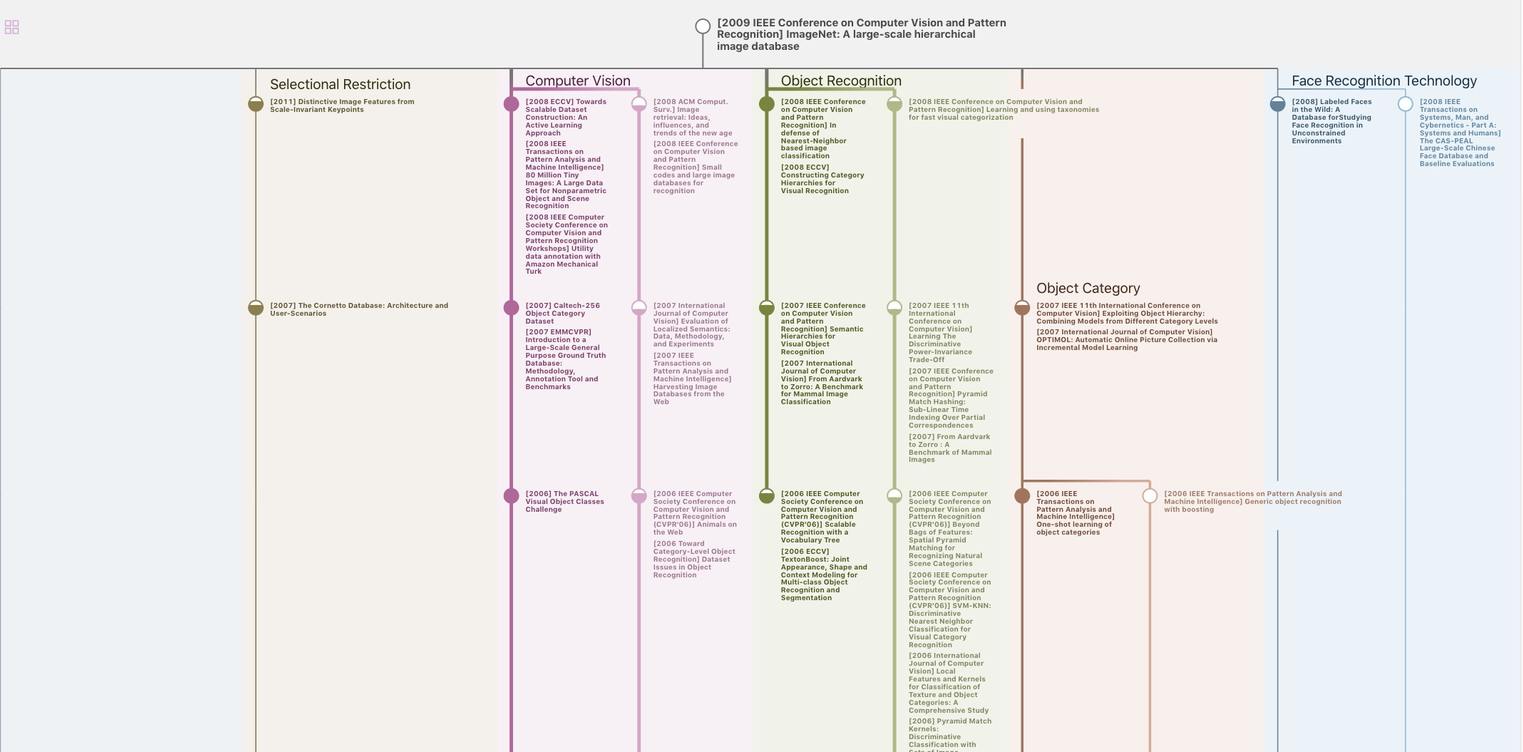
生成溯源树,研究论文发展脉络
Chat Paper
正在生成论文摘要